NCBI Bookshelf. A service of the National Library of Medicine, National Institutes of Health.
Johnson BT, Huedo-Medina TB. Meta-Analytic Statistical Inferences for Continuous Measure Outcomes as a Function of Effect Size Metric and Other Assumptions [Internet]. Rockville (MD): Agency for Healthcare Research and Quality (US); 2013 Apr.
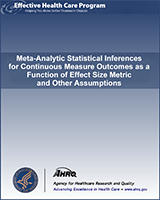
Meta-Analytic Statistical Inferences for Continuous Measure Outcomes as a Function of Effect Size Metric and Other Assumptions [Internet].
Show detailsGeneral Design
- The data sets from a collection of k single studies were randomly generated using commands from the statistical software R, version 2.14.1. Two independent normal distributions were simulated for conditions in part 1; and for the conditions in part 2 that do not require repeated-measures design, R’s rnorm command was used. Two bivariate normal distributions were generated specifically for part 2 when repeated-measures two-groups conditions were simulated using the R command mvnorm. (Both of these R commands rely on the Marsenne-Twister27 random number generator.) The variance-covariance matrix was manipulated with the identity matrix being a particular condition when groups of scores are not correlated and homogeneous variances equal to one are assumed between groups and time measures; the appropriate matrix was generated to create heterogeneous distributions for each group and time measure. The distributions were modified in some conditions, as we describe below.
- We generated two bivariate normal distributions, each with a homogeneous variance-covariance matrix,representing the experimental and control groups, respectively; only the YE matrix was generated in the case of single-group designs. The parameters for these distributions in the standardized units are , with σPre,Post, and being manipulated factors in the simulation. These values were permitted to remain in their unstandardized units to create a comparison for statistical inferences between raw and standardized conditions.
- The necessary basic statistics (i.e., means, standard deviations, correlations) were estimated from the sampling data for each method using basic R commands. Thus, the estimates of ES and the ES sampling variance were calculated using all the effect size equations from Tables 1, 2, and 5, as relevant.
- The calculations for the estimations, and their sampling variances were repeated for each simulated study (and comparing the equations in Tables 1–5; note that the between-groups sampling variance is the same as the raw-score metric, that is, Table 4, no. 15).
- In order to evaluate the robustness of the estimates under different conditions the bias of the estimate was calculated:
- The efficiency of the estimates was obtained as the variability of the estimate across replications,
- Finally, the particular formulas for estimating the sampling variances of each index of specific aim 2 were computed in each replication and their values averaged over the 10,000 replications of the same condition. The average of the empirical variability of each index was compared with the average of the variance obtained from each sampling variance estimate to obtain the adjustment to the theoretical variance.
Conditions Manipulated
Monte Carlo simulations established many different conditions by crossing these factors (see Table 6) to evaluate Specific Aim 1:
Table 6
Characteristics of simulated datasets.
- The number of studies in a meta-analysis, k = 10, 20, 50, and 100.
- The mean sample size (N) in the literature. The mean sample size for each generated meta-analysis replicated the 10th (N = 30), 40th (N = 50), and 80th (N = 80) percentiles of the sample sizes from HIV prevention trials in the Syntheses of HIV and AIDS Research Project meta-analytic database at the University of Connecticut, which summarizes over 700 trials. Three vectors of sample sizes were generated as [12, 16, 18, 20, 84], [32, 36, 38, 40, 104], and [62, 66, 68, 70, 134], one for each selected averaging 30, 50, and 80, respectively. Each vector was replicated either 2, 4, 10, or 50 times for meta-analyses of k = 10, 20, 50, and 100.
- The within-study variances for experimental and control groups and at pretest and post-test measures were varied using ratios for experimental and control groups, respectively, of 1:1, 2:1, and 4:1.28,29 The variance of the experimental group was increased in comparison to that of the control group because increases in variability are more plausible when there is experimental manipulation (e.g., a psychological treatment) and doing so permitted clearer inferences about results.11
- The mean of the post-test for the experimental group, following the parametric values for the standardized mean difference,30 , 0.5, and 0.8. The means and standard deviations of the scores for the experimental and control participants in each pseudo-study were generated assuming a variety of different distributions: both normal distributions and nonnormal distributions:
- For the normal distributions, values for means and standard deviations were kept as following the parametric normal distributions described above.
- To generate nonnormal distributions, the normality pattern was manipulated to obtain skewed distributions through use of the Fleishman31 algorithm, with the following values of skewness/kurtosis: 0.5/0, 0.75/0, and 1.75/3.75.
Condition Manipulated Specifically for Specific Aim 2
- 6.
The correlations between the two conditions were manipulated through the variance-covariance matrix, where homogeneous variances equal to 1 can be assumed. The manipulated correlation was equal to the covariance between the two measures. The values were ρpre-post = 0, 0.25, 0.50, and 0.75.
- Methods - Meta-Analytic Statistical Inferences for Continuous Measure Outcomes a...Methods - Meta-Analytic Statistical Inferences for Continuous Measure Outcomes as a Function of Effect Size Metric and Other Assumptions
Your browsing activity is empty.
Activity recording is turned off.
See more...