NCBI Bookshelf. A service of the National Library of Medicine, National Institutes of Health.
Johnson BT, Huedo-Medina TB. Meta-Analytic Statistical Inferences for Continuous Measure Outcomes as a Function of Effect Size Metric and Other Assumptions [Internet]. Rockville (MD): Agency for Healthcare Research and Quality (US); 2013 Apr.
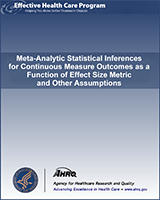
Meta-Analytic Statistical Inferences for Continuous Measure Outcomes as a Function of Effect Size Metric and Other Assumptions [Internet].
Show detailsBackground
Over the past 30 years, meta-analytic methods to accumulate knowledge have experienced a sharp increase in use across the sciences, and have been applied to many topics of high import to public health. Using meta-analysis, the result of every study is quantified by means of a statistical index that one can apply to all studies in a given literature, thereby enabling a comprehensive summary of the magnitude of the effect in every study and analyses of outcomes according to coded study features.1–11 Conventionally, meta-analysis has three main objectives: (1) synthesizing different studies’ effect size values to obtain a weighted mean, (2) assessing the consistency of the results, and (3) in the case of inconsistency (or heterogeneity), using moderator variables in an attempt to explain the variability. To do their work, meta-analysts must complete a series of interrelated steps: (1) conceptually define the topic of the review, (2) set selection criteria for the sample of studies, (3) comprehensively search for qualified studies, (4) code studies for their distinctive substantive, methodological and external characteristics, (5) represent the magnitude of each study’s effect on the same metric, (6) analyze the database, and (7) interpret and present the results. To the extent that meta-analysts have the best available techniques to complete each step, the accuracy of their conclusions will be enhanced; science and its applications can accumulate and report its research findings in a more efficient manner. The current report focuses on the fifth and sixth steps as applied to literatures of studies that report outcomes on a single continuous outcome. Thus, dichotomous outcomes are outside the scope of this study, as are literatures of studies for which continuous outcomes are measured on a variety of measures.
Statistical modeling in meta-analysis cannot proceed unless each study outcome is represented on the same metric and has an appropriate sampling variance estimate, the inverse of which is used as the weight for each study result in meta-regression and other meta-analytic statistics (see Tables 1 through 5). In contemporary practice, when comparing treatments for trials that use the same continuous measures across studies, meta-analyses routinely use the original or unstandardized mean difference (UMD) to model the difference between the observed means (i.e., ME−MC) rather than representing effects in the standardized mean difference (SMD). A fundamental difference between the two strategies is that the UMD incorporates the observed variance of the measures as a component of the analytical weights (viz., sampling error or inverse variance) in statistically modeling the results for each study. In contrast, the SMD incorporates the measure’s variance directly in the effect size (ES) itself (i.e., SMD=[ME−MC]/SD; e.g., see equation 6, Table 2) and not directly in the analytical weights. In effect, a UMD approach to meta-analysis (see equation 21, Table 5) more heavily weights individual studies’ differences to the extent that they have smaller observed variances and larger samples of observations. A SMD approach to meta-analysis more heavily weights studies’ differences to the extent that they have larger samples (equation 22, Table 5); the pooled standard deviation observed for each study is used to create the standardized difference between conditions (equation 6, Table 2). The UMD approach has been conventional even though its bias and efficiency are unknown and have not been compared with those of the SMD. Also unresolved is which of many possible available equations best optimize statistical modeling for the UMD and SMD (Tables 3 and 4).
Table 1
Standardized mean difference ES estimations (and their components) for a one-group repeated-measures design.
Table 2
Standardized mean difference (SMD) ES estimations (and their components) for two independent groups.
Table 3
Estimates of sampling variance for the SMD ES in the one-group design with repeated-measures.
Table 4
Estimates of sampling variances for the SMD ES from two-group designs with repeated-measures.
Table 5
Statistics related to the standardized mean difference (SMD) and unstandardized mean difference (UMD) for designs with two independent groups and continuous measures.
Another important and controversial issue is specifically related to the SMD. This estimator is used to measure the degree of change between repeated measures or the difference between two groups, using a standardization that can vary depending on the standard deviation used, with the assumption that the measures follow a normal distribution. In its between-groups form, SMD can be calculated from any two groups whether they are experimental or not; it is assumed that the individuals in the compared groups are independent. In its repeated-measures or within-subjects form, the SMD assumes that the observations are dependent, and while some extant meta- analytic procedures account for this dependency, many others do not (Tables 3 and 4); scholars will often integrate both types of estimates in a single meta-analysis. Similarly, the numerous methods of calculating the SMD and their variances are known to produce discordant results (Tables 3 and 4).12,13
In summary, it is unknown how much bias appears in the weighted effect sizes and moderator analyses when two-groups, two-groups repeated measures, or single groups with repeated measures are integrated without incorporating assumptions about possible dependence arising from the those observations with repeated measures. Further, it is not clear in the literature if the different methods of transformation to standardized mean difference from different statistical information types are equivalent across design types. There is conflicting advice about which specific technique equations to invoke when trials assess an outcome on the same measure and/or evaluate outcomes using repeated measures versus between-groups (or mixed) designs.
Objectives
This report has two objectives:
- 1.
Determine the bias and efficiency of the unstandardized mean difference (UMD) relative to the standardized mean difference (SMD) under a wide range of analytic circumstances.
In groups of studies for which a phenomenon is assessed using the same measure in every study, meta-analysts have the choice of examining either standardized effect sizes or leaving study outcomes in the original, unstandardized, measure.14 For example, blood pressure is always assessed in metric units (usually mmHg, or millimeters of mercury) and meta-analyses of blood pressure outcomes routinely leave it in these units, showing, say, that aerobic exercise lowers systolic blood pressure an average of 6 mmHg relative to controls. Efficacy in antidepressant trials is routinely assessed on the Hamilton Rating Scale of Depression (HAM-D), and many meta-analyses examine it in this metric. Analysts typically leave study results in the original unstandardized measure in order to facilitate their interpretability. Many prominent statisticians have even recommended leaving comparisons in unstandardized units in order to facilitate comparisons between studies.15,16
Nonetheless, the assumptions underlying such advice must be evaluated. For example, they had primary-level studies in mind rather than comparisons of the results of independent studies, such as is the case in meta-analysis. One issue has to do with unequal variances across studies. Homogeneity of compared group variances in primary-level research is an analogous assumption to the problem that appears in terms of between-studies heterogeneity in observed measurement variances. For example, antidepressant trials focusing on very severely depressed individuals (e.g., M HAM-D=33) will typically have much larger standard deviations than trials that focus on moderately depressed individuals (e.g., M HAM-D=17). Change of, say, 6 units on the HAM-D is more dramatic change for a sample with a small standard deviation than for a sample with a large one. Similarly, parametric inferential statistics, the most developed and used methods in meta-analysis, routinely must meet the normality assumption (lack of skewness and kurtosis). For an example, see Pedhauzer, 1997.17
Weights for unstandardized outcomes in meta-analysis routinely use the sample size and the variance (see Table 5),14,18 but it is unclear whether meta-analytic inferences will be equivalent for the two solutions. To date, no research has examined the comparability of statistical inferences between the UMD and SMD. In the current work, we consider the case of a design that compares two independent groups such as an experimental group and a control group.
The second objective of the current project is:
- 2.
Determine the best techniques to calculate SMD effect size estimates and their sampling variances under different design and parametric conditions.
Statistical modeling in meta-analysis cannot proceed unless each study outcome is on the same metric and an appropriate sampling variance is calculated. As Tables 1 and 2 show, current meta-analytic methods yield conflicting advice about which specific techniques to invoke when the outcomes are provided from different designs, specifically, within-, between-subjects, or mixed-designs, again with the result that significance testing and interpretation may vary depending on how they are integrated.
An effect size estimator is used to measure the degree of change between repeated measures or to compare the difference between two or more groups, with the assumption that the measures follow a normal distribution. In its between-groups form, the ES estimator can be calculated from any two groups whether they are experimental or not; it is assumed that the individuals in the compared groups are independent. To the extent that the ES deviates from the null value, it reflects a greater difference between the groups. In its repeated-measures or within-subjects form, the ES estimator assumes that the observations are dependent, and while some meta-analytic procedures account for this dependency, many others do not; scholars often integrate both types of estimates in a single meta-analysis or they more simply focus on post-test results without incorporating baseline measures. Similarly, there are numerous methods of calculating the ES when the outcome is continuous and their available variances are known to vary.12,13 Further, the literature leaves unclear whether the different methods of transformation to standardized mean difference from different statistical information types are equivalent and perform well under different parametric conditions.19–22
Orientation to Method
For both specific aims, Monte Carlo simulation studies are used to generate data under a wide variety of conditions to determine the extent to which parameter estimates, sample sizes, and number of studies are unbiased and their standard errors efficient. The simulations will (1) evaluate the differences between using unstandardized versus standardized metric of effect size (objective 1); and (2) evaluate current solutions to estimate the ES and its sampling variance, differentiating among three main design types (i.e., two-groups, two-groups repeated-measures, and repeated measures design) (objective 2). The simulations gauge the performance of these methods of estimation for both objectives.
Significance of Project
The goals of this project are relevant to any empirical literature that has systematic observations; these concern statistical operations that are very commonly used in contemporary practice. Even if it turns out that meta-analytic statistics in the original metric are robust to underlying deviations in the variance of the measures, the results of this investigation are of great interest. If meta-analytic statistics and inferences do depend on choice of unstandardized vs. standardized effect sizes under some circumstances, then the findings may have far-ranging implications for the practice of meta-analysis. Moreover, it is also important to know the best estimates of within-subjects ESs (in single-and in two-group designs) and to determine which estimates of variance are best for use in conducting weighted analyses and when those two types of designs can be combined in a single meta-analytic database. Knowing how well each effect size index for each design performs will enable future analysts a better choice of the most appropriate operations and, as a consequence, permit more studies to be integrated and more accurate meta-analytic results. Thus, this methodological study offers considerable potential to improve the accuracy and progress of science and public health. An overarching goal is to enable more accurate empirical generalizations.
- Introduction - Meta-Analytic Statistical Inferences for Continuous Measure Outco...Introduction - Meta-Analytic Statistical Inferences for Continuous Measure Outcomes as a Function of Effect Size Metric and Other Assumptions
Your browsing activity is empty.
Activity recording is turned off.
See more...