This book is distributed under the terms of the Creative Commons Attribution-NonCommercial-NoDerivs License which permits noncommercial use and distribution provided the original author(s) and source are credited. (See https://creativecommons.org/licenses/by-nc-nd/4.0/
NCBI Bookshelf. A service of the National Library of Medicine, National Institutes of Health.
Structured Abstract
Background:
Juvenile idiopathic arthritis (JIA) is the most common pediatric rheumatic condition, affecting 1 to 4 of 1000 children. More than half have polyarticular JIA (pJIA) and experience poorer outcomes and quality of life. Many effective treatments for pJIA are now available, but there is significant treatment variation among pediatric rheumatologists, due in part to a lack of data comparing the effectiveness of different timing for starting biologic medication. The Childhood Arthritis and Rheumatology Research Alliance (CARRA) has developed 3 standardized pJIA consensus treatment plans (CTPs) to be the subject of comparative effectiveness research (CER). By directly comparing clinical and patient-reported outcomes (PROs) in patients with pJIA treated with the 3 CTPs, the results of this study can provide critical information to assist physicians and patients in their treatment decisions.
Objectives:
Our study, Start Time Optimization of biologics in Polyarticular-JIA (STOP-JIA), compared the effectiveness of the pJIA CTPs in real-life settings. We proposed the following specific aims: to compare (1) the clinical effectiveness of 3 different strategies for starting biologic therapy in untreated pJIA using clinically inactive disease (CID) at 12 months as the primary outcome, and (2) PROs and caregiver-reported outcomes as the secondary outcome.
Methods:
STOP-JIA was a prospective observational cohort study conducted using the CARRA Registry that compared 3 CTPs:
- Step Up. Nonbiologic disease-modifying antirheumatic drug (DMARD) monotherapy (such as methotrexate or sulfasalazine), adding biologic medication if needed after ≥3 months
- Early Combination. DMARD and biologic medication are started together
- Biologic First. Biologic monotherapy, adding DMARD medication later if needed
Children and their caregivers in consultation with their pediatric rheumatologist chose 1 of the 3 CTPs and were followed for 12 months. There was no randomization.
The primary outcome was the proportion of children achieving CID at 12 months off glucocorticoids (GCs). Secondary outcomes were the comparison of Patient-Reported Outcomes Measurement Information System (PROMIS) Pain Interference and Mobility PROs that addressed variables identified as important in a caregiver survey and/or were recommended by the PCORnet PRO Common Measures Working Group (as part of the PCORI initiative to facilitate integration of electronic health record data into CER.1 The primary analysis was intention to treat.
Other secondary outcomes included the achievement of Juvenile Arthritis Disease Activity Score (JADAS) remission, time to achievement of JADAS remission and CID, adverse events, medication adverse effects, and time to discontinuation of GCs. Propensity score (PS) modeling was used to adjust for potential confounders, and multiple imputation (MI) was used to address missing data. Adjusted comparisons were also made using Bayesian models.
Results:
We enrolled 401 children and adolescents with untreated pJIA at 56 CARRA Registry sites. One patient who did not have JIA was started on treatment but was subsequently excluded from the analysis, leaving 400 analyzable patients. In total, 257 (64%) patients were started on the Step Up CTP, 100 (25%) patients were started on the Early Combination CTP, and 44 (11%) patients were started on the Biologic First CTP. Twenty (5%) patients were lost to follow-up before the 12-month visit, and of the remaining 380 patients, 43 (11%) did not have a 12-month visit (28 Step Up, 12 Early Combination, and 3 Biologic First). Treatment choice was made through shared decision-making. There were significant baseline differences between the CTP groups in JIA categories, number of active joints, physician global assessment of disease activity, and 10-joint clinical JADAS (cJADAS10) scores. The unadjusted rates for achieving CID at 12 months (primary outcome) were 37% (95% CI, 27%-49%) in Early Combination, 32% (95% CI, 26%-39%) in Step Up, and 24% (95% CI, 12%-43%) in Biologic First. After PS weighting and MI, the rates were 47.3% (95% CI, 32.6%-62%) in Early Combination vs 37.8% (95% CI, 29.4%-46.2%) in Step Up (P = .17). The cJADAS10 remission (score ≤2.5) at 12 months (secondary outcome) favored Early Combination over Step Up (66.6% vs 42.4%, respectively; P = .02). No significant differences were observed in the 2 PROMIS measures of pain interference and mobility between groups, although both outcomes improved during the study.
Conclusions:
The STOP-JIA study addressed a question of critical importance to children with pJIA, their caregivers, and their health care providers: When is the best time to begin biologic medications to achieve the optimal clinically reported outcomes and PROs? Our results indicate no significant differences between the 3 CTPs in achieving the primary outcome of CID at 12 months off GCs. The Early Combination CTP group did show improvement in a secondary outcome (cJADAS10 remission) compared with the Step Up group, but these results are limited by substantial missing data. This is the first use of CARRA standardized CTPs in an observational prospective study to determine comparative effectiveness.
Limitations:
This is a diverse population of patients, and even though all patients had polyarticular forms of JIA, multiple categories of JIA were included. Because this was an observational study without randomization, there were baseline differences in the CTP groups, which required adjustments to reduce bias, but there is likely residual confounding. Small sample size, variation in CTP and medication adherence, missed visits, incomplete questionnaires, and missing data were also limitations. Last, fewer patients were enrolled in the Early Combination and Biologic First CTPs than was expected, limiting the power of some of the planned analyses.
Background
Juvenile idiopathic arthritis (JIA) is the most common pediatric rheumatologic disease, with prevalence estimates ranging from 1 to 4 per 1000 children.2-6 The term JIA describes a clinically heterogeneous group of diseases characterized by childhood arthritis involving ≥1 joints. The majority of affected children have a polyarticular form of JIA (pJIA) involving ≥5 joints (see Childhood Arthritis and Rheumatology Research Alliance [CARRA] Registry Protocol in Appendix A for a breakdown of different types of JIA that were found in the CARRA Legacy Registry). Children and adolescents with pJIA often have refractory disease and long periods of active disease that increase their risk of joint damage, impair their quality of life (QOL), and cause poor functional outcomes.7,8 A major goal of treatment is disease inactivity, which can help prevent growth disturbances, joint contractures/destruction, and functional limitations.9 Children with JIA report poorer health-related QOL (HRQOL) than do their healthy peers. Up to 30% of children treated aggressively with biologic medications report poor HRQOL.10-12 The impact of JIA is not confined to childhood: Nearly half of patients in longitudinal observational cohorts report recurrent or ongoing disease activity into adulthood, including active arthritis, progressive joint destruction, and decreased QOL.11,13-16 In addition to decreased overall HRQOL, children with JIA report pain and fatigue that is not closely correlated to disease activity.17-21 Thus, it is of central importance that treatment approaches for JIA target not only clinical disease activity, but also aspects of HRQOL important to patients and their families.
The treatment dilemma this study addresses is which of the 3 most common treatment strategies used in JIA is most effective. They are (1) starting with methotrexate alone and then adding a biologic disease-modifying antirheumatic drug (DMARD) only if the response has been suboptimal after a period of time; (2) using both methotrexate and a biologic DMARD at the start of treatment; and (3) using only a biologic DMARD first. Although many patients respond well to methotrexate alone and never have to start a biologic (in the first strategy), it is possible that those who do not respond may not do as well as they would have if a biologic had been started earlier (because there may be a window of opportunity early in the disease). On the other hand, if biologics are started early for all patients, there may be patients who would have done well on methotrexate alone and are needlessly exposed to biologics (biologics have potentially adverse effects, some of which depend on the specific medication).
Adjunctive glucocorticoids (GCs) are commonly used to treat children with pJIA, with almost 40% of patients with JIA in the CARRA Registry ever having been exposed to GCs despite the fact that these drugs have known adverse effects and long-term toxicity. Rapid reduction and discontinuation of GC treatment (if started) is an important goal of treatment.22 In our study, the suggested tapering schedules for GCs were provided and were followed if possible, but in real-world situations, clinicians must taper by assessing each patient's ability to tolerate lowering the GC dose. Therefore, each patient's GC tapering schedule will vary depending on disease severity, disease activity, and response to treatment. Knowing which treatments are most effective at achieving the outcomes desired by patients and families (including discontinuing GCs as quickly as possible) while maintaining overall disease control is critically important.
The American College of Rheumatology (ACR) has published treatment recommendations for JIA, but they are based on consensus review of the very limited existing literature and have not been formally assessed.23,24 Because of the absence of evidence, treatment practices vary widely among pediatric rheumatologists.25,26 A variety of therapies are currently used to treat pJIA, including both biologic drugs and DMARDs. Etanercept, adalimumab, tocilizumab, and abatacept are approved by the FDA for the treatment of pJIA. However, because the clinical trials of these medications were designed to obtain regulatory approval, eligibility criteria usually included prior failure of DMARD therapy, meaning that these patients usually had long-standing JIA27-29 and only placebo comparators were used. Postmarketing approval studies have assessed only single agents in the context of drug-specific registries or single-center cohorts. As a result, the comparative effectiveness and safety of the most commonly used medications (and combinations thereof) for pJIA are unknown. We lack studies evaluating the relative effectiveness of medications for pJIA, the optimal time to add or change medications if disease inactivity is not achieved, and the optimal timing for initiation of biologic treatments for new-onset disease. Two clinical trials (Trial of Early Aggressive Therapy in Juvenile Idiopathic Arthritis [TREAT]30 and Aggressive Combination Drug Therapy in Very Early Polyarticular Juvenile Idiopathic Arthritis [ACUTE-JIA]) that assessed the effect of Early Combination treatment with a DMARD and a biologic compared with DMARD only had differing rates of achieving clinically inactive disease (CID).31 Both trials supported the concept that initiating effective therapy early in the disease course of pJIA can lead to improved disease activity outcomes. Lack of evidence perpetuates the wide variation in the treatment of children with pJIA, hindering optimal health outcomes. Our comparative effectiveness research (CER) study, Start Time Optimization of biologics in Polyarticular-JIA (STOP-JIA), addressed these knowledge gaps by assessing the effectiveness of the 3 different but commonly used strategies for introducing biologic DMARDs early in the course of pJIA.
Standardized consensus treatment plans (CTPs) were developed using consensus methodology for newly diagnosed children and adolescents with pJIA.32 The CTPs were developed through CARRA, a pediatric rheumatology research network that encompasses >95% of pediatric rheumatologists and clinical sites across the United States and Canada. The CTPs for pJIA vary by the timing of introduction of biologic medications into the treatment of new-onset pJIA: (1) Step Up, start nonbiologic DMARD followed by biologic if inadequate response; (2) Early Combination, start DMARD and biologic together at treatment initiation; and (3) Biologic First, start biologic monotherapy at initiation. All CARRA members had the opportunity to participate in the consensus meetings that laid the foundation for the CTPs, ensuring the broad acceptance of, familiarity with, and ability to use the CTPs in daily clinical practice. By standardizing medication doses and routes, interval assessments, treatment escalation strategies, and outcome assessments, care pathways such as CTPs may by themselves improve care. However, STOP-JIA was intended to provide desperately needed evidence that can be used to reduce treatment variation and improve outcomes by determining which initial treatment strategy is most effective at achieving overall disease control as well as other outcomes desired by patients and families.
STOP-JIA was a prospective observational study comparing the clinical effectiveness and impact on patient-reported outcomes (PROs) of 3 different CTPs in patients with new-onset pJIA to answer the question of when (or if) to start biologic medications. The CARRA Registry (described below) was used for data collection.
Specific Aims
Specific Aim 1
To compare the clinical effectiveness of 3 different strategies (CTPs) for using biologic medications in achieving CID off GCs at 12 months in untreated pJIA. Three common strategies that differ in the timing of biologic medication introduction were compared: (1) Step Up, using nonbiologic DMARD monotherapy and adding a biologic medication later if needed; (2) Early Combination, using a DMARD plus biologic medication at treatment onset; and (3) Biologic First, using biologic medication monotherapy at treatment onset.
Hypothesis 1
A significantly higher proportion of children started on a biologic medication at onset (Early Combination or Biologic First) will achieve CID after 12 months of therapy than those started on standard therapy (Step Up).
Specific Aim 2
To compare PROs and caregiver-reported outcomes of the 3 different CTPs.
Hypothesis 2
Between CTPs, there will be statistically significant differences in PROs and caregiver-reported outcomes that can inform future patients and providers in selecting optimal treatments.
Patient and Stakeholder Engagement
Patient engagement activities for STOP-JIA began several years before the study. Ann Murphy, the mother of a patient with JIA, participated with researchers and clinicians in the Delphi process to develop the CTPs for pJIA. This contribution made an enormous impact on the original design and development of the pJIA CTPs themselves, which formed the basis for this comparative effectiveness study. Vincent Del Gaizo, BS, the caregiver of a patient with JIA and a PARTNERS Patient-Powered Research Network (PPRN) investigator, joined the study team early in the process of drafting the application and protocol for STOP-JIA. Katherine Murphy, MPH, a young adult with JIA, was also recruited to the study team so that both the caregiver and patient perspectives could be included. The initial patient partners (PPs), Mr Del Gaizo and Ms Katherine Murphy, drafted an engagement plan to form a stakeholder advisory panel (SAP). A statement of work for the SAP was created to include the following:
- Share the patient and caregiver experience and advise on all stages of the study.
- Advise on all publicly facing study communications to ensure health literacy.
- Participate in quarterly teleconferences to discuss study progress and troubleshoot recruitment and other challenges.
- Participate in periodic email communications to develop content for a quarterly participant newsletter.
Next, the PPs engaged the Arthritis Foundation advocacy organization to assist in recruiting members to the SAP. The Arthritis Foundation created a survey to identify skill sets and perspectives needed for the panel and disseminated it via email to their listserv. Blinded responses were vetted and obtained through consensus. The study team identified 4 patient/caregivers with varied pJIA experiences and backgrounds to join the panel, in addition to a nurse, research coordinator, insurance payer, and Arthritis Foundation staff member. The stakeholders were then trained in patient-centered research, leveraging tools created by the PARTNERS PPRN. The panel held quarterly teleconferences and a face-to-face meeting at the CARRA Annual Meeting in spring 2016, 2017, 2018, and 2019.
While the SAP was being recruited and trained, the PPs participated in weekly study team calls to advise on many aspects of the project. The PPs selected the PROs based on their lived experience of knowing which outcomes are important to the community. The PPs changed the planned PRO data collection method from paper to electronic using an iPad in the clinic. The SAP piloted the electronic platform and thought it was too cumbersome to use because responses to questions had to be submitted 1 at a time. Based on this feedback, the study team had the surveys reprogrammed so all questions in a measure could be answered in 1 screen, which positively affected participants' willingness to complete the surveys.
An important contribution of the PPs to the study was the selection of an interactive Prezi (web-based informational tool) presentation and a quarterly newsletter as the main recruitment and retention tools. To create content for the presentation, the PPs and SAP identified barriers to enrollment based on their lived experience. The Prezi presentation included information about the disease, medications used, what it means to participate in the study, and why the study is important. A health literacy software program was used to ensure that the transcript reading level was as low as possible. The newsletter topics and content were written entirely by the SAP and revised by the study team. The newsletters provided participants with updates to the study and tips from experienced families to new families in navigating life after a pJIA diagnosis.
Patient engagement activities continued through the launch, activation, and follow-up phases of the study, with the PPs participating in all site activation calls for the multicenter project. On these calls, the PPs presented the lived experience of JIA and helped alleviate the concerns of site coordinators, nurses, and doctors about recruitment and enrollment of new patients into a research study. They discovered that providers often felt guilty about approaching newly diagnosed patients and families about participating in research. By speaking about their perspectives as patients and parents during the site activation calls and other meetings, the PPs addressed these concerns. Throughout the study, they continued to participate in all study team meetings, monitored the study and provided feedback, interacted with the study team to ensure that key stakeholder concerns and viewpoints were addressed throughout the study, and spearheaded the development of the quarterly newsletter materials given their unique perspectives of patients and family members.
STOP-JIA successfully enrolled one of the largest prospective cohorts of patients with untreated pJIA in the history of pediatric rheumatology. This effort required a dynamic effort from its diverse stakeholders. STOP-JIA engaged patients/caregivers as well as researchers to successfully complete this ambitious research study. The processes and tools developed for this project have been shared with the worldwide pediatric rheumatology research community. The PPs have become mentors to other parents and patients, thereby sharing engagement activities and changing the culture of research.
Although we were able to measure the stakeholders' sense of value through surveys and open and honest communication with all SAP members, we were not able to measure the impact that their contributions made on the successful execution of the study. More work is therefore needed to quantify the contributions of patients/caregivers on research studies going forward. The stakeholders' work is continuing with the development of a dissemination committee and plan for the results of this study (see Appendix B).
Methods
STOP-JIA was a prospective observational cohort study that addressed the clinical dilemma of when (or if) to start biologics in patients with untreated pJIA by comparing the clinical effectiveness and impact on PROs of 3 different CTPs. The CARRA Registry was used for data collection, and CARRA CTPs for pJIA were the strategies used to address our specific aims.
Study Design
This was a prospective observational cohort study assessing the comparative effectiveness of 3 CTPs currently used for pJIA. Although large, multicenter, randomized controlled trials (RCTs) are frequently considered the gold standard for measuring treatment efficacy, few such studies in pJIA have been performed because of the relatively low prevalence of the disease and the financial and logistical constraints associated with traditional RCTs. Furthermore, an RCT is not feasible in this setting given that many insurance companies will only cover biologic use once a nonbiologic DMARD has failed, therefore limiting the ability to randomly assign participants to Early Combination and Biologic First groups. Observational study design approaches, and CER methodologies specifically, are more efficient and feasible to execute in this patient population. This study used a particularly novel approach to conducting CER: implementing consensus-based standard-of-care strategies within the setting of an observational patient registry to reduce treatment variability and collect standardized data at regular intervals to allow for comparisons of effectiveness in a real-world clinic setting.33
CARRA developed a multicenter registry of pediatric rheumatic diseases, which was begun in 2009 with funding from a 2-year NIH grant (RC2AR058934).34 This original “legacy” registry enrolled almost 10 000 patients with a variety of pediatric rheumatic diseases, including JIA, by the time enrollment ended in 2012.22 The CARRA Registry was subsequently adapted, refined, and relaunched in 2015 to serve as a platform for CER and pharmacosurveillance that meets postmarketing regulatory requirements. CARRA established rigorous standards for data collection, including compliance with FDA requirements, data safety, adverse event reporting, security, and quality assurance, with systems in place for patient follow-up and data collection.35
With the goals of evaluating safety of medications, documenting treatment patterns, and assessing outcomes of patients with pediatric-onset rheumatic diseases, the new CARRA Registry began enrollment in April 2015, just before STOP-JIA began (November 2015). The data are housed at CARRA's clinical and data coordinating center, the Duke Clinical Research Institute (DCRI). According to the CARRA Registry Protocol (Appendix A), the CARRA Registry fulfills all PCORI standards for registries (https://www.pcori.org/research-results/about-our-research/research-methodology/pcori-methodology-standards#Data%20Registries). As of November 2019, >9000 patients had been enrolled in the CARRA Registry, including >8000 patients with JIA at 70 CARRA Registry sites spread across the United States and Canada.
The CARRA Registry selects sites that are pediatric rheumatology practices with at least 1 pediatric rheumatologist who agrees to serve as the site's principal investigator. All sites must also have resources to assist the principal investigator in conducting registry activities (patient enrollment, consenting, data entry, and regulatory maintenance). Typically, this will be a study coordinator along with ≥1 subinvestigators (other clinical providers at the site who treat patients with pediatric rheumatologic diseases). Staff working on the CARRA Registry also work on registry cohort studies (such as STOP-JIA), so study teams are able to capitalize on staff's knowledge and familiarity with the registry's procedures to operationalize additional study-specific activities quickly and efficiently. Patients are identified by their treating pediatric rheumatologist and are enrolled in the registry after providing informed consent. Patients continue to be seen clinically per standard-of-care recommendations, and sites collect data for the registry at regular intervals (at least every 6 months and whenever a new medication is started). Data collected include the following: demographics, disease characteristics and activity, laboratory results, medication history, safety events, and PRO measures of pain, physical activity, and overall well-being. If patients change pediatric rheumatologists, they will continue to participate in the CARRA Registry with the new provider. When patients transition to adult providers or to another nonregistry pediatric site, they will still continue to contribute data to the registry for at least 10 years from enrollment by participating in the long-term follow-up program, which is housed within the registry's coordinating center. This program allows patients to continue to provide self-reported data every 6 months pertaining to medication exposures, disease status, safety events, and overall QOL via a telephone interview. The long-term follow-up program is run by a team of experienced interviewers who are specifically trained to collect these data from participants.
Fifty-six of these registry sites were activated to enroll in STOP-JIA. All STOP-JIA participants are enrolled in the CARRA Registry. Therefore, all enrollment and administrative procedures related to the registry are the same for STOP-JIA. The standard CARRA Registry data (as stated above: medications, disease characteristics, laboratory results, PRO measures, and safety events) are collected for STOP-JIA participants, plus additional data specific to the aims of STOP-JIA (CTP selection and rationale and additional PRO measures). To minimize data collection burden at the sites, we used the same CARRA Registry data collection forms for all STOP-JIA participants, with the additional STOP-JIA–specific data added. Additional data collection time points (every 3 months, which is standard of care) were also required to appropriately assess the timing of CTP changes (see Appendix C).
Patients with new-onset, untreated pJIA who were ≤19 years of age at baseline and who presented to 1 of 56 CARRA sites participating in STOP-JIA (see Appendix D and Appendix E) were approached for enrollment by the treating physician during a clinic visit and were consented by either the physician or a study coordinator.
Entry Criteria
The following were the criteria for enrollment at baseline. Once patients were enrolled, they could begin treatment with 1 of the CTPs (which included GC treatment if the physician decided it was needed).
The patient should meet the following criteria:
- Be ≤19 years of age at baseline (if aged ≥18 years, agrees to be followed for at least 1 year)
- Have arthritis (per ACR definition):
- –
Present in 1 joint for at least 6 weeks
- –
≥5 active joints at baseline
- –
May have any of the following:
- Rheumatoid factor-positive (RF+) pJIA
- RF negative (RF−) pJIA
- Extended oligoarticular JIA
- Psoriatic JIA
- Enthesitis-related JIA
- Undifferentiated JIA
- Use contraception if sexually active (male and female)
Patients MAY have:
- Psoriasis
- Sacroiliitis
- Uveitis
- Enthesitis
- Past or current treatment with
- –
nonsteroidal anti-inflammatory drugs;
- –
intra-articular, topical, and intraocular steroids; or
- –
hydroxychloroquine.
Patients should NOT have:
- features consistent with systemic JIA;
- treatment with any medications for JIA aside from those listed previously before baseline (including GCs, which could be initiated after enrollment);
- known inflammatory bowel disease;
- known celiac disease;
- known trisomy 21 (Down syndrome);
- history of or current malignancy;
- concomitant serious active or recurrent chronic bacterial, fungal, or viral infection;
- significant organ system disorder limiting the use of treatments for pJIA; or
- live vaccine within a month before baseline.
Participant Selection, Recruitment, and Retention (Aims 1 and 2)
Eligible participants were enrolled in the CARRA Registry (see Appendix A) and started on a CTP treatment strategy at 1 of 56 participating CARRA centers across the United States and Canada. Patients were approached during a regularly scheduled visit, often at their first visit to the pediatric rheumatologist when they were initially diagnosed, because prior treatment for JIA (including GCs) was an exclusion criterion. Patients, caregivers, and providers together decided which CTP to initiate; this was an observational nonrandomized study. Each site had a study coordinator(s) who assisted in consenting participants and entering data. Although sites were asked to keep screening logs, it was not possible to document every potential participant, because the study coordinator was not aware of every new patient who was being diagnosed with pJIA (the diagnosis is almost always unknown before the actual visit). Still, if a patient was identified and approached and declined to participate, their reasons for nonparticipation were documented in the screening log.
Our enrollment strategy was to keep awareness of the study high at the sites among all study personnel, including all providers and nurses (not just study principal investigator and coordinators), in the following ways:
- We hosted a training webinar at the beginning of the study; additional webinars and calls were scheduled with each site to remind and educate all site physicians and study coordinators about the STOP-JIA study and to address site-specific recruitment issues.
- We distributed laminated cards containing details of the enrollment criteria and each of the 3 CTPs to all participating centers, providers, and study coordinators.
- We sent bimonthly newsletters to all CARRA Registry sites to provide updates on recruitment goals.
- We hosted monthly teleconferences with site study coordinators and investigators.
- We provided updates regarding the study at a face-to-face meeting at the CARRA Annual Meeting for stakeholder advisors, investigators, and coordinators, and at a CARRA Registry retreat held in 2018 that obtained feedback regarding barriers to enrollment at their sites and potential solutions.
- The PPs, SAP, and the PARTNERS PPRN assisted in devising strategies to address barriers from a patient/caregiver perspective, which included a participant newsletter, development of a Prezi presentation explaining the study, advertisement of the study on websites and social networking platforms, and development of a patient-produced video regarding why patients should participate in research.
Selection of Intervention and Comparators (Aims 1 and 2)
The proposed CTPs were developed using a CARRA-wide survey about current treatment practices, face-to-face consensus conferences at CARRA meetings, and a core workgroup of JIA experts who met through regular teleconferences. The final CTPs were endorsed by 96% of the CARRA JIA workgroup at the 2013 CARRA Annual Meeting and were published.32
Table 1 shows the details of the 3 CTPs (Step Up, Early Combination, and Biologic First). Each CTP details the classes of medications allowed at initiation (baseline) and then at follow-up visits depending on the condition of the patient (improved, no improvement, or worsened). The Juvenile Arthritis Disease Activity Score (JADAS), a composite measure of disease activity used to quantify disease state and change in disease status, was used as a guide to disease activity and escalation of therapy if needed.36 The components of the 10-joint clinical JADAS (cJADAS10; sum of physician global assessment of disease activity scored from 0 to 10, patient global assessment of overall well-being scored from 0 to 10, and the active joint count [to a maximum of 10]) as well as medication doses and start and stop dates were recorded at each visit, allowing for assessment of adherence and fidelity to the CTPs. The cJADAS10 is scaled from 0 to 30, with ≤2.5 representing “remission,” 2.6 to 5.1 representing low disease activity, 5.2 to 19 representing moderate disease activity, and 19.1 to 30 representing high disease activity.
Table 1
Descriptions of the pJIA CTPs: Medications Used and Escalation of Therapy at Follow-up Visits if Needed.
Study Outcomes
Aim 1
The primary outcome was the ACR provisional criteria for CID off GCs at 12 months after initiation of therapy (see below).9 CID was chosen because it was the only validated measure of disease status in JIA and is strongly related to disease remission (sustained CID), which is the first step toward cure, the goal of JIA treatment.9,30 Being off GCs is of critical importance as part of the outcome, because even though GCs by themselves can reduce disease activity, they should not be continued long term due to serious adverse effects and long-term toxicity, including growth failure, obesity, immunosuppression, bone and joint destruction (avascular necrosis), cataracts, and diabetes. In the analyses, children were classified as having active or inactive disease at each of their clinic visits using the JADAS and the ACR provisional criteria for CID in JIA at 12 months. CID requires that all the following criteria be met:
- No joints with active arthritis
- A physician global assessment of disease activity of “best possible” on the rating scale used
- No fever, rash, or generalized lymphadenopathy attributable to JIA
- No active uveitis
- Erythrocyte sedimentation rate (ESR) or C-reactive protein (CRP) level within normal limits, or, if elevated, elevation is not attributable to JIA
- Morning stiffness duration ≤15 minutes
Each variable was collected at every clinic visit by the physician, nurse, or medical assistant using the standard registry case report forms and entered into the database by the research coordinator and at the 12-month primary end point visit. Achieving remission prevents chronic disability and joint deformity/destruction, decreases chronic pain/fatigue, and improves work/school participation. Prior RCTs in more restricted cohorts of children with pJIA have illustrated that this outcome is likely obtainable in a large proportion of patients treated early in their disease course.30,31
The JADAS is a continuous measure of disease activity that has cutoffs for remission and for low, moderate, and severe disease activity, which makes it ideal for assessing patients at the point of care.36 The cJADAS10 is a simple sum of easily measured components: the physician global assessment of disease activity scored from 0 to 10, the patient/parent global assessment of overall well-being scored from 0 to 10, and the active joint count (to a maximum of 10). The cJADAS10 was not used as a primary outcome measure, but attainment of remission according to the JADAS and rates of JADAS remission at 12 months were important secondary outcomes that were assessed along with CID.
The pediatric ACR 70 (pACR70) score was also measured as a secondary outcome. This is a composite measure of improvement from baseline in 6 clinical variables; it has been used as an outcome measure in RCTs and is expressed as percentage of improvement. Patients are classified as meeting this end point if they have at least 70% improvement from baseline in 3 of any of the 6 variables in the core set: (1) active joint count; (2) joints with loss of range of motion; (3) physician global assessment; (4) patient/parent global assessment; (5) ESR and/or CRP levels, which are both common clinical laboratory inflammatory markers; and (6) score on the Childhood Health Assessment Questionnaire (CHAQ), with no more than 1 of the remaining variables worsening by >30%.37
Attainment of the primary outcome of CID required that the participant also be off GCs. Likewise, the JADAS remission and the pACR70 outcomes were only assessed in participants who were also off GCs.
Adverse events were reported if they were serious adverse events (SAEs) or events of special interest (ESIs) as defined by the CARRA Registry Protocol. SAEs are adverse events that result in death; are life-threatening; result in a hospitalization or prolongation of hospitalization, disability, or permanent damage; cause a congenital anomaly or birth defect; or require intervention to prevent permanent damage. ESIs are adverse events that are of special interest to the patient population in the registry or their treatments that occur even if they do not meet the definition of an SAE (see “Events of Special Interest” in Appendix A for a full list).
Aim 2
The PROs that we assessed are listed and described in Table 2.
Table 2
PROs for STOP-JIA.
PROs were chosen based on the results of a survey of 75 parents of children with established pJIA, in combination with the recommendations for pediatric items made by the PCORnet PRO Common Measures Working Group.1
The patient/parent survey was conducted by Y.K., P.F.W., and S.R. at Hackensack University Medical Center, Children's Hospital of Philadelphia, and Seattle Children's Hospital pediatric rheumatology clinics, respectively. Patients and parents were asked to select the 5 outcomes they felt were most important to capture from a list of 11 possible outcomes. The outcomes chosen most often were medication adverse effects (87.7%), pain (79.5%), medication safety (65.8%), ability to participate in usual activities (53.4%), growth (46.6%), anxiety (37%), depression (32.9%), and fatigue (30.1%). The PROMIS pediatric measures include specific modules for pain interference, fatigue, upper-extremity function, and mobility that have undergone preliminary validation in patients with JIA.40 Because the PROMIS measures offer reduced respondent burden with increased sensitivity and responsiveness to change, they are a particularly useful measurement tool for clinical research trials, especially pragmatic trials and comparative effectiveness studies. For these reasons, these measures were used in this study for each of the outcomes of interest if available. Parent-proxy reports were obtained for all participants able to complete the forms in English or in Spanish and by self-report for children ≥8 years of age (English or Spanish). PROs were completed on paper and entered into the registry by trained clinical research associates in cases where tablets were not available.
The 2 main secondary outcomes analyzed for this aim were the PROMIS Pain Interference and Mobility (patient-report) scores for the following reasons: Pain was a highly rated outcome in our patient/parent survey, as was the ability to participate in activities; and minimal important differences (MIDs) were available for these outcomes.40 PROs were assessed and analyzed for all participants, not only those who were off GCs. Because there are no validated instruments that specifically capture adverse effects and activity limitation, capturing and understanding these PROs was exploratory. To capture medication adverse effects, we used 1 question from the Juvenile Arthritis Multidimensional Assessment Report (JAMAR), a parent/patient questionnaire that includes items covering many domains, including physical function, pain, medication adverse effects, and satisfaction with disease status.38 Data pertaining to growth (height and weight) and medication safety, both selected by parents as outcomes of importance, were routinely collected by physician report at each visit.
Sample Size Calculations and Power
The following data sources were used to identify the percentages of patients undergoing treatment protocols approximating each of the 3 CTPs to provide an initial estimate of CTP use:
- 2011 CARRA-wide survey of initial treatment for pJIA. This survey was conducted to inform the early stages of CTP development. Providers were presented with various case vignettes with and without the following risk factors for poor prognosis (positive RF or anti–cyclic citrullinated peptide [CCP] antibodies, arthritis of the hip or cervical spine, and/or joint space narrowing or erosions on x-ray) and then asked to choose which treatment(s) should be started. The percentages of providers choosing each treatment in the presence and absence of the risk factors and other disease characteristics were as follows: DMARD monotherapy (equivalent to Step Up strategy), 41% with poor-prognosis risk factors and 81% without; DMARD plus biologic (Early Combination), 46% with risk factors and 9% without; and Biologic First, 31% with risk factors and 1% without.
- Retrospective analysis of medication use in participants with pJIA enrolled in the CARRA Registry (unpublished). A total of 368 of 2460 patients with pJIA had recent onset (within 6 months of diagnosis at baseline). There was significant treatment diversity among those started on systemic DMARD and/or biologic therapy: 63% were on a DMARD followed by a biologic (Step Up), 28% on therapy with both a DMARD and a biologic (Early Combination), and 9% on a biologic alone (Biologic First).
- A 2014 study of commercial insurance claims data of patients with JIA. Of patients with JIA newly started on a common class of biologic medication, tumor necrosis factor-α (TNFα) inhibitor medication, 37% had received the equivalent of Step Up therapy, 11% received Early Combination therapy, and 57% received Biologic First therapy.41
The study was originally planned to be analyzed using bayesian principles, so a bayesian approach was taken for calculating sample size and the probability of showing benefit (power). This resulted in assumptions for the use of each strategy as Step Up, 0.45; Early Combination, 0.35; and Biologic First, 0.20. Respondents to the survey at the 2014 CARRA Annual Meeting provided corresponding probabilities of achieving CID with the 3 CTPs of 0.2, 0.6, and 0.4, respectively. Using these assumptions for the true values of use and response probability, and a total sample size of 400, power was calculated for simple pairwise bayesian analyses addressing the primary aim of the estimating effect of the timing of introduction of biologics (comparing the proportion of participants with CID at 12 months in Early Combination vs the proportion with CID at 12 months in Step Up, or the proportion with CID at 12 months in Biologic First vs the proportion with CID at 12 months in Step Up), with a significant posterior probability being defined as ≥97.5%.
Power was calculated for a set of sample sizes in the neighborhood of 400, as these were considered plausible for the time frame of the study based on observed past rates of recruitment into the registry; the final sample size of 400 was chosen as a compromise between feasibility and high power. The power to detect any differences in these unadjusted analyses was >99.9%. The power for pairwise comparisons was >99.9% for Early Combination vs Step Up, 82% for Biologic First vs Step Up, and 70% for Early Combination vs Biologic First.
Imbalance in covariates that may occur between CTPs and the reduction of the effective sample size due to propensity score (PS) modeling is not known, so these power calculations represented a best-case scenario. With increasing amounts of confounding, represented by decreasing overlap between CTPs in the distributions of PSs, power will decrease, as the effective sample size for the comparisons depends on how many participants in each CTP are similar on PSs. However, in the planned bayesian analysis including information already collected from experts, classical power for these analyses could be higher if the results are in accordance with the priors; in this case, the prior distributions would act like additional data with the same response probabilities as the observed data. If results are in conflict with the priors, power could be higher or lower than calculated. The important case to consider is when the priors are more conservative about differences between CTPs than are the observed data. Here, the priors will shift the bayesian estimates for the differences in CTPs back toward the values in the priors; the priors act like data with smaller between-CTP differences, so the pooled data are less convincing. This scenario would suggest that more evidence is needed to change existing clinical opinion.
Using the sample size of 400, we calculated power for the PROMIS Pain Interference and Mobility outcomes. In the bayesian analysis, power was the chance of obtaining a posterior probability >97.5% that a CTP would be found to be better than its comparator. Recent work suggested that between-group standardized differences of 0.5 to 0.75 SDs are clinically important for these PROMIS measures.40 We investigated 3 scenarios: 1 scenario where the changes from baseline in mean PRO values were all equal; 1 scenario where they were 0, 0.25, and 0.5 SDs; and 1 scenario where the changes were 0, 0.375, and 0.75 SD. Because the PROMIS measures may not be concordant with clinical responses to treatment, we examined power across all possible combinations of changes across CTPs. Overall, the probability that bayesian linear models will show that at least 2 CTPs will differ in effectiveness is >94%. When there are no differences across CTPs, expected 95% CIs for pairwise comparisons range from ±0.22 SD to ±0.28 SD, and there is a high probability (89%-98%) that the 95% CIs will exclude a clinically important difference of 0.5 SD. All power calculations used simulations of 1000 studies for a given scenario, followed by bayesian analysis of those 1000 studies. The percentage of comparisons that met the criterion for “significance,” defined previously, was the estimated power for that comparison. As these simulations are all based on the use of uninformative prior distributions, the results will be very similar to what would be obtained if simulation was used to estimate power at a 1-sided significance level of α = .025.
Time Frame for the Study
All participants were started on 1 of the 3 CTPs and had a follow-up visit every 3 months for 12 months. This schedule is standard of care for follow-up visits for this disease, especially at onset and with the start of treatment. Because these patients were all also enrolled in the general CARRA Registry, they will continue to be followed every 6 months after their primary outcome visit for at least an additional 10 years, allowing the opportunity to assess longer-term outcomes in a future study. Some participants were assigned to the Long-Term Follow-up Call Center program during the study because they either transitioned from their pediatric provider to an adult provider or stopped being followed at a STOP-JIA site for various other reasons. These patients continued to be followed by phone, but they could not be assessed for the study outcomes because a physical assessment could not be performed.
Data Collection and Sources
Data collection for STOP-JIA followed the standard procedures for the CARRA Registry (see Appendix A). All participants were screened and enrolled by their CARRA Registry clinical site treating physician's team. All enrollment and follow-up clinical data were collected from the participants' medical record or directly from the provider(s) during a clinic visit. The research coordinator entered the data into the registry's electronic data capture (EDC) platform. The CARRA Registry compensates sites for completing routine registry visits (enrollment and every 6 months afterward). Site payments for the additional visits that were specific to STOP-JIA (3-month and 9-month visits) came from the PCORI funding. QOL and other patient/family-reported data were collected either via paper survey or electronically and entered into the registry's survey database. All follow-up data collection visits for STOP-JIA were tied to standard-of-care clinical visits, so families did not have to plan for extra visits. Site staff maintained visit trackers to ensure that all STOP-JIA data collection time points were captured. These trackers included the allowable visit windows during which a clinic visit may count for a STOP-JIA time point. Sites received regular reports from the coordinating center listing participants who were due for a follow-up visit, but one had not been entered into the EDC. Especially for the 12-month visit, site staff called participants to remind them they needed to return to the clinic for a follow-up visit. Staff would also use other standard communication methods approved at their clinical site to remind participants they were due for a visit with their rheumatology provider (such as sending letters or emails). Staff were trained to update a participant's status in the EDC once they became aware that the participant had moved or would not be returning to the site for continued care. By updating the status, the registry's long-term follow-up team was triggered to call the participant to determine whether he or she planned to return to a registry site, and, if not, the team documented the reason and initiated the long-term follow-up data collection procedures (these involved collecting self-report of medication use, medical events, disease status, and QOL measures every 6 months for the duration of registry participation). If the long-term follow-up team learned that a participant was planning to return to their clinical site, they would send a communication that would prompt the site to reach out to the participant and monitor their clinic schedule for an appointment.
Analytical and Statistical Approaches
Aim 1
The key exposures for this study were the 3 CTPs for treating patients with untreated pJIA. The primary end point was CID off GCs at 12 months. As the assignment to a CTP was nonrandom, the analysis had to address the possibility of confounding; the method of choice involved PS. Covariates that were potentially associated with both treatment choice and outcome were used to calculate the probability of choosing each of the 3 strategies.42,43
Primary outcome
The primary analysis compared the proportion of patients who had CID off GCs at 12 months in the 3 treatment approaches. There were 2 major stages to the analysis: (A) Stage 1: construction of a generalized boosted model from potential confounders to produce a PS for each participant to be on each of the CTPs; each participant would have 3 PS values.43 The goal of this stage was to find a PS model that gives satisfactory balance between CTP groups on the potential confounders. (B) Stage 2: PS-adjusted pairwise comparisons between CTPs. Given the moderate sample size, care had to be taken to exclude PS variables that were associated with CTP assignment but not with the primary outcome, CID off GCs at 12 months, as the inclusion of that class of variables could increase the variance of PS-adjusted estimated treatment effects without further reducing their bias.44 Standardized mean differences (SMDs) for baseline variables were calculated between CTPs and between those achieving and not achieving CID at 12 months. The following variables with an SMD of ≥0.1 in both sets of comparisons were identified as potential confounders: age, sex, race (classified as White and other), months since symptoms, 3 disease course groups (classified as RF+; RF−; and enthesitis related, extended oligoarticular, psoriatic, or undifferentiated), morning stiffness (classified as ≤60 minutes or >60 minutes), and abnormal ESR. In addition, 3 baseline Severity Scores were calculated and treated as potential confounders; these were the first 3 principal components from an analysis that included 5 baseline measures of disease activity or severity (MD global rating, parent global rating, JADAS score, active joint count, and limited joint count). These 3 components explained 93.5% of the variance in all 5 measures.
The twang package in R, which uses a generalized boosted logistic regression model, was used to fit 3 separate propensity models from these potential confounders; the 3 models predicted receipt or nonreceipt of each CTP.45 The optimal tree depth in each boosted regression was selected to minimize the SMDs between groups for the variables in the PSs. The PSs were used to generate weights (calculated as the reciprocal of the probability of receiving the treatment actually received), which were in turn used to reweight observations in each CTP. The aim of the reweighting is to create, for observations in each CTP, a pseudosample that has the characteristics of the combined sample. When applied to baseline characteristics, the weights allow an assessment of the balance between the characteristics of these pseudosamples. When applied to outcomes, the weights allow an estimation of average treatment effects, adjusted for baseline imbalance in the variables in the PS.
Several analytic options considered before recruitment began (eg, PS matching in a 1:1:1 ratio and 3 separate PS model analyses between each pair of CTPs) were judged to be less useful once it became apparent that recruitment to the Biologic First CTP was approximately 10% of the entire sample, not the expected 25%.
A more traditional modeling approach using adjusted logistic regression models was also used to estimate the probabilities of CID and odds ratios for CID between CTPs. These models included all variables in the PS added as predictors. The purpose of this was 2-fold: (1) as a check of the robustness of findings to a completely different analytic strategy; and (2) to facilitate a bayesian analysis that could assess the impact of including expert prior opinion. Once our primary analysis plan changed to use PS-weighted methods, the primary analyses could not incorporate the bayesian priors, as methods for PS-weighted bayesian models are still under development. The methods and results for the bayesian logistic regression analyses are presented in Appendix F.
Secondary outcomes
T-scores for the 2 main PROs (pain interference and mobility) were analyzed with linear mixed-effects models, with weighting by the reciprocal of the individual's PS score. An initial model had a random intercept for each participant and fixed effects for time of assessment, CTP, and the interaction between time and CTP, which represents a differential response to treatment. Where the test of the hypothesis of differential response to treatment had P > .05, a second model was fitted without the interaction. The time variable was parameterized so that estimates represent the mean difference in T-scores between adjacent assessment times (0-3 months, 3-6 months, etc).
Exploratory outcomes
Three binary exploratory outcomes were analyzed in the same way as the primary outcome, except for the reanalysis with the expert priors, as we did not elicit from experts their prior distributions for the effects on these outcomes. We compared CTPs on the (1) proportion of patients off GCs at 3, 6, 9, and 12 months; (2) the proportion with CID off GCs at 6 months; and (3) the proportion in remission (cJADAS10 ≤2.5) or with low disease activity (cJADAS10 ≤5.1) at 12 months.46 The cJADAS10 was calculated as a simple sum of (1) the active joint count (to a maximum of 10), (2) the physician global assessment of disease activity (0-10), and (3) the patient/parent global assessment of overall well-being (0-10). The pACR70 score was calculated per published criteria, with patients being classified as meeting this end point if they had at least 70% improvement from baseline in 3 of any of the 6 variables in the core set : (1) active joint count; (2) joints with loss of range of motion; (3) physician global assessment; (4) patient/parent global assessment; (5) ESR and/or CRP levels; and (6) CHAQ score, with no more than 1 of the remaining variables worsening by >30%.37 Scores were calculated for visits where at least 5 of the 6 core set variables were available. One planned exploratory outcome (change in CTP at any time) could not be analyzed, as CTP changes were not well documented by study personnel. A final exploratory outcome, time to first inactive disease visit, was analyzed with a Weibull proportional hazards model using interval censoring because we did not know the exact date of occurrence of CID, again weighting by PS.
Heterogeneity of treatment effects
Potential differential effects of CTPs across predefined subgroups were assessed by including group–CTP interaction terms in the regression model for the corresponding analysis and carrying out a Wald test of the statistical significance of the parameters representing the interaction. This was done separately for subgroups formed by sex (male and female), age at onset (0-4, 5-10, or 11-18 years), and JIA category (RF+, RF−, or other).
Interim analyses
Analyses of the primary outcome were conducted after one-third and two-thirds of the patients had completed follow-up assessments. No CTP was found to be decidedly inferior to the others at either interim analysis, so enrollment continued as planned for the remainder of the study. These analyses were conducted by the study's lead statistician (G.T.) and presented to the study team (investigators and patient partners listed as authors) to make decisions about the study.
Adherence to protocols and switching
The primary analysis was carried out with patients assigned to their initial CTP, a so-called intention-to-treat analysis. However, some patients switched CTPs, did not receive the intended treatment, or received treatments outside the CTP. Some of these treatment reassignments were due to administrative issues (eg, insurance approval), and some were due to clinical response (eg, a patient on Early Combination did not receive an initial biologic and had adequate response to DMARD monotherapy, thus being recategorized as Step Up); some patients changed their minds about the treatment, and in some cases there was a combination of issues. At the end of the study, the observed sequences of treatments in all 400 patients were assessed by an expert panel blinded to patient outcomes and were reclassified into relatively homogeneous groups.
Because patients could start biologic treatment at any time, not necessarily at baseline or at the follow-up visits, we decided to use 6 weeks as the cutoff for determining whether a patient was assigned to the Early Combination CTP (biologic and DMARD started before 6 weeks) or the Step Up CTP (biologic started after being on DMARD for at least 6 weeks). For the largest groups, the methods we used to analyze the initial CTPs were used to compare these de facto CTPs. The reclassified CTPs omitted 5 patients whose treatment patterns were adjudicated not to match any of the CTPs; a total of 39 of the remaining patients were reclassified to a different CTP from the one chosen at the outset of the study. These reclassifications were (1) from Early Combination, 18 patients reclassified to Step Up and 2 patients to Biologic First; (2) from Step Up, 15 patients reclassified to Early Combination and 1 patient to Biologic First; and (3) from Biologic First, 2 patients reclassified to Early Combination and 1 patient to Step Up. As the conclusions from the analyses of the reclassified CTPs are largely the same as the results for the declared CTPs, they are not reproduced in this report.
Methods for handling missing data
Although it was anticipated that the primary outcome would be available for essentially all patients, as children with pJIA require close follow-up, there were patients who were missing elements of the CID criteria or missed the 12-month visit entirely; thus, they could not be included in analyses of the primary end point. The data coordinating center (DCRI) made all attempts to retrieve any missing data through electronic monitoring and repeated site queries. However, where data were missing, they were likely not missing completely at random. Data quality (eg, missing values and inconsistencies) was assessed continually via feedback between the specific data analysis team and the data and clinical coordinating center for the registry, the DCRI.
Where the primary outcome, CID at 12 months, was missing, we took 2 different approaches to investigating the dependence of our results on the unobserved value: (1) Because this is a binary variable, we performed a sensitivity analysis comparing the results of best-case (all missing participants had CID) and worst-case (none had CID) scenarios for each of the 3 CTPs. We checked the robustness of results across these 2 scenarios as a measure of how important the issue of missing data could be to our conclusions. (2) Recognizing that the best-case and worst-case scenarios are likely unrealistic, we also generated a single set of results using multiple imputation (MI). As detailed in the “Study Outcomes” section (for aim 1), CID is a composite outcome. Any component indicating a failure to achieve CID means that the composite outcome is “not CID,” but if a participant has all observed components in the “good” category but ≥1 components missing, CID status is missing. However, the relationships between the CID components can be exploited to improve imputation of partially observed CID data. The missing 12-month CID outcome was imputed from individual elements of 12-month CID; assessments of CID at 3, 6, and 9 months; initial CTP choice; baseline values of JADAS score, MD global rating, and parent global rating; and the number of active joints. Twenty imputed data sets were created using the mice package in R. Estimates of the relative treatment effects between the CTPs (from propensity models or logistic regression) and their uncertainty were estimated in each data set and then averaged to produce point estimates and intervals that reflect both the variability in the observed data and the variability due to uncertainty in the missing values.47 Plans to assess nonignorable mechanisms for missing data were not pursued after we examined the results for CID from the complete-case, best-case scenario, worst-case scenario, and MI analyses.
Changes to the Original Study Protocol
The only modification that we made to the original study protocol involved expanding the allowable treatment amount a participant could have received before being enrolled in STOP-JIA. This change (2 weeks of GC, 1 month of methotrexate, and/or 1 prior dose of a biologic within 1 week of baseline) was initiated by the study investigators to ensure that sites were not missing patients who had newly started treatment with a CTP and were still early enough in their treatment course to contribute meaningfully to the STOP-JIA analyses. The updated protocol was approved by all applicable IRBs and by PCORI at the time the change was made.
Results
There was no randomization in this observational cohort study, as the provider and patient/family chose the CTP used. See Figure 1 for patient disposition throughout the study.
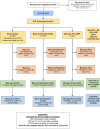
Figure 1
Participant Flow Diagram.
Overall, 444 participants were assessed for eligibility, and 401 were enrolled. One patient who was eventually found not to have JIA was excluded from the analysis. Of the 400 analyzable patients, 257 patients (64%) were started on the Step Up CTP, 100 (25%) on the Early Combination CTP, and 43 (11%) on the Biologic First CTP at baseline. Twenty patients (5%) were lost to follow-up before the 12-month visit: 2 of them withdrew consent, and 18 patients moved to a nonparticipating clinical site. That left 380 patients who continued with follow-up at the registry site for at least 12 months (249 Step Up, 93 Early Combination, and 38 Biologic First). However, 43 of these patients missed the 12-month primary end point visit, leaving a total of 337 patients (84%) who had data available for the primary end point analysis. Those who were included in the 12-month end point analysis included 221 patients for the Step Up CTP, 81 patients for the Early Combination CTP, and 35 patients for the Biologic First CTP.
Baseline Characteristics
The baseline characteristics of all participants are shown in Table 3. Although there were few demographic differences between the CTP groups, there were significant differences in some baseline disease characteristics, including length of time since diagnosis, number of active joints, limited joints, physician global assessment of disease activity, patient/parent global assessment score, JADAS score, duration of morning stiffness, disease course (JIA category), and CHAQ score (measure of functional assessment). In general, the participants on the Early Combination and Biologic First CTPs had higher disease activity and Severity Score measurements, as might be expected since initial treatment with a biologic is considered more aggressive treatment. PS weighting was used to adjust for these differences (Appendix G).
Table 3
Baseline Characteristics and Prevalence of Missing Data for All Participants.
Primary Results
Results for Specific Aim 1
Specific aim 1
To compare the clinical effectiveness of 3 different strategies to start biologic therapies in achieving CID without concomitant GC use at 12 months in patients with untreated pJIA.
If the patient had ever been on GCs, discontinuation of GCs (systemic or intra-articular) was required at least 1 month before the 12-month assessment to qualify as being off GC and meeting the primary end point.
Overall, 328 participants had complete data for the primary CID end point at 12 months. Tables 4a and 4b show the unadjusted estimates of CID at 12 months with the 95% CIs and the unadjusted between-group differences in CID, respectively. Tables 4c and 4d show the results after MI for missing data, showing that with this adjustment, each group achieved CID at a higher rate, but the between-group differences in achieving CID were similar to those before imputation. Overall, there were no significant differences between the groups in achieving CID in either the unadjusted or the imputed data.
Table 4a
Unadjusted Estimates of CID at 12 Months.
Table 4b
Between-Group Differences in CID, Estimates From Unadjusted Analyses.
Table 4c
Unadjusted CID Estimates After MI.
Table 4d
Unadjusted Between-Group Differences in CID After MI.
PS-Weighted and MI-Adjusted Results for Specific Aim 1
As shown in Table 3, several baseline variables differed across the 3 CTP groups. The PS-weighted analysis aims to reweight each sample so that it has the same distribution of potential confounders as the overall sample. The plots in Appendix G show the number of standard deviations difference (standardized differences) between each CTP group and the overall sample before and after weighting by the PS. In large studies of administrative data, differences <0.1 are considered unimportant, and although it may be more difficult to achieve this level of balance in smaller clinical data sets, this is also the standard for balance used here. The second figure in Appendix G shows that the Early Combination group was similar to the overall sample in, for example, White race, male sex, and months since start of symptoms, but that they had a higher prevalence of RF+ disease, morning stiffness >60 minutes, and the 2 calculated disease Severity Scores. One can think of the PS giving less weight to patients with severe disease and those with morning stiffness in the Early Combination group and more weight to patients with less severe disease. After propensity weighting, the sample standardized differences for the 3 variables (age and the 2 Severity Scores) were 0.12, 0.11, and 0.17, and all other values were <0.1, suggesting that the weighting had created a sample of Early Combination patients with characteristics close to those of the entire sample. As these weights create groups that are reasonably well balanced on the baseline characteristics, the same weights are applied to the outcomes so that the estimated differences between groups are the differences that would be seen in groups balanced on the observed baseline characteristics (ie, unconfounded differences).
Tables 5a and 5b show the same estimates and between-group differences after implementing both propensity weight adjustments and MI for missing data, which used the methods described in the “Analytical and Statistical Approaches” section (see “Methods for handling missing data”). The trend toward a higher percentage of participants in the Early Combination CTP achieving CID off GCs at 12 months was more pronounced after these adjustments, but the confidence intervals were wide, and the differences did not achieve statistical significance.
Table 5a
PS-Adjusted CID Estimates After MI.
Table 5b
PS-Adjusted Between-Group Differences in CID After MI.
Results for Specific Aim 2
Specific Aim 2
To compare PROs and parent-reported outcomes between the 3 different CTPs.
The primary outcomes chosen for this aim were the PROMIS Pain Interference and Mobility scores because pain and mobility were highly rated outcomes in a caregiver survey performed during the planning for the study and because MIDs are available for these outcomes.
A major limitation of this analysis was that the completion rates for all the PROs were low and worsened over the time frame of participation. For example, 75% of 400 participants completed the PROMIS Pain Interference measure at baseline, but only 49% completed it at the 12-month visit. When the numbers are broken down by CTP, they become exceedingly small, especially in the less used CTPs. Based on the low response rates, limited analyses of the PRO data were performed.
PROMIS Pain Interference Scores
Table 6a shows the summary statistics for Pain Interference for the entire cohort and for each CTP over time, illustrating that the scores began in the range of 1 SD above the population mean but that each group improved toward the population mean by the 12-month visit, except for the Biologic First CTP (however, the numbers in that group were exceedingly small throughout the study; see Figure 2). With these caveats, there was no statistically significant difference in the time trends between the groups (P = .21). The results from a linear mixed-effects model with fixed effects for time point and group are shown in Table 6b. The first 4 rows show the estimated changes between pairs of time points in Pain Interference scores. The next 2 rows show the estimated average differences from the Early Combination CTP at any time, and the last row shows the estimated change from baseline to 12 months. Higher T-scores for Pain Interference indicate more pain.
Table 6a
PROMIS Pain Interference Scores for Entire Cohort.
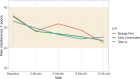
Figure 2
PROMIS Pain Interference T-Scores Over Time for Each CTP Group.
Table 6b
Estimates From Linear Mixed-Effects Model With PS Weighting for PROMIS Pain Interference T-Score Over Time.
PROMIS Mobility Scores
All the patients were less than 1 SD from the population mean with regard to PROMIS Mobility scores at baseline (Table 7a). Higher Mobility T-scores indicate better mobility. Responses to this measure and completion of the questionnaire were somewhat higher than those for Pain Interference, but at the 12-month visit, <50% of the participants had complete responses. Table 7b shows the estimated common effects of time and the average differences compared with the Early Combination CTP. Table 7a shows the summary statistics for Mobility for the entire cohort and for each CTP, illustrating that the scores began in the range of 1 SD above the population mean but that each group improved toward that value by the 12-month visit, except for the Biologic First CTP, which had a worsening at 9 months (however, the numbers in that group completing the survey were exceedingly small throughout the study). With these caveats, there was no evidence for a differential rate of change over time (P = .35), and all groups improved (see Table 7a and Figure 3).
Table 7a
PROMIS Mobility Scores for Entire Cohort.
Table 7b
Estimates From Linear Mixed-Effects Model With PS Weighting for PROMIS Mobility T-Score Over Time.
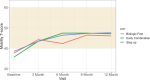
Figure 3
PROMIS Mobility T-Scores Over Time in Each Group.
Other PRO Measures
The results of multiple other PROs that we collected are shown in Appendix H.
Other Results
Adverse Events
The SAEs and ESIs (see Appendix A for all reportable ESIs) reported are listed in Tables 8a and 8b below. Adverse events were collected from November 2015 to September 2019. Forty-four participants experienced an SAE or ESI, of which 20 were SAEs and 25 were ESIs. No deaths were reported. The following conditions and SAEs were reported:
Table 8a
SAEs Reported in All Participants and by CTP.
Table 8b
ESIs Reported in All Participants and by CTP.
- Inflammatory bowel disease in 3 participants (1 SAE)
- Infections (including influenza [2 SAEs], IV antibiotics for infections [5 SAEs], shingles [1 SAE], and cellulitis [1 SAE])
- Fractures (2 SAEs)
- Hip pain and effusion (3 SAEs)
- Drug-induced lupus (1 SAE)
- Macrophage activation syndrome (1 SAE)
- Psychiatric events (2 SAEs)
- Vertigo (1 SAE)
- Leukopenia in 2 participants (no SAE)
- New-onset uveitis in 12 participants (no SAE)
- Hepatitis in 6 participants (no SAE)
- Hypersensitivity (immune system disorder) in 1 participant (no SAE)
- Psoriasis in 3 participants (no SAE)
The numbers were too small to compare any differences between the groups. We did compare these SAE and ESI proportions with other safety registries. A recent report describing event rates for 3 large registries (Pharmachild, Germany and Sweden) included >15 000 children and reported SAEs in 6.9% to 7.4% of them, which is comparable to the proportion of children with SAEs in this cohort (5.3%).48
Medication-Related Adverse Effects
Although there was a significant difference in the number of adverse effects reported in both the Step Up and Early Combination CTP groups compared with the Biologic First CTP group, there were no significant differences between the groups for specific adverse effects. Of note, 79 of the 392 participants never responded to the adverse effects question (yes or no). Of the 777 responses to the adverse effects question, 290 indicated the presence of ≥1 medication adverse effects over the course of the study (Table 9a).
Table 9a
Any Medication Adverse Effect.
The most commonly reported adverse effects were nausea (25.6%); mood disturbance (20.8%); headache (19.8%); sleep disturbance (13.1%), injection site reaction (12.8%); stomachache and vomiting (each 12.1%); and rash, mouth sores, and weight gain (10.5% each). All other adverse effects, including constitutional symptoms, weight loss, diarrhea, fever, urinary symptoms, increased body hair, stool problems, and gum problems, were reported in <10% of participants. Table 9b shows the unadjusted percentages of participants with medication adverse effects by group and shows that the Step Up and Early Combination CTPs had higher incidences of adverse effects than the Biologic First CTP group. Table 9c shows between-group differences of propensity weight–adjusted estimates of adverse effect incidence over 12 months. When propensity weight adjustments were applied, the differences between the Biologic First, Step Up, and Early Combination CTP groups were confirmed, but they were only significant for the comparison between the Biologic First and Step Up groups. We hypothesize that these differences reflect treatment with methotrexate, which is known to have a relatively high rate of associated adverse effects (used in both the Step Up and Early Combination CTPs but not initially in the Biologic First CTP).
Table 9b
Unadjusted Percentages of Participants With Adverse Effects and 95% CIs by CTP Group.
Table 9c
Between-Group Differences of Propensity Weight–Adjusted Estimates of Adverse Effect Incidence Over 12 Months.
Heterogeneity of Treatment Effect Analysis
Tables 10a-10c show the preplanned analyses examining potential heterogeneity of treatment effect (HTE) for the primary outcomes of CID. We did not find any variation in treatment effect across the subgroups that we analyzed.
Table 10a
Subgroup Analysis for CID by Sex.
Table 10b
Subgroup Analysis for CID by Age at Onset.
Table 10c
Subgroup Analysis for CID by JIA Category.
Results From Secondary Analyses
Clinical Juvenile Arthritis Disease Activity Score 10
A significant limitation of the JADAS analysis was that a large proportion of the data we needed to compute the score was missing, especially as time went on (there were 360 scores available at baseline and 264 scores at 12 months; see Table 11a). This was especially problematic for the comparative analyses because in the smaller 2 groups—the Early Combination and Biologic First CTPs—68 and 21 scores were available, respectively. We are planning an exploratory analysis combining the 2 early-biologic CTPs (Early Combination and Biologic First) to try to address this issue. Scores improved overall from almost all the participants being in a state of moderate-to-severe disease activity (mean [SD], 18.1 [4.7]) at baseline to low disease activity at 12 months (mean [SD], 4.7 [5.5]; see Figure 4).
Table 11a
Mean (SD), Number of Participants With Scores on cJADAS10, Overall and by CTP.
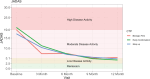
Figure 4
Mean cJADAS10 Scores in Each CTP Group Over Time.
Table 11b shows the observed JADAS Inactive Disease (ID) (cJADAS10 score ≤2.5) by CTP group. Given the extent of missing data on the cJADAS10 at 12 months, even though it was not in the prespecified analysis plan, we also combined the unweighted and the PS-weighted estimates of percentages with cJADAS10 ID, each across 30 imputed data sets. Missing cJADAS10 scores during follow-up were imputed from a model that included, for each participant, all other available cJADAS10 scores, CID at each month, CTP, and baseline values of the physician global rating, the parent global rating, and the active joint count. Because several variables (ie, Severity Scores 1 and 3, White race, morning stiffness > 60 minutes, and abnormal ESR) are somewhat imbalanced between CTP groups, we added those variables to the PS-weighted model as covariates.
Table 11b
Observed JADAS ID (cJADAS10 Score ≤2.5) by Group.
Table 11c shows the unweighted JADAS ID (cJADAS10 score ≤2.5) estimates by group after imputation of 12-month cJADAS10. Table 11d shows the unweighted between-group differences in percentages of JADAS ID using estimates from the imputed data. The results of the imputed data with PS-weighting and covariate adjustment are shown in Tables 11e and 11f. These results, even after multiple adjustments, show that cJADAS10 scores were significantly improved in the Early Combination CTP group compared with the Step Up CTP group, unlike the results for the primary outcome of CID at 12 months. The numbers in the Biologic First CTP group are too small to be able to make any definite conclusions. Figure 5 shows the unadjusted cJADAS10 data (all JADAS disease activity groups) of the percentages of participants in each CTP group over time.
Table 11c
JADAS ID (cJADAS10 Score ≤2.5) Estimates From Imputed Data by Group.
Table 11d
Between-Group Differences in Percentages in JADAS ID Using Estimates From Imputed Data.
Table 11e
Predicted Percentage of Participants With JADAS ID From PS-Weighted and Imputed Model With Additional Adjustment for Severity Scores 1 and 3, White Race, Morning Stiffness >60 Minutes, and Abnormal ESR.
Table 11f
Differences in JADAS ID Estimates From PS-Weighted Model and Imputed Model With Additional Adjustment for Severity Scores 1 and 3, White Race, Morning Stiffness >60 Minutes, and Abnormal ESR.
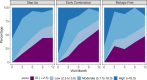
Figure 5
Disease Activity States by JADAS Scores Over Time in Each CTP Group (Observed Data).
Assessing Achievement of pACR70 Scores at 6 and 12 Months
We assessed achievement of pACR70 scores (which represent at least a 70% improvement in clinical variables), as outlined in the Methods section, among all the participants and in the 3 CTP groups at 6 months and 12 months.
Table 12a shows the distribution of participants who achieved the pACR70 at 6 and 12 months and the total number of participants with enough data at these time points to calculate the score. Of all the participants, 65% had enough data at 6 months, and 60% had enough at 12 months.
Table 12a
Achievement of pACR70 at 6 and 12 Months.
pACR70 Score at the 6-Month Visit by CTP Group
Tables 12b and 12c show the unadjusted pACR70 scores at 6 months. The between-group analyses do not show any significant differences among the CTP groups, although as shown for other outcomes, there is a trend toward higher percentages of participants achieving the ACR70 in the Early Combination group.
Table 12b
Unadjusted Estimates of pACR70 at 6-Month Visit.
Table 12c
Between-Group Differences in pACR70 Outcome, Estimates From Unadjusted Analyses.
Table 12d
pACR70 Estimates at 6 Months From PS-Weighted Model.
Table 12e
Between-Group Differences in pACR70 at 6 Months, Estimates From PS-Weighted Model.
pACR70 Score Data for the 12-Month Visit
At the 12-month visit, higher percentages of participants achieved the pACR70 than at the 6-month visit. The unadjusted numbers show that there was a significantly higher chance of patients achieving the pACR70 in the Early Combination group than in the Step Up group (Tables 12f and 12g).
Table 12f
pACR70 Estimates at 12 Months From Unadjusted Analyses.
Table 12g
Between-Group Differences in Outcome of pACR70 at 12 Months, Estimates From Unadjusted Analyses.
Tables 12h and 12i show the PS-adjusted analyses of the imputed 12-month data, and this again shows that there is a statistically significant difference in the Early Combination CTP group achieving the pACR70 compared with the Step Up CTP group.
Table 12h
pACR70 Estimates From PS-Weighted and Imputed Model at 12 Months.
Table 12i
Between-Group Differences in pACR70 at 12 Months, Estimates From PS-Weighted and Imputed Model.
The trend of the Early Combination CTP group achieving this outcome is in keeping with what was seen with the cJADAS10 analyses. Further work including MI for missing data in these groups needs to be done to confirm these results.
GC Use Over Time in the 3 Groups
The results in this section first show the numbers and percentages of participants on GC (where recorded) at each follow-up time. Because GC use, as well as how quickly GC is weaned, is left up to the treating physician and because the goal of arthritis treatment in pJIA is to be able to discontinue GC as quickly as possible, this is a measure of success for any treatment. We therefore assessed the percentage of participants in each CTP group remaining on GC at each follow-up visit, not just at the 12-month end point visit. Figure 6 shows the decreases in GC use over time, by CTP group. Tables 13a-13g show the differences adjusted by the PS used in the main analysis of CID. At 12 months, so few patients were on GC that no adjusted analysis was performed; however, the Early Combination CTP group consistently performed better with respect to the percentages of participants being off GC at all time points except for the 9-month visit, likely indicating that these participants consistently had lower disease activity (this is also supported by the JADAS data discussed previously).
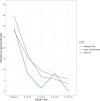
Figure 6
Decreases in GC Use Over Time, by CTP Group.
Table 13a shows the numbers and percentages of participants on GC at each time point by CTP.
Table 13a
Number and Percentage of Participants on GC at Each Time Postenrollment, Overall and by CTP.
GC use at 3 months
Tables 13b and 13c show PS-weighted estimates and between- group differences of GC use at 3 months.
Table 13b
GC Estimates From PS-Weighted Model at 3 Months.
Table 13c
Between-Group Differences in Percentages of Participants on GC (Estimates From PS-Weighted Model at 3 Months).
GC use at 6 months
Tables 13d and 13e show PS-weighted estimates and between-group differences of GC use at 6 months.
Table 13d
GC Estimates From PS-Weighted Model at 6 Months.
Table 13e
Between-Group Differences in GC Use, Estimates From PS-Weighted Model at 6 Months.
GC use at 9 months
Tables 13f and 13g show PS-weighted estimates and between-group differences in GC use at 9 months.
Table 13f
GC Estimates From PS-Weighted Model at 9 Months.
Table 13g
Between-Group Differences in GC Use, Estimates From PS-Weighted Model at 9 Months.
Attainment of CID Over Time
Attainment of CID at 6 Months
We assessed whether there were any differences in attaining CID at the 6-month time point between the CTP groups, but we did not find any significant differences either in the unadjusted numbers or after adjusting with PS weighting. Tables 14a and 14b show the unadjusted percentages and between-group differences, and Tables 14c and 14d show the PS-weighted adjusted analyses of CID at 6 months.
Table 14a
Unadjusted Percentages of Participants With CID at 6 Months and 95% CIs.
Table 14b
Unadjusted Between-Group Differences at 6 Months in Percentage of Participants With CID.
Table 14c
PS-Weighted Adjusted Estimates of Outcome CID at 6 Months.
Table 14d
Between-Group Differences in CID at 6 Months From PS-Weighted Model.
Time to First Attaining CID
Time to first attainment of CID off GCs was analyzed as another potentially important outcome, given that earlier disease control is hypothesized to lead to better disease outcomes. Comparisons of unweighted time to CID were made using the Weibull model with interval censoring. However, no PS-weighted interval-censored model was readily available, so weighted results are shown for a weighted Kaplan-Meier curve and a PS-weighted Cox regression with the visit time as the assigned event time (Figure 7). CID was assessed at each postbaseline visit, and the timing of CID was attributed to the interval since the last visit. The estimated hazard ratios of achieving CID (higher numbers are better) were similar between the 3 CTPs, although confidence intervals for the hazard ratios comparing each CTP with the Step Up CTP were wide (Table 15). PS-weighted time-to-event analyses (Figure 8) also suggest that there may have been more children on the Biologic First CTP at the 3-month time point with CID (faster achievement of CID) relative to the other groups, but overall, there were no significant differences between the groups.
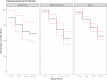
Figure 7
Unadjusted K-M Plot of Percentages of Participants Not Attaining CID.
Table 15
Results From PS-Weighted Cox Model for Time to First Visit With CID.
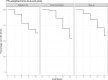
Figure 8
Adjusted K-M Plot of Percentages of Participants Not Attaining CID.
Comparison of JIA Disease Activity Measures
Because we have discussed multiple outcomes measures that assess JIA disease activity (or inactivity) in this report, we thought it would be useful to summarize the results in a table that compares the various measures and the results that were obtained through the STOP-JIA study (Table 16). Hopefully, this information will be helpful in planning and choosing end points and outcomes measures for future studies assessing various treatments for JIA.
Table 16
Comparison of Participants Attaining CID Off GCs, pACR70 Scores, and cJADAS10 Remission Outcomes at 12 Months.
Discussion
STOP-JIA is the first prospective observational study to measure the comparative effectiveness of different strategies for starting biologic therapy in pJIA. STOP-JIA's innovative approach using CARRA's CTPs, which represent the most commonly used current treatment approaches, facilitated the successful enrollment of 400 children with untreated pJIA and made this one of the largest prospectively followed inception cohorts of children with pJIA. Because the patients are also enrolled in the CARRA Registry, they will continue to be followed for at least 10 years, allowing unprecedented, detailed long-term follow-up of this cohort.
The optimal timing for starting biologics in children with untreated JIA has been the focus of active research. Two prior RCTs of initial biologic therapy in pJIA reached different conclusions about early-biologic use, reflecting different designs and study populations. The ACUTE-JIA (2011) study randomly assigned children with very early pJIA to infliximab/methotrexate, methotrexate, or triple therapy (methotrexate, sulfasalazine, and hydroxychloroquine).31 This study found that children receiving initial biologic therapy achieved ACR Pediatric-75 level of response at 54 weeks significantly more frequently than did the other arms. However, this study was limited to Finland, enrolled small numbers of children (19-20 per arm), and initiated treatment very early in the disease course (median, 1.5 months), thus reducing the generalizability of the results. TREAT-JIA (2012), a double-blind RCT comparing rates of CID at 6 months in participants receiving initial therapy with etanercept/prednisone/methotrexate vs placebo/prednisone/methotrexate, found no significant difference between the 2 groups.30 Recent ACR recommendations for the treatment of pJIA, derived from a systematic review of published data and expert consensus, supported initial treatment with DMARDs with rapid escalation to biologics if disease was not well controlled.24 The recommendations suggest that children with risk factors for more severe disease (eg, RF positivity, presence of joint damage, involvement of high-risk joints) may benefit from initial biologic treatment.
STOP-JIA adds important information to this body of literature by providing real-world outcomes among a large group of children managed in routine clinical care. Overall, there were no significant differences between CTPs in the achievement of CID off GCs at 12 months, even after adjusting for imbalances in baseline characteristics between CTPs.
Although there were no significant differences in the primary outcome, differences noted for important secondary outcomes may inform treatment decisions and future research. Analyses using the cJADAS10, a less stringent categorization of inactive disease (JADAS “remission”), suggested a potential benefit of using Early Combination treatment compared with using Step Up treatment (P = .0186), a result that merits additional evaluation in focused future studies and exploratory analyses. Analysis of the pACR70 results suggested similar results, with the Early Combination CTP group having significantly higher rates of pACR70 achievement after PS weighting than that of the Step Up CTP group (P = .0082), although again, there was a high rate of missing data for this outcome. The Early Combination group also had significantly lower rates of GC use than did the Step Up group (P = .016 and .026, respectively) at 3 and 6 months, which may reflect improved disease control. The ability to more rapidly reduce and stop use of GCs is an important outcome to physicians and stakeholders, given known toxicities associated with long-term GC use. Interestingly, CID was achieved much less commonly than predicted by expert physicians who participated in the bayesian exercise conducted during the planning phase of the study. Physicians overestimated the frequency of disease control, perhaps reflecting the fact that CID is a more stringent and less realistic target than is sought by patients and physicians in routine practice. Analyses of the secondary outcomes should be interpreted with caution given the limitations noted here.
Although limited by low response rates, analyses of PROs showed similar improvement across CTPs. Detailed PRO data were not reported for ACUTE-JIA and TREAT-JIA; therefore, PRO data from STOP-JIA aid in our understanding of how PROs respond to initial therapy and suggest that these responses do not differ between treatment approaches. However, patient- and parent-reported medication adverse effects were less commonly reported by participants in the Biologic First group than in the other groups, a difference that was statistically significant for the comparison between the Biologic First and Step Up CTPs even after PS weight adjustment.
SAEs and ESIs were reported in STOP-JIA participants, but the numbers of events were too low to detect any differences between the CTPs, and the proportion of children experiencing an SAE was similar to proportions of children with SAEs in other large, observational safety registries of patients with JIA.48 Last, STOP-JIA was the first large-scale study to use the CTPs and the CARRA Registry to perform a comparative effectiveness study, an approach specifically developed by CARRA for CER in rare diseases.49 The results suggest that the CTP development process was successful in distilling highly variable treatment practices into general treatment strategies.
In this study, health care providers achieved similar outcomes at 12 months with all 3 CTPs, suggesting that currently used strategies result in equivalent outcomes at 12 months. However, the results also indicate a need to improve current treatment approaches, as the majority of children did not achieve CID at 12 months. Nonetheless, as measured in this study, CID reflects disease inactivity status at only 1 point in time, and remission at 12 months may not have been the correct outcome to target at this time point. The clinical significance of CID is unknown: For example, it is not known whether CID at 12 months predicts future longer-term outcomes. Our secondary analyses show that far more children reached the cJADAS10 targets for remission and low disease activity, and exploratory analyses suggested that there may be differences between CTPs for achieving these outcomes. Further data collection and analysis are needed to assess the reasons for the disparity in these outcome measures and which outcome measure may be more useful in predicting future long-term outcomes for patients with JIA. Future research needs to address how to improve rates of CID and/or disease inactivity/low disease activity states in children with JIA, including continuing to work toward identifying subgroups that may particularly benefit from early biologics and assessing whether stricter treat-to-target approaches lead to sustained disease control and better long-term outcomes.
Subpopulation Considerations
STOP-JIA included a diverse population of children with multiple categories of pJIA and at least ≥5 active joints at baseline. The categories included (1) RF− pJIA, (2) RF+ pJIA, (3) extended oligoarticular JIA, (4) psoriatic JIA, (5) enthesitis-related JIA, and (6) undifferentiated JIA. It is possible that some categories of JIA may respond more favorably to a specific CTP than others, but, given the lower-than-expected enrollment in some of the CTPs, this study was not designed to have adequate power to detect such differences. The Early Combination and Biologic First CTPs also enrolled fewer patients than expected. Last, as stated in the Methods section, this study had sufficient power to detect important differences in outcomes between the CTPs but not to detect differential effects of the CTPs across subpopulations. We found no evidence of HTE of CTPs on CID across subgroups formed by sex, age, or category of JIA. However, the limitations imposed by sample size mean that we cannot rule out the possibility that the response to the optimal CTP may vary across these specific subpopulations or others that we did not test.
Study Limitations
The CARRA Registry and observational study design facilitated the enrollment of 400 children into this study, one of the largest prospectively followed JIA cohorts and a number not achievable for a rare disease in the context of a conventional RCT. An RCT was not feasible for many reasons: JIA is a rare disease with small numbers of untreated patients; many insurance companies will not cover biologic use until a DMARD has failed to gain disease control (making randomization to Early Combination and Biologic First groups not possible); and families are reluctant to enroll in an RCT, because enrollment most often occurs at the diagnosis visit when families are overwhelmed. However, this approach also highlighted the limitations of the current data collection practices in the registry, particularly regarding problems associated with missing data.
These results also identified important opportunities to optimize data collection in the CARRA Registry, which will in turn improve the registry's ability to continue to support CER, including using CTPs developed for other diseases. Only 328 out of 400 children had complete data for an assessment of the primary end point. Although MI did not alter the substantive conclusions, the overall incidence of CID was higher in the imputed data, which could be interpreted as a sign that the patients without data on CID were those who would be expected to have a better outcome. However, MI as used here assumes that missing data are missing at random, an assumption that cannot be tested with the observed data. If this assumption is incorrect or the model for imputation is incorrect, this process will not fully compensate for the missing data.
Furthermore, missing PRO data were also a significant limitation of the study, limiting our ability to detect differences in PROs between treatment strategies. Additional analyses suggest that the baseline characteristics of children with missing PROs did not differ significantly from those with complete data, but the planned analyses could not be completed. Although in principle, MI can be used with a ≥50% prevalence of missing values, the results become increasingly dependent on the assumptions of the imputation procedure, missingness at random in particular, so we did not try to impute the missing PROs.
There were significant differences in the CTP populations, including disease activity measures between the groups (see baseline characteristics in Table 3). We used statistical methods to maximize the similarity of the study populations across the CTPs, including propensity weight adjustments. Additionally, the distribution of patients in the study groups was quite imbalanced, with lower-than-expected enrollment in the early-biologic groups (Early Combination and Biologic First). Lower enrollment in the early-biologic groups, in combination with missing data, resulted in few analyzable patients for some outcomes. MI can reduce the bias that can result from differential omission of patients with missing outcomes. If there are good proxies for the prediction of missing outcomes, the increase in precision from the inclusion of all participants in the analyses can outweigh the increase in variance due to the uncertainty in the prediction of the missing values. However, the fact remains that we do not have the actual primary outcomes for almost 20% of participants. To identify potential bias in the sample, we are performing analyses to determine whether participants with missing PRO data were systematically different from those who completed the measures. This report does not address CTP adherence, but future planned exploratory analyses include assessing treatment effectiveness by actual CTP (rather than by intention to treat) and assessing treatment effectiveness based on the actual timing of medication introduction. Furthermore, undocumented changes in CTP might add variance to the outcome estimates.
Future Directions for STOP-JIA Data Analyses and Other Studies
- Improved understanding of genetic heterogeneity underlying current JIA categories may help identify patients who are most likely to benefit from early-biologic therapies and provide additional context for interpretation of the STOP-JIA results. Biosamples have been collected from a subgroup of STOP-JIA patients, which will be analyzed with this aim.
- Because children enrolled in STOP-JIA were also enrolled in the CARRA Registry, study participants will have ongoing follow-up that will allow for analyses of outcomes at later time points (eg, 2 years, 5 years). This is critically important, as CID is not durable, and JIA is a chronic disease.
- Assessment of a potential window of opportunity by determining whether STOP-JIA patients treated closer to symptom onset had improved outcomes at 12 months (CID, cJADAS10 scores) regardless of the initial therapy.
- Analysis of early- vs later-biologic groups: combining the Early Combination and Biologic First CTPs and comparing with the Step Up CTP, and assessment of patients who never started biologic therapy.
- Analysis using adjudicated treatment groups instead of intention-to-treat analysis performed for the primary analysis (for example, some participants were declared Early Combination but in fact ended up following the Step Up CTP).
- Reanalysis using the results of TREAT-JIA and ACUTE-JIA to inform the bayesian priors.
- Analysis of latent trajectories within each CTP cohort to identify patterns of response, which can inform treatment decision-making (and CTP choices) for future patients with pJIA, their families, and their physicians.
- Analyses and assessment of adherence using actual time elapsed from treatment start date and medication start and stop dates.
- Assessment of outcomes in patients with biologic use within 3 months and 6 months (regardless of CTP group) compared with patients who do not receive biologics in that time frame.
- The development of methods to widely disseminate and implement what we have learned from this study will enable more widespread use of the CTPs. Capturing the data resulting from widespread use will allow for an improved understanding of the impact of biologic timing for individual patients.
Conclusions
The CARRA STOP-JIA comparative effectiveness study addressed the optimal timing of initial biologic therapy in patients with pJIA and found no clear differences between Biologic First, Early Combination, and Step Up approaches in the ability to attain the primary end point of CID off GCs at 12 months. These study results confirm that any effective treatment, whether or not it includes biologic treatment, may lead to equivalent outcomes at 12 months (at least for CID), a result that was consistent across the primary outcome and all secondary outcomes. However, formal statements of equivalence are not possible, given the wide confidence intervals for differences between CTPs in the proportions of participants achieving CID, especially for comparisons involving the Biologic First CTP. The results of other outcomes indicating disease activity and treatment response (cJADAS10 and pACR70) did show that the Early Combination approach was superior to the Step Up CTP, but interpretation is limited because of missing data. It remains to be seen whether understanding individual patient characteristics and trajectories can lead to more individualized approaches, which will lead to improved outcomes. Last, the STOP-JIA data set represents a unique and rich resource of highly curated data on a large cohort of patients with untreated pJIA that will help answer many other questions through additional data analyses that are ongoing and planned and through longer-term follow-up with the CARRA Registry. The registry data set will also be available for analysis by other interested investigators, which will yield more insights into pJIA and its optimal management.
References
- 1.
- Fleurence RL, Curtis LH, Califf RM, Platt R, Selby JV, Brown JS. Launching PCORnet, a national patient-centered clinical research network. J Am Med Inform Assoc. 2014;21(4):578-582. [PMC free article: PMC4078292] [PubMed: 24821743]
- 2.
- Sacks JJ, Helmick CG, Luo YH, Ilowite NT, Bowyer S. Prevalence of and annual ambulatory health care visits for pediatric arthritis and other rheumatologic conditions in the United States in 2001-2004. Arthritis Rheum. 2007;57(8):1439-1445. [PubMed: 18050185]
- 3.
- Denardo BA, Tucker LB, Miller LC, Szer IS, Schaller JG. Demography of a regional pediatric rheumatology patient population. Affiliated Children's Arthritis Centers of New England. J Rheumatol. 1994;21(8):1553-1561. [PubMed: 7983664]
- 4.
- Malleson PN, Fung MY, Rosenberg AM. The incidence of pediatric rheumatic diseases: results from the Canadian Pediatric Rheumatology Association Disease Registry. J Rheumatol. 1996;23(11):1981-1987. [PubMed: 8923379]
- 5.
- Oen KG, Cheang M. Epidemiology of chronic arthritis in childhood. Semin Arthritis Rheum. 1996;26(3):575-591. [PubMed: 8989803]
- 6.
- Krause ML, Crowson CS, Michet CJ, Mason T, Muskardin TW, Matteson EL. Juvenile Idiopathic Arthritis in Olmsted County, Minnesota, 1960-2013. Arthritis Rheumatol. 2016;68(1):247-254. [PMC free article: PMC5024534] [PubMed: 26316119]
- 7.
- Wallace CA, Huang B, Bandeira M, Ravelli A, Giannini EH. Patterns of clinical remission in select categories of juvenile idiopathic arthritis. Arthritis Rheum. 2005;52(11):3554-3562. [PubMed: 16255044]
- 8.
- Ringold S, Seidel KD, Koepsell TD, Wallace CA. Inactive disease in polyarticular juvenile idiopathic arthritis: current patterns and associations. Rheumatology (Oxford). 2009;48(8):972-977. [PubMed: 19535609]
- 9.
- Wallace CA, Giannini EH, Huang B, et al. American College of Rheumatology provisional criteria for defining clinical inactive disease in select categories of juvenile idiopathic arthritis. Arthritis Care Res (Hoboken). 2011;63(7):929-936. [PubMed: 21717596]
- 10.
- Gutierrez-Suarez R, Pistorio A, Cespedes Cruz A, et al. Health-related quality of life of patients with juvenile idiopathic arthritis coming from 3 different geographic areas. The PRINTO multinational quality of life cohort study. Rheumatology (Oxford). 2007;46(2):314-320. [PubMed: 16877459]
- 11.
- Seid M, Opipari L, Huang B, Brunner HI, Lovell DJ. Disease control and health-related quality of life in juvenile idiopathic arthritis. Arthritis Rheum. 2009;61(3):393-399. [PMC free article: PMC5289409] [PubMed: 19248113]
- 12.
- Haverman L, Grootenhuis MA, van den Berg JM, et al. Predictors of health-related quality of life in children and adolescents with juvenile idiopathic arthritis: results from a web-based survey. Arthritis Care Res (Hoboken). 2012;64(5):694-703. [PubMed: 22238240]
- 13.
- Minden K, Niewerth M, Listing J, et al. Long-term outcome in patients with juvenile idiopathic arthritis. Arthritis Rheum. 2002;46(9):2392-2401. [PubMed: 12355487]
- 14.
- Oen K, Malleson PN, Cabral DA, Rosenberg AM, Petty RE, Cheang M. Disease course and outcome of juvenile rheumatoid arthritis in a multicenter cohort. J Rheumatol. 2002;29(9):1989-1999. [PubMed: 12233897]
- 15.
- Packham JC, Hall MA, Pimm TJ. Long-term follow-up of 246 adults with juvenile idiopathic arthritis: predictive factors for mood and pain. Rheumatology (Oxford). 2002;41(12):1444-1449. [PubMed: 12468828]
- 16.
- Zak M, Pedersen FK. Juvenile chronic arthritis into adulthood: a long-term follow-up study. Rheumatology (Oxford). 2000;39(2):198-204. [PubMed: 10725073]
- 17.
- Schanberg LE, Anthony KK, Gil KM, Maurin EC. Daily pain and symptoms in children with polyarticular arthritis. Arthritis Rheum. 2003;48(5):1390-1397. [PubMed: 12746912]
- 18.
- Schanberg LE, Gil KM, Anthony KK, Yow E, Rochon J. Pain, stiffness, and fatigue in juvenile polyarticular arthritis: contemporaneous stressful events and mood as predictors. Arthritis Rheum. 2005;52(4):1196-1204. [PubMed: 15818661]
- 19.
- Schanberg LE, Sandstrom MJ, Starr K, et al. The relationship of daily mood and stressful events to symptoms in juvenile rheumatic disease. Arthritis Care Res (Hoboken). 2000;13(1):33-41. [PubMed: 11094924]
- 20.
- Ringold S, Ward TM, Wallace CA. Disease activity and fatigue in juvenile idiopathic arthritis. Arthritis Care Res (Hoboken). 2013;65(3):391-397. [PMC free article: PMC4117647] [PubMed: 22807336]
- 21.
- Ringold S, Wallace CA, Rivara FP. Health-related quality of life, physical function, fatigue, and disease activity in children with established polyarticular juvenile idiopathic arthritis. J Rheumatol. 2009;36(6):1330-1336. [PubMed: 19411394]
- 22.
- Beukelman T, Ringold S, Davis TE, et al. Disease-modifying antirheumatic drug use in the treatment of juvenile idiopathic arthritis: a cross-sectional analysis of the CARRA Registry. J Rheumatol. 2012;39(9):1867-1874. [PMC free article: PMC3763075] [PubMed: 22859354]
- 23.
- Beukelman T, Patkar NM, Saag KG, et al. 2011 American College of Rheumatology recommendations for the treatment of juvenile idiopathic arthritis: initiation and safety monitoring of therapeutic agents for the treatment of arthritis and systemic features. Arthritis Care Res (Hoboken). 2011;63(4):465-482. [PMC free article: PMC3222233] [PubMed: 21452260]
- 24.
- Ringold S, Angeles-Han ST, Beukelman T, et al. 2019 American College of Rheumatology/Arthritis Foundation guideline for the treatment of juvenile idiopathic arthritis: therapeutic approaches for non-systemic polyarthritis, sacroiliitis, and enthesitis. Arthritis Rheumatol. 2019;71(6):846-863. [PMC free article: PMC6561114] [PubMed: 31021537]
- 25.
- Beukelman T, Guevara JP, Albert DA, Sherry DD, Burnham JM. Variation in the initial treatment of knee monoarthritis in juvenile idiopathic arthritis: a survey of pediatric rheumatologists in the United States and Canada. J Rheumatol. 2007;34(9):1918-1924. [PubMed: 17787051]
- 26.
- Cron RQ, Sharma S, Sherry DD. Current treatment by United States and Canadian pediatric rheumatologists. J Rheumatol. 1999;26(9):2036-2038. [PubMed: 10493688]
- 27.
- Lovell DJ, Giannini EH, Reiff A, et al. Etanercept in children with polyarticular juvenile rheumatoid arthritis. Pediatric Rheumatology Collaborative Study Group. N Engl J Med. 2000;342(11):763-769. [PubMed: 10717011]
- 28.
- Lovell DJ, Ruperto N, Goodman S, et al. Adalimumab with or without methotrexate in juvenile rheumatoid arthritis. N Engl J Med. 2008;359(8):810-820. [PubMed: 18716298]
- 29.
- Ruperto N, Lovell DJ, Quartier P, et al. Abatacept in children with juvenile idiopathic arthritis: a randomised, double-blind, placebo-controlled withdrawal trial. Lancet. 2008;372(9636):383-391. [PubMed: 18632147]
- 30.
- Wallace CA, Giannini EH, Spalding SJ, et al. Trial of early aggressive therapy in polyarticular juvenile idiopathic arthritis. Arthritis Rheum. 2012;64(6):2012-2021. [PMC free article: PMC3319524] [PubMed: 22183975]
- 31.
- Tynjälä P, Vähäsalo P, Tarkiainen M, et al. Aggressive combination drug therapy in very early polyarticular juvenile idiopathic arthritis (ACUTE-JIA): a multicentre randomised open-label clinical trial. Ann Rheum Dis. 2011;70(9):1605-1612. [PubMed: 21623000]
- 32.
- Ringold S, Weiss PF, Colbert RA, et al. Childhood Arthritis and Rheumatology Research Alliance consensus treatment plans for new-onset polyarticular juvenile idiopathic arthritis. Arthritis Care Res (Hoboken). 2014;66(7):1063-1072. [PMC free article: PMC4467832] [PubMed: 24339215]
- 33.
- Sox HC, Greenfield, S. Comparative effectiveness research: a report from the Institute of Medicine. Ann Intern Med. 2010;151(3):203-205. [PubMed: 19567618]
- 34.
- Natter MD, Quan J, Ortiz DM, et al. An i2b2-based, generalizable, open source, self-scaling chronic disease registry. J Am Med Inform Assoc. 2013;20(1):172-179. [PMC free article: PMC3555330] [PubMed: 22733975]
- 35.
- Beukelman T, Kimura Y, Ilowite NT, et al. The new Childhood Arthritis and Rheumatology Research Alliance (CARRA) registry: design, rationale, and characteristics of patients enrolled in the first 12 months. Pediatr Rheumatol Online J. 2017;15(1):30. [PMC free article: PMC5392971] [PubMed: 28416023]
- 36.
- Consolaro A, Ruperto N, Bazso A, et al. Development and validation of a composite disease activity score for juvenile idiopathic arthritis. Arthritis Rheum. 2009;61(5):658-666. [PubMed: 19405003]
- 37.
- Giannini EH, Ruperto N, Ravelli A, Lovell DJ, Felson DT, Martini A. Preliminary definition of improvement in juvenile arthritis. Arthritis Rheum. 1997;40(7):1202-1209. [PubMed: 9214419]
- 38.
- Filocamo G, Consolaro A, Schiappapietra B, et al. A new approach to clinical care of juvenile idiopathic arthritis: the Juvenile Arthritis Multidimensional Assessment Report. J Rheumatol. 2011;38(5):938-953. [PubMed: 21362761]
- 39.
- Varni JW, Sherman SA, Burwinkle TM, Dickinson PE, Dixon P. The PedsQL Family Impact Module: preliminary reliability and validity. Health Qual Life Outcomes. 2004;2:55. [PMC free article: PMC521692] [PubMed: 15450120]
- 40.
- Morgan EM, Mara CA, Huang B, et al. Establishing clinical meaning and defining important differences for Patient-Reported Outcomes Measurement Information System (PROMIS) measures in juvenile idiopathic arthritis using standard setting with patients, parents, and providers. Qual Life Res. 2017;26(3):565-586. [PMC free article: PMC5311023] [PubMed: 27913986]
- 41.
- Mannion ML, Xie F, Curtis JR, Beukelman T. Recent trends in medication usage for the treatment of juvenile idiopathic arthritis and the influence of tumor necrosis factor inhibitors. J Rheumatol. 2014;41(10):2078-2084. [PubMed: 25086081]
- 42.
- Austin PC. An introduction to propensity score methods for reducing the effects of confounding in observational studies. Multivariate Behav Res. 2011;46(3):399-424. [PMC free article: PMC3144483] [PubMed: 21818162]
- 43.
- McCaffrey DF, Griffin BA, Almirall D, Slaughter ME, Ramchand R, Burgette LF. A tutorial on propensity score estimation for multiple treatments using generalized boosted models. Stat Med. 2013;32(19):3388-3414. [PMC free article: PMC3710547] [PubMed: 23508673]
- 44.
- Adelson JL, McCoach DB, Rogers HJ, Adelson JA, Sauer TM. Developing and applying the propensity score to make causal inferences: variable selection and stratification. Front Psychol. 2017;8:1413. [PMC free article: PMC5562725] [PubMed: 28861028]
- 45.
- Ridgeway G, McCaffrey D, Morral A, Griffin BA, Burgette L. twang: toolkit for weighting and analysis of nonequivalent groups. R package version 1.5. 2017. Accessed December 30, 2019. https://CRAN
.R-project.org/package=twang - 46.
- Consolaro A, Trincianti C, van Dijkhuizen P, et al. New JADAS10- and cJADAS10-based cutoffs for juvenile idiopathic arthritis disease activity states: validation in a multinational dataset of 4830 patients [abstract]. Arthritis Rheum. 2018;70(suppl 10). Accessed March 10, 2021. https:
//acrabstracts .org/abstract/new-jadas10-and-cjadas10-based-cutoffs-for-juvenile-idiopathic-arthritis-disease-activity-states-validation-in-a-multinational-dataset-of-4830-patients/ - 47.
- van Buuren S, Groothuis-Oushoorn K. mice: multivariate imputation by chained equations. J Stat Softw. 2011;45(3):1-67.
- 48.
- Swart J, Giancane G, Horneff G, et al. Pharmacovigilance in juvenile idiopathic arthritis patients treated with biologic or synthetic drugs: combined data of more than 15,000 patients from Pharmachild and national registries. Arthritis Res Ther. 2018;20(1):285. [PMC free article: PMC6307151] [PubMed: 30587248]
- 49.
- Ringold S, Nigrovic PA, Feldman BM, et al. The Childhood Arthritis and Rheumatology Research Alliance consensus treatment plans: toward comparative effectiveness in the pediatric rheumatic diseases. Arthritis Rheumatol. 2018;70(5):669-678. [PubMed: 29333701]
- 50.
- Johnson SR, Tomlinson GA, Hawker GA, Granton JT, Feldman BM. Methods to elicit beliefs for Bayesian priors: a systematic review. J Clin Epidemiol. 2010;63(4):355-369. [PubMed: 19716263]
- 51.
- Johnson SR, Tomlinson GA, Hawker GA, Granton JT, Grosbein HA, Feldman BM. A valid and reliable belief elicitation method for Bayesian priors. J Clin Epidemiol. 2010;63(4):370-383. [PubMed: 19926253]
Related Publications
- Kimura Y, Schanberg LE, Tomlinson GA, et al; CARRA STOP-JIA Investigators. The Childhood Arthritis & Rheumatology Research Alliance Start Time Optimization of Biologics in Polyarticular Juvenile Idiopathic Arthritis Study. Arthritis Rheumatol. In press. [PMC free article: PMC8518909] [PubMed: 34105312]
- Sing Ong M, Ringold S, Kimura Y, Schanberg LE, Tomlinson GA, Natter MD, and the CARRA Registry Investigators. Improved disease course associated with early initiation of biologics in new-onset polyarticular juvenile idiopathic arthritis. Arthritis Rheumatol. In press. [PubMed: 34105303]
Acknowledgments
We would like to first acknowledge all the patients and families who participated in this important study.
This work could not have been accomplished without the aid of the NIH's National Institute of Arthritis and Musculoskeletal and Skin Diseases (NIAMS), and the Arthritis Foundation.
We would also like to thank all participants and hospital sites that recruited patients for the CARRA Registry. We thank the following CARRA Registry site principal investigators, subinvestigators, and research coordinators: A. Adams, R. Agbayani, S. Akoghlanian, E. Allenspach, W. Ambler, E. Anderson, S. Ardoin, S. Armendariz, I. Balboni, S. Balevic, L. Ballenger, S. Ballinger, N. Balmuri, F. Barbar-Smiley, M. Basiaga, K. Baszis, M. Becker, H. Bell-Brunson, H. Benham, S. Benseler, W. Bernal, T. Beukelman, T. Bigley, B. Binstadt, M. Blakley, J. Bohnsack, A. Brown, H. Brunner, M. Buckley, D. Bullock, B. Cameron, S. Canna, L. Cannon, V. Cartwright, E. Cassidy, E. Chalom, I. Chang, J. Chang, V. Chauhan, T. Chinn, P. Chira, D. Co, A. Cooper, J. Cooper, C. Correll, R. Cron, L. Curiel-Duran, M. Curry, A. Dalrymple, A. Davis, T. Davis, D. De Ranieri, J. Dean, F. Dedeoglu, M. DeGuzman, V. Dempsey, E. DeSantis, J. Dingle, J. Dowling, J. Drew, K. Driest, Q. Du, D. Durkee, J. Dvergsten, A. Eberhard, M. Eckert, C. Edens, M. Elder, S. Fadrhonc, L. Favier, B. Feldman (author), J. Fennell, P. Ferguson, K. Fields, C. Fleming, L. Fogel, E. Fox, R. Fuhlbrigge, J. Fuller, D. Gerstbacher, M. Gillispie-Taylor, I. Goh, A. Gotte, B. Gottlieb, T. Graham, S. Grevich, T. Griffin, A. Grom, M. Guevara, P. Guittar, M. Guzman, M. Hager, O. Halyabar, M. Hance, S. Haro, J. Harris, J. Hausmann, K. Hayward, J. Heiart, L. Henderson, M. Henrickson, A. Hersh, L. Hiraki, M. Hiskey, P. Hobday, C. Hoffart, M. Holland, M. Hollander, S. Hong, M. Horwitz, J. Hsu, A. Huber, J. Huggins, J. Hui-Yuen, A. Huttenlocher, M. Ibarra, C. Inman, H. Jackson, S. Jackson, K. James, G. Janow, J. Jaquith, S. Jared, N. Johnson, J. Jones, K. Jones, S. Jones, S. Joshi, C. Justice, K. Kaufman, U. Khalsa, B. Kienzle, S. Kim, Y. Kimura (author), D. Kingsbury, M. Kitcharoensakkul, T. Klausmeier, K. Klein, M. Klein-Gitelman, S. Kramer, C. Kremer, J. Lai, B. Lang, S. Lapidus, A. Lasky, E. Lawson, R. Laxer, P. Lee, P. Lee, T. Lee, M. Lerman, D. Levy, S. Li, S. Lieberman, C. Lin, N. Ling, M. Lo, D. Lovell, N. Luca, B. Malla, J. Maller, M. Mannion, A. Martyniuk, T. Mason, K. Mcallister, L. McAllister, K. McConnell, I. McHale, E. Meidan, E. Mellins, P. Miettunen, M. Miller, M. Mitchell, R. Modica, K. Moore, E. Morgan Dewitt, T. Moussa, V. Mruk, E. Muscal, K. Nanda, L. Nassi, S. Nativ, J. Neely, B. Nelson, L. Newhall, P. Nigrovic, B. Nolan, E. Oberle, O. Okeke, M. Oliver, K. O'Neil, K. Onel, A. Orandi, M. Orlando, R. Oz, E. Pagano, A. Paller, N. Pan, J. Patel, P. Pepmueller, R. Pooni, S. Protopapas, B. Puplava, J. Quach, C. Rabinovich, S. Radhakrishna, S. Ramsey, R. Randell, A. Reed, H. Reid, A. Richmond, S. Ringold (author), M. Riordan (author), M. Riskalla, M. Ritter, M. Rodriquez, K. Rojas, M. Rosenkranz, T. Rubinstein, N. Saad, R. Sadun, C. Sandborg, L. Schanberg (author), K. Schikler, H. Schmeling, K. Schmidt, E. Schmitt, R. Schneider, G. Schulert, T. Seay, C. Seper, J. Shalen, R. Sheets, S. Shenoi, J. Shirley, E. Silverman, V. Sivaraman, C. Smith, J. Smith, E. Smitherman, J. Soep, M. Son, S. Spence, L. Spiegel, J. Spitznagle, H. Stapp, K. Steigerwald, S. Stern, A. Stevens, B. Stevens, R. Stevenson, K. Stewart, C. Stingl, M. Stoll, E. Stringer, J. Sumner, R. Sundel, M. Sutter, R. Syed, S. Taber, T. Tanner, G. Tarshish, S. Tarvin, J. Taylor, M. Tesher, A. Thatayatikom, B. Thomas, T. Ting, K. Torok, C. Toruner, S. Tse, M. Twilt, T. Valcarcel, H. Van Mater, N. Vasquez, R. Vehe, K. Veiga, J. Velez, N. Volpe, E. von Scheven, S. Vora, L. Wagner-Weiner, D. Wahezi, H. Walters, M. Waterfield, A. Watts, P. Weiser, J. Weiss, P.F. Weiss (author), A. White, L. Woolnough, T. Wright, M. Yee, R. Yeung, K. Yomogida, Y. Zhang, Y. Zhao, and A. Zhu.
Additionally, we would like to acknowledge the DCRI CARRA Registry staff, without whom this study could not have succeeded, especially Kristin Siebenaler, Renee Leverty, Cheryl Rorick, Alan Russell, Rebecca Mark, and Nina Hines.
We would also like to thank Juan Pablo Diaz Martinez for his biostatistical assistance.
Last, we would like to acknowledge our SAP members, who worked together with our study team to make our study accessible and understandable to our patients and families. Our SAP included Janet Hobble, Melanie Kohlheim, Charla Carey, Nick Yongjay Kim, Lauren Revis, Kathryn Kuhns, Uday Deshmukh, Jennifer Horonjeff, and Suzanne Schrandt, in addition to our PPs who are listed as authors on this report.
Research reported in this report was funded through a Patient-Centered Outcomes Research Institute® (PCORI®) Award (CER-1408-2053). Further information available at: https://www.pcori.org/research-results/2015/comparing-three-ways-start-treatment-patients-polyarticular-juvenile
Appendices
Appendix A.
CARRA Registry Protocol (PDF, 564K)
Appendix B.
STOP-JIA Dissemination Plan (PDF, 96K)
Appendix C.
STOP-JIA Study Guide (PDF, 348K)
Appendix D.
Site Map (PDF, 141K)
Appendix E.
List of Sites and CTP Use by Site (PDF, 375K)
Appendix F.
Bayesian Logistic Regression Analysis (PDF, 491K)
Appendix G.
Propensity Scoring Results (PDF, 587K)
Appendix H.
Results of Other Patient-Reported Outcome Measures (PDF, 981K)
Appendix I.
Frequency of Medications at Baseline (by CTP Group) (PDF, 93K)
Appendix J.
Reasons For Choosing CTP (PDF, 114K)
Suggested citation:
Kimura Y, Ringold S, Riordan ME, et al. (2021). Comparing Three Ways to Start Treatment for Patients with Polyarticular Juvenile Idiopathic Arthritis—The Stop-JIA Trial. Patient-Centered Outcomes Research Institute (PCORI). https://doi.org/10.25302/03.2021.CER.14082053
Disclaimer
The [views, statements, opinions] presented in this report are solely the responsibility of the author(s) and do not necessarily represent the views of the Patient-Centered Outcomes Research Institute® (PCORI®), its Board of Governors or Methodology Committee.
- Optimizing the Start Time of Biologics in Polyarticular Juvenile Idiopathic Arthritis: A Comparative Effectiveness Study of Childhood Arthritis and Rheumatology Research Alliance Consensus Treatment Plans.[Arthritis Rheumatol. 2021]Optimizing the Start Time of Biologics in Polyarticular Juvenile Idiopathic Arthritis: A Comparative Effectiveness Study of Childhood Arthritis and Rheumatology Research Alliance Consensus Treatment Plans.Kimura Y, Schanberg LE, Tomlinson GA, Riordan ME, Dennos AC, Del Gaizo V, Murphy KL, Weiss PF, Natter MD, Feldman BM, et al. Arthritis Rheumatol. 2021 Oct; 73(10):1898-1909. Epub 2021 Sep 3.
- Pilot study comparing the Childhood Arthritis & Rheumatology Research Alliance (CARRA) systemic Juvenile Idiopathic Arthritis Consensus Treatment Plans.[Pediatr Rheumatol Online J. 2017]Pilot study comparing the Childhood Arthritis & Rheumatology Research Alliance (CARRA) systemic Juvenile Idiopathic Arthritis Consensus Treatment Plans.Kimura Y, Grevich S, Beukelman T, Morgan E, Nigrovic PA, Mieszkalski K, Graham TB, Ibarra M, Ilowite N, Klein-Gitelman M, et al. Pediatr Rheumatol Online J. 2017 Apr 11; 15(1):23. Epub 2017 Apr 11.
- Review New Statistical Methods to Compare the Effectiveness of Adaptive Treatment Plans[ 2020]Review New Statistical Methods to Compare the Effectiveness of Adaptive Treatment PlansHuang B, Morgan EM, Chen C, Qiu T, Adams M, Zhang Y, Sivaganisan S, Seid M, Guo J, Lovell DJ, et al. 2020 Nov
- Childhood Arthritis and Rheumatology Research Alliance consensus treatment plans for new-onset polyarticular juvenile idiopathic arthritis.[Arthritis Care Res (Hoboken). ...]Childhood Arthritis and Rheumatology Research Alliance consensus treatment plans for new-onset polyarticular juvenile idiopathic arthritis.Ringold S, Weiss PF, Colbert RA, DeWitt EM, Lee T, Onel K, Prahalad S, Schneider R, Shenoi S, Vehe RK, et al. Arthritis Care Res (Hoboken). 2014 Jul; 66(7):1063-72.
- Review Protocols on classification, monitoring and therapy in children's rheumatology (PRO-KIND): results of the working group Polyarticular juvenile idiopathic arthritis.[Pediatr Rheumatol Online J. 2017]Review Protocols on classification, monitoring and therapy in children's rheumatology (PRO-KIND): results of the working group Polyarticular juvenile idiopathic arthritis.Horneff G, Klein A, Ganser G, Sailer-Höck M, Günther A, Foeldvari I, Weller-Heinemann F. Pediatr Rheumatol Online J. 2017 Nov 7; 15(1):78. Epub 2017 Nov 7.
- Comparing Three Ways to Start Treatment for Patients with Polyarticular Juvenile...Comparing Three Ways to Start Treatment for Patients with Polyarticular Juvenile Idiopathic Arthritis—The Stop-JIA Trial
Your browsing activity is empty.
Activity recording is turned off.
See more...