This book is distributed under the terms of the Creative Commons Attribution-NonCommercial-NoDerivs License which permits noncommercial use and distribution provided the original author(s) and source are credited. (See https://creativecommons.org/licenses/by-nc-nd/4.0/
NCBI Bookshelf. A service of the National Library of Medicine, National Institutes of Health.
Structured Abstract
Background:
There are many clinical prediction models (CPMs) available to inform treatment decisions, but little is known about the broad trustworthiness of these models and whether their performance is likely to improve clinical care.
Objectives:
To (1) describe the current state of the literature on validations of cardiovascular CPMs; (2) understand how well CPMs validate on independent data sets generally, with particular attention to calibration; (3) understand when models have the potential to worsen decision-making and cause patient harm; and (4) understand the effectiveness of various updating procedures across a broad array of models.
Methods:
A citation search was run on March 22, 2017, to identify external validations of the 1382 cardiovascular CPMs in the Tufts Predictive Analytics and Comparative Effectiveness Center (PACE) CPM Registry. We assessed the extent of external validation and the variation in performance across data sets, focusing on the percentage change in the C statistic on external validation compared with derivation. To evaluate factors that influence this CPM performance, we assessed the “relatedness” of the data sets used for validation to those on which the model was developed, using a set of detailed rubrics we developed for each clinical condition. To assess whether adherence to methodological standards influenced performance, we developed an abbreviated version of the Prediction model Risk Of Bias ASsessment Tool (PROBAST), here called the “short form.” Finally, we performed independent validations on a set of CPMs across 3 index conditions—acute coronary syndrome, heart failure, and incident cardiovascular disease—using publicly available clinical trial data and an evaluation framework, with both novel and conventional performance measures, including a model-based C statistic to estimate the loss in discrimination due to case mix rather than model invalidity, the Harrell E statistic (standardized EAVG) to quantify the magnitude of calibration error, and net benefit based on decision curve analysis (DCA) at 3 thresholds (the outcome rate, half the outcome rate, and twice the outcome rate). We then examined the effectiveness of model updating procedures for reducing the risk of harmful predictions, defined as predictions yielding a negative net benefit at one of the defined thresholds, where harm is identified through DCA.
Results:
A total of 2030 external validations of 1382 CPMs were identified: 1.5 validations per CPM (range, 0-94). Of the CPMs, 807 (58%) have never been externally validated. The median external validation area under the curve was 0.73 (interquartile range [IQR], 0.66-0.79), representing a median percentage change in discrimination of −11.1% (IQR, −32.4% to 2.7%) compared with performance on derivation data. For individual CPMs evaluated more than once, there was typically large variation in performance from 1 data set to another. The percentage change in discrimination from derivation set to validation set was −3.7% (IQR, −13.2 to 3.1) for “closely related” validation sets, −9.0% (IQR, −27.6 to 3.9) for “related validation sets,” and −17.2% (IQR, −42.3 to 0) for “distantly related” validation sets (P < .001). Agreement between the short form (ie, the abbreviated PROBAST) and the full PROBAST for low vs high risk of bias (ROB) was 98% (κ = 0.79), with perfect specificity for high ROB. Using the short form, 529 of 556 CPMs (95%) were considered high ROB, 20 (3.6%) low ROB, and 7 (1.3%) unclear ROB. The median change in discrimination was less in low ROB models (−0.9%; IQR, −6.2% to −4.2%) compared with high ROB models (−12%; IQR, −33% to 2.6%). We performed a total of 158 unique validations on 108 unique CPM models across 36 data sets. The median percentage decrease in the C statistic among 57 related validation data sets was 30% (IQR, −45% to −16%) and, among 101 distantly related data sets, the decrease was 55% (IQR, −68% to −40%; P < .001). The decrease was due both to a narrower case mix and to model invalidity: compared with the model-based C statistic, in related data sets, the median decrease in the validation C statistic was 11% (IQR, −25% to 0%) and 33% (IQR, −50% to −13%) in those distantly related. The median standardized EAVG was 0.5 (IQR, 0.4-0.7), indicating an average error that is half the average risk, and was not different based on relatedness. When we used DCA, only 22 of 132 (17%) of the unique evaluations we performed were either beneficial or neutral at all 3 decision thresholds examined. The risk of harm was most salient when decision thresholds departed substantially from the average risk in the target population. Updating the intercept alone prevented harm for decision thresholds at the outcome rate across all models, but the risk of harm remained substantial at the more extreme thresholds unless both the slope and intercept were updated. Findings were consistent across the 3 index conditions we tested.
Conclusions:
Many published cardiovascular CPMs have never been externally validated. Discrimination and calibration often decrease significantly when CPMs for primary prevention of cardiovascular disease are tested in external populations, leading to substantial risk of harm. Model updating can reduce this risk substantially and will likely be needed to realize the full potential of risk-based decision-making.
Limitations:
Our analysis of published validation studies (aim 1) was limited to changes in the C statistic, because this was the only performance measure routinely reported. The sample of data sets used in aims 2 and 3 was a convenience sample and this sample determined the CPMs selected.
Background
Although personalized medicine has become closely identified with genomics,1 it has been more usefully and broadly defined as “the practice of clinical decision making such that the decisions made maximize the outcomes that the patient most cares about and minimizes those that the patient fears the most, on the basis of as much knowledge about the individual's state as is available [emphasis added].”2 This definition captures the shared mission of personalized (or stratified or precision) medicine and patient-centered care. Prediction—that is, answering the first of PCORI's patient-centered research questions (“Given my personal characteristics, conditions, and preferences, what should I expect will happen to me?”3)—remains perhaps the most fundamental challenge facing patient-centered outcomes research.
Clinical prediction models (CPMs) are intended to address this question. CPMs are multivariable statistical algorithms that produce patient-specific estimates of clinically important outcome risks based on ascertainable clinical and laboratory characteristics. CPMs are increasingly common and important tools for patient-centered outcomes research and clinical care. By providing evidence-based estimates of the probability of health outcomes at a more patient-specific level, CPMs enable clinicians and patients to make decisions that are more rational and consistent with an individual patient's own risks, values, and preferences. CPMs can also be applied in comparative effectiveness studies to analyze heterogeneity of effects, and they offer advantages over conventionally defined (ie, 1 variable at a time) subgroups.4-8 CPMs thus have the potential to greatly enhance the efficiency of clinical decision-making by improving the targeting of risky or costly therapies.
Recent reviews have demonstrated the abundance of CPMs in the literature but have also pointed at shortcomings.9 Our own database, the Tufts Predictive Analytics and Comparative Effectiveness (PACE) Center CPM Registry,10 currently includes 1382 CPMs just for patients with cardiovascular disease (CVD), including 333 CPMs for patients with coronary artery disease, 227 for population-based samples (ie, predicting incident CVD), and 149 for patients with heart failure (HF). In this registry, we have observed a continued increase in CPMs for CVD, despite the substantial apparent redundancy of models.11 The increase in the literature reflects the increasing ease with which these models can be developed. With increasing access to research and clinical data sets, in addition to the broad availability of software packages, barriers to developing new models are rapidly diminishing. Although the vast majority of CPMs are not widely applied in practice, they are increasingly incorporated into clinical decisions.12 A recent horizon scan found that of 133 clinical practice guidelines for various chronic diseases, only 20 (15%) incorporated CPMs for care recommendations, but these were generally poorly justified and of unclear benefit.13
Despite the great potential of CPMs to advance patient-centered and evidence-based decision-making, substantial challenges remain, both in achieving accurate predictions and in translating CPMs into practice. For CPMs to be beneficial, they must yield accurate predictions about new cohorts (ie, external validation). There are various ways to assess the performance of a statistical prediction model, as summarized in Table 1 (adapted from Steyerberg et al14). One approach is to quantify how close predictions are to actual outcomes, using measures such as explained variation (eg, R2 statistics) and the Brier score.15 More typically, performance is decomposed into discrimination (Do patients with the outcome have higher risk predictions than those without?), usually quantified with a concordance statistic (ie, the C statistic), and calibration (Do x of 100 patients with a risk prediction of x% have the outcome?). An important limitation of these measures is that results tend to be difficult to interpret clinically. The C statistic of a CPM—the most widely reported of all CPM performance measures—may range from 0.5 (ie, no better than the flip of a coin) to 1 (ie, perfect discrimination), but we do not know how high discrimination must be to be considered “high enough” or “useful.” Moreover, discrimination is unaffected by whether a prediction is well calibrated.16
Table 1
Characteristics of Some Traditional and Novel Performance Measures.
Despite the increasing number of CPMs in the literature, how models perform generally on external validation and the determinants (eg, sample size of derivation cohort, adherence to best modeling practice, relatedness of the validation cohort) of that performance are largely unknown. Most CPMs have not been validated in external cohorts, and those that have been usually were validated on a single external cohort.11,17 Validation exercises are also infrequently performed completely independently from model development (ie, development and testing are often performed by the same team). The lack of independent and external validation is likely to make the literature misleading (ie, overly optimistic) in terms of model performance. Moreover, it is known that when models are externally validated, their performance degrades both in terms of discrimination and calibration.18 Model calibration is also infrequently reported19 and, unless the calibration is known to be excellent, CPMs may lead to harm if they are used to inform decisions at certain risk thresholds, because poorly calibrated models yield misinformation that can lead to clinical decision-making that is worse than when using best “on average” information.20,21 There is also evidence that single validation exercises are insufficient to broadly understand CPM performance across various data sets.22 For example, when performance in a new data set is poor, it can be due to model invalidity, on the one hand, or it can be because the new data set is not closely related to the derivation sample or because the case mix in the external data set is substantially restricted compared with the derivation data set. Finally, there are no widely accepted criteria by which one can claim that a model has been validated. Generally, the term validation is used to indicate models that have been evaluated using external data, without reference to a specific performance standard that a model should surpass. We use the term similarly in this report while acknowledging that so-called validated models may not be suitable for clinical care, because they discriminate poorly.
This decrement in performance can significantly threaten the utility of many CPMs by leading to harmful treatment decisions if the models are used naively in routine clinical practice. An illustrative example is the controversy surrounding the use of the pooled cohort equations23 (PCEs) to guide primary prevention statin therapy. Guidelines recommend statin therapy for individuals with a PCE-estimated 10-year CVD risk of ≥7.5%,24 but once it was shown that the PCE systematically overestimated risk in several populations—potentially resulting in significant overtreatment—some argued for a retreat to “one-size-fits-all” evidence-based medicine. It was suggested that outcomes might be better using trial-based guidelines, which emphasize applying the best treatment on average to populations defined by trial inclusion and exclusion criteria, instead of risk-based guidelines.25 Of course, the benefits of risk-based approaches, compared with trial-based approaches, depend on the specifics of each case and, in particular, the quality of the risk predictions.
The evaluation of CPM performance also tends to focus on the quality of predictions (ie, measures of statistical accuracy, such as discrimination) rather than the quality of decisions (ie, clinical utility–based measures).14 Even when considering statistical measures of accuracy, measures of discrimination are emphasized much more than measures of calibration,10,18,19 although calibration is frequently less stable than discrimination, and miscalibration can lead to poor decisions. Recently, several new measures have been proposed to facilitate model-to-model comparison and assess performance of a CPM for decision support. These include reclassification tables26 and net reclassification improvement,27 as well as decision-analytic measures, such as decision curves.14,28 Decision curve analysis (DCA)28-30 quantifies the clinical usefulness of a CPM (or the incremental value of a new risk marker) using a formal decision analytic perspective. Decision curves plot the net benefit (NB) of using a CPM on the y-axis, which can be interpreted as the number of true-positive (TP) predictions per patient penalized for the false-positive (FP) predictions across various decision thresholds (plotted on the x-axis) and weighted by their relative importance. This can be done because any decision threshold implicitly determines the relative importance of TP and FP predictions, according to a classic equation first attributed to Peirce.31 (A full description of NB is provided in the Methods section.) More routine use of measures that are oriented to clinical decisions can lead to novel insights about the value and, in particular, the potential harms of applying CPMs to influence patient-centered decisions. Of course, the most rigorous evaluation of the impact of CPMs in clinical care would be a randomized trial comparing either decisions or clinical outcomes between groups in the allocated to-use or not-use model predictions, but these are rarely conducted.
The CPM research community has made considerable efforts to improve standards for conducting and reporting prediction modeling research. In recent years, there has been a movement to establish standards for CPM development and testing,12,32-34 and to establish guidelines to promote transparency of model reporting.35-37 Most recently, a novel tool for assessing risk of bias (ROB) and applicability of diagnostic and prognostic models was developed by a steering group. The Prediction model Risk Of Bias ASsessment Tool (PROBAST)37,38 is intended to facilitate structured judgment of ROB, though it is unclear if this quality assessment can identify models that are likely to perform poorly on external validation.
Realizing the potential of CPMs to better individualize patient care is likely to require rigorous evidentiary standards, yet to date there is no standard evaluation framework for CPMs. For example, there are no PCORI methodology standards for CPM development and evaluation, although several PCORI-funded studies have evaluated decision tools with embedded CPMs.39-41 Dissemination of inaccurate models poses a substantial public health risk and undermines the great potential for benefit from individualizing evidence-based care. Broad changes are thus required in the processes by which CPMs are evaluated before their full potential to influence care can be realized.
The short-term objectives of this work are to (1) describe the current state of the literature on validations of cardiovascular CPMs; (2) understand how well CPMs validate on independent data sets generally, with particular attention to calibration; (3) understand when models have the potential to worsen decision-making and cause patient harm; and (4) understand the effectiveness of various updating procedures across a broad array of models. We also seek to gain insight on when models are likely (or unlikely) to robustly transport to different settings. This project offers researchers, clinicians, and other stakeholders a comprehensive catalog of extant CPMs in CVD, including a description of how well each has been tested and how each has performed in external validation, both in published studies and in our fully independent evaluation. The long-term goal of this work is to contribute to a framework and facilitate a culture of independent and ongoing CPM evaluation and updating.
The specific aims of this project are as follows:
- Aim 1. Perform a comprehensive systematic review of validation studies of CPMs in the cardiovascular literature.
- Aim 2. Test a broad set of cardiovascular CPMs on a diverse range of data sets.
- Aim 3. Systematically apply updating and recalibration procedures for the improvement of model performance in new data sets.
Participation of Patients and Other Stakeholders
Our stakeholder panel included representatives from various fields with vested interest in CPM validation, including Gary Collins, PhD (researcher, research policy maker); William H. Crown, PhD (the “Big Data” industry); John K. Cuddeback, MD, PhD (informatics); Bray Patrick-Lake, MFS (patient advocate, clinical trial participant); Dana G. Safran, ScD (insurance markets); John A. Spertus, MD, MPH (industry and domain expert, editor); James E. Udelson, MD (clinician, researcher); and Qi Zhou, BM, MBA, MHCM (insurance markets).
Stakeholders were consulted during all phases of the project, including design, implementation, and interpretation of results. Stakeholders participated in 90-minute teleconferences held biannually (ie, every 6 months) throughout the grant period, during which they were informed of study progress and asked for input on completed work and recommendations for ongoing and future work.
Some study decisions were made specifically because of stakeholder engagement. One major example is the addition of the PROBAST to the project. At the July 2018 stakeholder meeting, the study team reviewed preliminary results on model performance, which generally were surprisingly poor. Discussion focused on various ways to parse out whether the poor performance of the CPMs when they were used with external data sets was due to model invalidity vs changes in case mix or to the clinical relatedness of the data sets. This led to 3 changes in the protocol: (1) the inclusion of a model-based C statistic42 to control for differences in case mix between data sets; (2) refinement of relatedness rubrics (discussed in the following paragraph); and (3) the application of PROBAST, an unpublished tool under development by an international team of methodologists. The changes in the protocol ultimately led us to develop a shortened version of the tool appropriate for large-scale evaluation and to incorporate it into study analyses.
Another example of the impact of stakeholder input was the development of the relatedness rubrics. In the original grant proposal, we outlined a generic relatedness system to assess the similarity of the clinical population and setting between the model development and validation environments that would apply to all validation exercises. When we reviewed this system with the stakeholders, the inadequacies of a generic approach became apparent. For instance, we presented calibration plots and NB curves for the validation of a CPM using the population from the Beta-Blocker Heart Attack Trial.43 This particular match led to a discussion of the nuances between patients with acute myocardial infarction (AMI), those who are post-MI (ie, had an MI within the last 30 days but are no longer in the acute phase), and those who had an MI long enough ago to be considered as having stable coronary artery disease (CAD). To address these and similar issues, we developed separate rubrics for each cardiovascular index condition (limited to the top 10 most common index conditions). This revised process required more thorough rubrics that could better categorize relatedness for any given validation exercise—albeit through a more laborious, painstaking, and less broadly generalizable process.
Finally, we also received valuable stakeholder input on website design and functionality, how to display relevant information for each validation exercise, and how to summarize performance results in a way that would be informative for both clinicians and researchers. Based on this feedback, we revised the format for displaying validation results on the website, pulled additional population information to put each validation in context, and performed several iterations of summary statistics until we found the most informative set.
Methods
Overview
For aim 1, we describe the extent and quality of validation and reporting practices of CPMs included in the Tufts PACE CPM Registry,10 a novel database that extensively characterizes cardiovascular CPMs published from 1990 to May 2015. Using standard measures of model performance, we also summarize information about the transportability and generalizability of CPMs and examine determinants of model performance. To make the results of this aim more accessible to researchers and clinicians, we report our findings in a searchable format as part of our online registry (pacecpmregistry.org).
The purpose of aim 2 was to better understand the broad trustworthiness of published models by performing fully independent external validation using patient-level data from publicly available clinical trial data sets, with a particular focus on calibration (which is otherwise underreported) and including new utility-based measures of validation that assess the NB of model application. After matching available CPMs from the Tufts PACE CPM Registry for a given clinical condition to publicly available patient-level trial data, we assessed model performance. To better understand the degree to which any decrease in discrimination might be due to model invalidity rather than to narrower case mix, we compared any changes in performance against a model-based C statistic.42 We also sought to understand whether model performance would vary based on the clinical relatedness of the derivation and validation populations and the ROB of the CPM. We focused on 3 index conditions: population-based models (ie, predicting the onset of cardiac disease), acute coronary syndromes (ACSs), and HF.
Anticipating that aim 2 might uncover surprisingly serious and widespread issues with calibration and substantial potential for serious harm, for aim 3, we explored the effects of statistical remedies. Whereas aim 1 and especially aim 2 were hypothesized to provide compelling evidence that application of CPMs might be more problematic than widely believed, aim 3 was designed to point the way forward and emphasize the importance of model updating on local populations (and on multiple populations) and understanding the context of decisions. Using the CPM-trial data set pairs described in aim 2, we assessed model performance after efforts at recalibration.
Research Design
Aim 1: Systematic Review of Published Validation Studies
Aim 1 is based on a systematic review of the published literature spanning 25 years (1990-2015) in which we sought to identify CPMs for CVD. External validations of these models were identified by searching a source-neutral abstract and citation data set. Model performance upon validation was described and predictors of poor performance were assessed.
Aim 2: Validation Testing Using External Data
CPMs identified in the Tufts PACE Registry were matched to publicly available clinical trial data sets and industry-sponsored trial data. The performance of the CPMs was characterized using measures of discrimination, calibration, and NB.
Aim 3: Evaluating Statistical Remedies to Poor Performance
Various recalibration techniques to update the model intercept or to rescale or reestimate the regression coefficients were applied to the models matched to data sets in aim 2 to assess the impact on discrimination, calibration, and NB.
Data Sources and Data Sets
Aim 1: Systematic Review of Published Validation Studies
Tufts PACE CPM Registry of CVD-related CPMs
The cardiovascular CPMs that form the basis of this review are found in the Tufts PACE CPM Registry. This registry is available at pacecpmregistry.org and represents a field synopsis of CPMs for patients at risk for and with known CVD. For this registry, a CPM is an equation that estimates an individual patient's absolute risk for a binary outcome. To create the registry, we searched PubMed for English-language articles containing CPMs for CVD that were published from January 1990 to March 2015. Briefly, for inclusion in the registry, an article must present the development of a cardiovascular CPM and contain a model predicting a binary clinical outcome, and the model must be presented in a sufficiently detailed way to allow prediction of outcome risk for a future patient. The search strategy and inclusion criteria have been previously reported10,11 and are available in Table 1 of Appendix A.
External validation search and data extraction
An external validation was defined as any report that evaluated a CPM for the same outcome in a data set distinct from the derivation data, including validations that were performed on a temporally or geographically distinct part of the same cohort (ie, nonrandom split sample), and reported at least 1 measure of model performance. To identify validations of the CPMs, we conducted a Scopus citation search of registry CPMs on March 22, 2017. Citations were reviewed by 2 members of the study team to identify external validations. Discrepancies were reviewed by a third member of the research team. We focused on validations of cardiovascular CPMs published from January 1990 to March 2015 predicting outcomes for the 10 most frequently studied index conditions: (1) ACS, (2) aortic disease, (3) arrhythmia, (4) HF, (5) cardiac surgery, (6) population sample (ie, populations at risk for incident CVD), (7) revascularization procedures, (8) stroke, (9) venous thromboembolism, and (10) valve disease.
Information about each CPM-validation pair was extracted, including sample size, continent on which the study was conducted, number of events, and reported measures of discrimination and calibration. CPM performance in the validation focused on whether CPM discrimination changed when compared with that seen in the derivation population. We also documented whether validations included any assessment of calibration. Given the lack of a literature standard for assessing calibration, any comparison of observed vs expected outcomes was considered to be an assessment of calibration, such as a Hosmer-Lemeshow statistic or calibration plot. We also included measures of global fit, where overall observed event rates are compared with predicted rates (ie, calibration-in-the-large).
Population relatedness
To assess the similarity between the derivation and validation populations, we created relatedness rubrics for each of the top 10 index conditions (rubrics are available in Appendix B Tables 1-10). These rubrics were created by investigators with clinical expertise in these areas (ie, senior cardiology fellows or attending cardiologists). Generally, the relatedness rubric fields comprised 5 domains: (1) recruitment setting (eg, emergency department vs inpatient), (2) major inclusion and exclusion criteria, (3) intervention type (eg, percutaneous coronary intervention vs thrombolysis for AMI), (4) therapeutic era, and (5) follow-up duration. Relatedness was assessed for each CPM-validation pair to divide validations into 3 categories: closely related, related, and distantly related. A fourth category—“no match”—was assigned to potential validations that were excluded from analysis because they were not clinically appropriate (eg, CPM was validated using data from a population with a nonoverlapping index condition or outcome). Pairs were categorized as closely related when they were tested on a nonrandomly selected sample of the derivation data set that was excluded from model development (ie, a geographically or temporally distinct sample), and as related when the data set was different but there were no clinically relevant differences in inclusion criteria, exclusion criteria, recruitment setting, and baseline clinical characteristics. Any matches with clinically relevant differences in these criteria were categorized as distantly related. Two clinicians independently reviewed these domains for each CPM-validation match and assigned them to 1 of the 3 categories. Nonrandom split-sample validations were labeled “closely related.” Discrepancies were reviewed by the study team to arrive at a consensus. Clinical experts scoring relatedness were blinded to the derivation C statistic of the CPM and outcome rates in the derivation and validation cohorts.
Assessing CPMs for ROB: short form
The comprehensivePROBAST37 evaluates the adherence of prediction studies to rigorous methodological practices and might provide useful information to identify models that are likely to perform poorly when externally validated. PROBAST was specifically developed for systematic reviews and was proposed to provide a comprehensive overview of the quality of model development. However, our early efforts to apply the tool—which requires both subject and methodological expertise—indicated that it might be too time consuming for large-scale use across hundreds of CPMs. The study team therefore endeavored to develop a shorter version of PROBAST to efficiently apply to the CPMs included in this project and to evaluate the use of this tool in identifying CPMs likely to perform poorly.
The short form was developed by selecting the subset of items from the original PROBAST felt to be most likely to influence model validity and bias, based on expert opinion. Based on the methodological expertise of investigators (including D.M.K., E.W.S., B.V.C., and D.V.K.), we discussed the relevance of all 20 items and rated them according to their potential effect on the ROB. We did not include items related to applicability, because this concern is addressed by our assessment of relatedness. Because we were aiming for a tool that can be applied by research assistants (ie, trained study staff without doctoral-level clinical or statistical expertise) within 15 minutes, we evaluated usability of the separate instrument items by testing them with 2 research assistants.
The following 6 items were considered most relevant to the ROB and easiest to assess, and were thus included in the short form: (1) outcome assessment, (2) outcome events per predictor variable (EPV), (3) continuous predictors, (4) missing data, (5) univariable predictor selection, and (6) correction for overfitting/optimism. We developed a guide using definitions from the original PROBAST article with additions to improve clarity and reduce ambiguity (see “Short-Form Guidelines” in Appendix C, Item 1). One point was assigned for each item that was incorrectly performed, resulting in a total score ranging from 0 to 6 points. Similar to the original PROBAST, CPMs receiving a total score of 0 were classified as “low ROB” and models with a score of ≥1 as “high ROB.” When the total score was 0 but there was insufficient information provided to assess all items, the model was rated “unclear ROB.” Because we assumed that the effect of using univariable selection and failing to correct for optimism would be negligible when the effective sample size was large, we did not assign points to these items when the EPV exceeded a certain threshold (defined as ≥25 outcomes for each candidate predictor or, when the number of candidate predictors was not reported, ≥50 outcomes for each predictor in the final model).
Before applying the shortened version of the PROBAST (here called the short form) to the full set of CPMs, we compared its performance with that of the full PROBAST using a subset of models. Two independent reviewers used the guidelines reported in the PROBAST explanation and elaboration article38 to apply the full PROBAST and the short form to all stroke models with both a reported derivation set area under the receiver operating characteristic curve (AUROC) and at least 1 external validation (n = 52), plus 50 randomly selected models among other index conditions, yielding a total subset of 102 models. The Cohen κ value was calculated to assess interrater reliability, and discrepancies were discussed with a third reviewer to arrive at a consensus. Based on these results, the reviewer guidelines for scoring the short form were further improved.
After agreement with the original PROBAST was confirmed, 3 research assistants applied the short form to all CPMs in the registry that had at least one 1 external validation (n = 556). Blinded double extractions were performed for a random sample (n = 31 models) as a quality check to compare assessments and discuss discrepancies. Because the short form was designed from a subset of the original PROBAST, CPMs identified as having a high ROB were anticipated to have perfect specificity (because high ROB CPMs on the short form would also be high ROB on the full PROBAST), but models classified as having low ROB might be reclassified as having high ROB when the full 20-item PROBAST was applied (ie, imperfect sensitivity). Thus, all models that were rated as having low or unclear ROB were reassessed by a separate reviewer using the full PROBAST to identify any potential items suggestive of high ROB.
Aims 2 and 3: Assessing CPM Performance Using External Data and the Effects of Different Recalibration and Updating Procedures
Validation data
Publicly available clinical trial data sets44-59 were accessed through the National Heart, Lung, and Blood Institute via the Biologic Specimen and Data Repository Information Coordinating Center (BioLINCC) and supplemented with industry-sponsored clinical trials60,61 (the list of trials is in Table 2; details are summarized in Appendix D, Table 1, Table 2, and Table 3). This list represents a convenience sample of publicly available trials on the selected index population that ascertained the relevant outcomes.
Table 2
Clinical Trial Data Sets Used to Externally Assess CPM Performance.
CPM-data set matching procedure
We used a hierarchical matching procedure to identify appropriate validation cohorts for a given CPM. First, each CPM was compared with each data set by nonclinical research staff to identify pairs that had grossly similar inclusion criteria and outcomes, which were then reviewed for appropriateness by clinical experts. Potential pairs that passed these screening steps were carefully reviewed at a granular level by statistical and clinical investigators. Only pairs for which sufficient patient-level data existed in the trial data set, such that the CPM could be used to generate a predicted outcome probability for each patient, were included in the analysis.
Relatedness
To explore sources of variability in external validation model performance, we categorized each CPM-data set pair based on the relatedness of the underlying study populations using the methods described previously. We assessed the pairs using the same method we used in aim 1 to evaluate the derivation and data set populations (the rubrics are available in Appendix E, Table 1, Table 2, and Table 3). There were no “closely related” designations because no data set served as the derivation populations for a CPM.
Analytical and Evaluative Approach
Aim 1: Systematic Review of Published Validation Studies
Percentage changes in CPM discrimination from derivation to validation were described on a scale of 0% (no change in discrimination) to −100% (complete loss of discrimination) because this more intuitively reflects the true changes in discriminatory power (Box 1).22 Positive changes represent improvements in discrimination. The percentage change in discrimination is calculated using Equation 1:
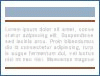
Box 1
Understanding Percentage Change in Discrimination (ΔAUROC) and the Difference in ΔAUROC.
where AUROC is numerically equivalent to the C statistic for binary outcomes, and 0.5 is a prediction with no discrimination.
We calculated the median and interquartile range (IQR) of the change in discrimination for low-ROB vs high-ROB models and for (closely) related vs distantly related models.
Factors associated with external validation
We examined a set of study-level factors for their association with whether external validation of a CPM was performed. These factors were identified based on observed methodological and reporting patterns, as well as previously studied predictors.62 These factors included the index clinical condition, internal validation performed, year of publication (divided here into the following categories: before 2004, 2004-2009, 2009-2012, after 2012), continent of origin, study design (eg, clinical trial vs medical record), sample size, number of events, number of predictors, prediction time horizon (<30 days, 30-365 days, >365 days), regression method (eg, logistic regression vs Cox regression), and reporting of discrimination or calibration. We looked at univariate associations and used generalized estimation equations (GEEs) to assess whether these variables were associated with CPM external validation.
Factors associated with poor performance on validation
A set of study-level factors defined a priori were evaluated for their association with changes in CPM performance (% change in discrimination) during validation. These factors included presence of overlapping authors (between model derivation and validation), validation performed in the same or a different article from the derivation, CPM modeling method (eg, logistic regression vs Cox regression), CPM data source (eg, clinical trial vs medical record), validation data source, large outcome rate discrepancy between derivation and validation data set (defined as >40%), and outcome EPV. To take into account the correlation between validations of the same CPM, we used GEEs to assess the effects of these variables on the observed change in discrimination. Multiple imputation using 20 imputation sets was used to accommodate missingness.
Analyzing the PROBAST and the short form
The Cohen κ statistic was calculated to assess interrater reliability and agreement between the PROBAST and the short form. We also used sensitivity and specificity for detecting studies with a high ROB, using the full PROBAST as the gold standard. As a measure of the observed bias in model performance in each derivation-validation pair, we used the percentage change in the C statistic between the derivation cohort and the validation cohort, as described previously. We calculated the median and IQR of the change in discrimination for low-ROB vs high-ROB models and for (closely) related vs distantly related models.
To consider the correlation between multiple validations of the same CPM and to control for potential confounders, such as the factors discussed in the previous section, we again used GEEs to assess the effect of ROB classification on the observed percentage change in discrimination. Multiple imputation using 20 imputation sets was used to accommodate missingness accounting for the uncertainty in the missing values under a missing-at-random assumption.
Aim 2: Assessing CPM Performance in External Study Cohorts
CPMs were evaluated on measures of discrimination, calibration, and NB when used to predict outcomes in external validation cohorts. We focused on CPMs for the following 3 index conditions: (1) population samples (ie, prediction of incident CVD), (2) HF, and (3) ACS. For each CPM-data set pair, the linear predictor was calculated using the predictor variable for each patient in the data set and the intercept and coefficients from the published CPM. Model discrimination was assessed using the C statistic. The percentage change in discrimination from the CPM to the validation data set was calculated using Equation 1 in aim 1.63 Change in discrimination was also compared using the model-based C statistic (MB-C).42 The MB-C is a measure of the C statistic that would be expected based solely on the distribution of model-derived predictions in a given population (a measure of case-mix heterogeneity), independent of the actual observed outcomes in the validation sample.42 Thus, any difference between the C statistic measured in the derivation cohort and the MB-C in the validation cohort reflects differences in case mix, whereas differences between the MB-C and the apparent C statistic in the validation cohort reflect model invalidity. Because calculation of the MB-C depends entirely on the validation cohort, MB-C could be calculated for all derivation-validation pairs.
Model calibration was assessed by converting the linear predictor (lp) to the estimated event probability (including a specified time point if Cox proportional hazards modeling was used) using the following equation: predicted value = (1 / [1 + e−lp]). Calibration-in-the-large as well as calibration slope (both defined in Table 1) and the Harrell E statistic for calibration error (EAVG), standardized to the outcome rate, were assessed. EAVG computes the average absolute calibration error (difference between the observed outcome rate and the estimated probabilities, where the observed rate is estimated using a nonparametric locally weighted scatterplot smoothing). The E90, representing the 90th percentile of the absolute calibration error, was also calculated.
Clinical utility was summarized by using DCA to estimate the NB28 (Box 2) of each model in each paired validation data set. DCA estimates the NB over a full range of decision thresholds, where decision threshold refers to the probability at which a clinical decision changes (eg, from “do not treat” to “treat”). DCA presents a comprehensive assessment of the potential population-level clinical consequences of using CPMs to inform treatment decisions by examining correct classification and misclassification of patients across the full range of thresholds, while weighting the relative utility of true-positive and false-positive predictions as implicitly determined by the threshold. Thus, NB integrates model performance (calibration and discrimination) with the relative utility weights of classification errors to provide a comprehensive assessment of the potential clinical consequences of using CPMs to inform treatment decisions.
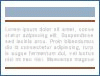
Box 2
Using DCA to Estimate NB.
We used standardized NB64 (ie, scaled to the incidence rates) to make results comparable across validations. Models were assessed for whether they resulted in an NB above or below the best default strategy (ie, treat all or treat none) at each of 3 arbitrary decision thresholds: (1) the outcome incidence, (3) half the outcome incidence, and (3) double the outcome incidence. (For example, if the average mortality rate in a sample is 10%, we report NB with decision thresholds at 5%, 10%, and 20%.) Models in which NB was equivalent to the best default strategy were considered neutral (no benefit or harm). Differences in various model performance measures (between related and distantly related validations) were assessed using the Wilcoxon rank-sum test.
Because the NB (a weighted sum of the benefits minus the harms, as described in Box 2) provides a summary measure of whether a CPM can improve decision-making at a given decision threshold, accounting for both discrimination and calibration, we used this measure to classify model evaluations into those that demonstrated the potential for harm at any 1 of the 3 thresholds of interest and nonharmful models (NB or neutral at all 3 thresholds). In an exploratory analysis, we examined the relationship between this binary outcome and calibration (using the standardized EAVG) and discrimination (ie, C statistic on validation), using plots and logistic regression.
Aim 3: Evaluating Statistical Remedies to Poor Performance: Recalibration and Model Updating
It is not uncommon for CPMs to be substantially miscalibrated in an external data set, particularly when the new data set has different outcome rates than the derivation data set, even while retaining reasonable discrimination. CPM recalibration adjusts model predictions to the outcome rates in new data sets, which is more statistically efficient than deriving an entirely new model. We performed recalibration or updating using 3 separate techniques. The first (most conservative) method addresses only calibration-in-the-large. In this approach, the difference between the mean observed outcome rates in the derivation and validation cohorts is used to update the intercept. The second technique corrects the intercept (as in the first method) and also applies a uniform correction factor to rescale the regression coefficients to better fit the validation cohort (ie, correction of the intercept and slope). This is done by running a regression on the new data set with the linear predictor (or logit) as the only variable in the model. The third approach reestimates the regression coefficients to better fit the validation data set but maintains the predictors from the original model.65 Although the first 2 approaches affect only calibration, reestimating coefficients will change the rank ordering of patients and therefore also improve discrimination.
All statistical analyses were performed in R version 3.5.3 (R Foundation for Statistical Computing).
Research Conduct
We received initial approval from the Tufts Health Sciences IRB on February 23, 2017. We submitted annual continuing reviews to update the IRB on study progress and maintain approval. A study protocol was posted to the International Prospective Register of Systematic Reviews (PROSPERO) in April 2017.66
One modification to the study protocol was the addition of an ROB assessment. During a stakeholder discussion, bias in model development arose as a potential explanation for poor model performance. Our investigator team was aware of a tool—PROBAST—that was being developed by another research team and designed to assess adherence to methods created to avoid bias during model development. Although the tool had not yet been published, we reached out to the investigators (Drs Robert Wolff and Carl Moons), who generously shared a prepublication version of the instrument. We found that the tool required a fair amount of expertise (both clinical and methodological) to apply and also was time intensive (requiring ~45 minutes to 1 hour to evaluate each CPM). Thus, it was not suitable for a large-scale evaluation of CPMs. We endeavored to create a short-form version of the full PROBAST, which would (1) shorten the time required for each model, (2) allow research assistants to apply the instrument, and (3) facilitate quantitative analysis by providing a simplified score. After evaluation of the short form by comparing results with the original PROBAST on 102 CPMs, we applied the short form to all CPMs in the registry that were externally validated so that we could examine the relationship (if any) between ROB as assessed by the instrument and model performance on external validation.
Another change in study procedures was the modification of the relatedness rubrics (also described in the Patient and Stakeholder Engagement section). In the original grant submission approved by PCORI, we proposed a general framework for quantifying the similarity between the derivation and validation populations for any given validation exercise. Based on input from the stakeholders, we revised this system so that each index condition had its own relatedness rubric.
Results
Aim 1: Systematic Review of Published Validation Studies
Overview of Validations
The Tufts PACE CPM Registry includes 1382 unique CPMs for CVD, and the citation search identified 2030 external validations that were extracted from 413 papers (Table 3 and Table 4). The results of this systematic review have been made publicly available on the registry website. Only 575 of the CPMs (42%) in the full registry have ever been validated, which was defined as having at least 1 published external validation study (Table 4). On average, there were 1.5 validations per CPM (range, 0-94), but the distribution across models was very skewed. The Logistic EuroSCORE67 has been externally validated 94 times, whereas 807 of the CPMs (58%) have never been externally validated. For this analysis, we have included a subset of the full registry—1846 validations of 556 CPMs—after the exclusion of 19 decision trees and CPMs tested only on inappropriate populations (ie, a mismatch index condition). The median external validation sample size was 861 (IQR, 326-3306), and the median number of events was 68 (IQR, 29-192) (Table 4).
Table 3
Study and Model Characteristics of CPMs in the Tufts PACE CPM Registry.
Table 4
Characteristics of External Validation Exercises of CPMs.
CPM Validation Discrimination
Overall, 91.3% (n = 1685) of the external validations report area under the receiver operating characteristic curve (AUROC, or equivalently, the C statistic). The median external validation AUROC was 0.73 (IQR, 0.66-0.794), representing a median percentage change in discrimination of −11.1% in the validation cohort (IQR, −32.4% to 2.7%) (Table 4). Two percent (n = 35) had a >80% drop in discrimination, whereas 19% (n = 352) of model validations showed CPM discrimination at or above the performance reported in the derivation data set.
CPM Calibration
In total, 53% (n = 983) of the validations reported some measure of CPM calibration in the external data (validation sets). The Hosmer-Lemeshow goodness-of-fit test was most commonly reported (30% of validations; n = 555), followed by calibration-in-the-large (26% of validations; n = 488), and calibration plots (22% of validations; n = 399) (Table 4). Overall, including those CPMs that have not been validated at all, there is no information available on calibration in an external data set for 86% (n = 1182) of the CPMs in the registry.
Clinical Domains
The 10 cardiovascular conditions with the most CPM validations are listed in Table 5. The condition with the largest number of validated CPMs was stroke (n = 104 CPMs). The condition with the highest proportion of validated CPMs was arrhythmia (81%; 17 CPMs). There were 295 validations of CPMs for populations at risk of developing CVD (population samples). Although the change in discrimination varied widely, there was generally a large loss of discriminatory performance, overall, during external validations across all conditions studied (Table 5).
Table 5
Characteristics and Relatedness of External Validations by Index Condition.
Range of Performance for Individual CPMs
There was substantial range in performance of CPMs when evaluated in >1 validation data set. Discrimination for the Logistic EuroScore67 (validated 94 times) ranged from 0.48 to 0.90 across different data sets. Indeed, the 10 models that have been validated most frequently, with or without reported AUROC, were all validated >20 times, and virtually all showed a range of discriminatory performance from near useless to excellent (Table 6). The variation likely relates to differences in the clinical relatedness of the derivation and validation populations, and changes in the case mix across different validation populations, as well as other unknown factors (including the role of chance). This indicates that a single external CPM validation is unlikely to broadly reflect how well a model is likely to perform in other data sets.
Table 6
Characteristics of the Top 10 Most Validated CPMs.
Relatedness
Population relatedness was assigned to derivation or validation pairs for the top 10 index conditions (n = 1877). Of those, validation studies were excluded from our analysis when there was no overlap in index condition (n = 156) and when nonregression methods were used (eg, decision trees; n = 19), leaving 1702 validations with a relatedness determination. Of these, 123 (7%) of the validations were performed on closely related populations, 862 (51%) were performed on related populations, and 717 (42%) were performed on distantly related populations. The median AUROC was 0.78 (IQR, 0.719-0.841) for closely related validations, 0.75 (IQR, 0.68-0.803) for related validations, and 0.70 (IQR, 0.64-0.77) for distantly related validations (P < .001). Overall, the percentage change in discrimination from derivation to validation sample was −3.7% (IQR, −13.2 to 3.1) for closely related validations, −9.0 (IQR −27.6 to 3.9) for related validations, and −17.2% (−42.3 to 0) for distantly related validations (P < .001) (Table 4).
Studying Predictors of External Validation
Study features that are associated with CPM external validation are listed in Table 7. The index condition was strongly associated with subsequent external validation. Models that were internally validated and models that were published more recently were less likely to be externally validated. Sample size, number of predictors, and reporting of discrimination or calibration were associated with subsequent external validation. On multivariate analysis, these predictors remained associated with CPM external validation. Study design, prediction time horizon, and regression method were not associated with a model being externally validated.
Table 7
Predictors of External Validation: Analysis of Maximum Likelihood Parameter Estimates (n = 1382).
Studying Predictors of Poor Model Performance
Predictors of CPM validation performance are reported in Table 8. On univariate analysis, population relatedness was significantly associated with CPM discrimination in validations. When CPMs were tested on distantly related cohorts, the AUROC decrease from derivation to validation set was −15.6% (95% CI, −22.0 to −9.1) greater than validations performed on “closely related” cohorts. When evaluated in a multivariate model, population relatedness remained significantly associated with CPM discrimination in validations. Validations had AUROCs that were 9.8% (95% CI, 5.4-14.2) higher when reported in the same manuscript (with the same authors) as the original CPM report, compared with validations reported in different manuscripts with nonoverlapping authors, although this effect was attenuated (and no longer statistically significant) in the multivariable analysis.
Table 8
Univariable and Multivariable Predictors of CPM Validation Performance.
ROB Assessments and Influence on Model Performance
First, the full PROBAST was assessed on the initial set of 102 models (n = 52 stroke models; n = 50 other index conditions). Of these models, 98 (96%) were rated as having high ROB and only 4 (3.9%) were rated as having low ROB. Overall, high ROB was mainly caused by high ROB in the analysis domain, whereas the other 3 domains seemed to contribute little information (Figure 1A). Agreement between the 2 reviewers, before the final consensus meeting, was 96% for the overall judgment (κ = 0.49). Interrater agreement ranged between 62% and 100% per item (κ = 0.05-0.96). When applying the short-form version of the PROBAST to the same 102 models, we found that the sensitivity to detect high ROB was 98% (using the full PROBAST as the gold standard) and specificity was 100%. Overall agreement was very good (98%; κ = 0.79). The outcome assessment was rated as having high ROB in only 4% of the models; the percentage of high ROB of the other items ranged between 39% and 77% (Figure 1B). Figure 2 shows the distribution of the short-form total scores.

Figure 1
Summary of ROB Assessment Using (A) PROBAST and (B) Short Form.

Figure 2
Distribution of Short-Form Total Scores (n = 102 CPMs).
After making final adjustments to the PROBAST guidelines, we applied the short form to all CPMs in the database (n = 556) that had been externally validated at least once, with a total of 1846 validations (Table 9). In total, 529 (95%) were considered as having high ROB, 20 (3.6%) as having low ROB, and 7 (1.3%) as having unclear ROB. Only 1 model with unclear ROB was reclassified to high ROB after full PROBAST assessment of all low and unclear ROB models. Because the specificity for high ROB of the short form was 100%, this approach is expected to result in identical classification to using the full PROBAST for all models. Information on both the derivation AUROC and validation AUROC was available for 1147 validations (62%). The median change in discrimination of these derivation-validation pairs was −11% (IQR, −32% to 2.6%). The difference was much smaller in low-ROB models (−0.9%; IQR, −6.2% to 4.2%) than in high-ROB models (−12%; IQR, −33% to 2.6%) (Table 9).
Table 9
Change in Discrimination Between Derivation AUROC and Calibration AUROC by Short-Form ROB Score.
Similar ROB effects were seen in both related (combined closely and related) and distantly related CPMs (Table 9). The multivariable GEE model (Table 10) showed a difference in ΔAUROC of 17% (95% CI, 6.6% to 28%; P = .002) for low ROB vs high ROB, after controlling for clustering and confounding variables.
Table 10
Crude and Adjusted Associations Between ROB (as Assessed by Short Form) and Difference in ΔAUROC (n = 1048 Validations).
Tufts PACE CPM Registry: A Searchable Online Resource on Models and Their Validations
The Tufts PACE CPM Registry is a publicly available online resource that has additional information on external validations, including sample size, AUROC, whether calibration was reported, and relatedness of the validation population (pacecpmregistry.org). The registry can be searched by model index condition, outcome, or covariate, as well as publication author, year, and journal.
Aim 2: Assessing CPM Performance in External Data
To evaluate CPM performance using measures beyond what is typically reported in the literature, we tested selected CPMs on publicly available data sets. Of 674 total CPMs across the 3 index conditions (ACS, HF, and population samples), we were able to match 108 CPMs to 17 publicly available clinical data sets for 158 fully independent validations and for model recalibration and updating. Below, we report the results stratified by index condition.
Acute Coronary Syndrome
Patient-level data from 5 large randomized trials (namely, Aspirin Myocardial Infarction Study [AMIS],50 Thrombolysis in Myocardial Infarction [TIMI]-II,59 TIMI-III,52 Magnesium in Coronaries [MAGIC],53 and Enhancing Recovery in Coronary Heart Disease Patients [ENRICHD]54) were identified and used as validation data sets. Of 269 ACS CPMs screened from the CPM registry, 23 (8.5%) were compatible with at least 1 of the trials at the granular level (ie, CPM-required variables were reliably collected in the data set). Eighteen CPMs matched to a single data set, and 5 CPMs matched to 2 data sets. Thus, in total, 28 clinically appropriate external validations were performed (Figure 3).

Figure 3
Selection of ACS Models for External Validation in Trial Data Sets.
Discrimination
The median C statistic of the CPMs in the derivation cohorts was 0.76 (IQR, 0.74-0.78), and in the external validations it was 0.70 (IQR, 0.66-0.71), reflecting a 24% decrement in discrimination (Table 11). Validations using data from related populations showed a median 19% (IQR −27% to −9%) loss in discriminatory ability, and those using data from distantly related populations showed a median 29% (IQR, −44% to −15%) loss in performance. However, the MB-C was 0.71 (IQR, 0.66-0.75), which was very similar to the measured C statistic for the validation data sets (Figure 4), indicating that the narrower case mix of the validation population accounted for most of the decrement. Models had moderately better-preserved discrimination when tested on related (vs distantly related) data sets (Figure 4).
Table 11
Discrimination, Calibration, and NB of ACS Models on External Validation in Trial Data Sets (n = 28).

Figure 4
Percentage Change in Validation C Statistic Performance vs MB-C for ACS Models.
Calibration
The median calibration slope in external validations was 0.84 (IQR, 0.72-0.98), which indicates some overfitting (ie, overestimated risk predictions for patients with high risk of an outcome and underestimated risk predictions for patients with low risk). The median EAVG (standardized to the outcome rate) was 0.4 (IQR, 0.3-0.8)—that is, the average error was 40% of the observed outcome rate—and the standardized E90 was 1.0 (IQR, 0.5-1.4), indicating that 90% of individuals had prediction errors less than the average risk of the outcome. For validations using data from related populations, the median calibration slope was 0.9 (IQR, 0.79-1.05) compared with 0.83 (IQR, 0.65-0.93) for distantly related matches. (Calibration slopes <1.0 [ie, perfect calibration] represent a degree of model overfitness.) For related matches, the EAVG standardized to the outcome rate was 0.3 (IQR, 0.2-0.4), and the standardized E90 was 0.6 (IQR, 0.5-1.0). For distantly related validations, the EAVG standardized to the outcome rate was 0.7 (IQR, 0.4-0.8), and the standardized E90 was 1.2 (IQR, 1.0-1.7). (Appendix F, Table 1). Thus, calibration was relatively poor generally, and particularly for CPMs tested on distantly related data sets.
Net benefit
At a decision threshold set at the outcome rate (ie, at the incidence of the outcome), 100% of the CPMs were beneficial compared with the default strategies, because only a small deviation from the population average outcome should change the decision. However, at a threshold set to half the observed outcome rate, 32% of CPMs were harmful, 50% were beneficial, and 18% yielded outcomes similar to the default strategy of “treat all” (Table 12). With the threshold set to twice the outcome rate, 25% of CPMs were harmful, 54% were beneficial, and 21% would result in outcomes similar to the default “treat no one” strategy. CPMs tested on distantly related populations were more likely to be harmful than related validations at thresholds set at half (40% vs 23%) or twice (40% vs 8%) the outcome rate.
Table 12
NB of ACS Models Compared With Default Strategy According to 3 Decision Thresholds (n = 28).
Heart Failure
CPM–data set matches
A total of 1080 potential HF CPM–data set matches that included 135 individual CPMs were identified. After the exclusion of pairs with no match on population, predictor variables, or outcomes, 42 clinically appropriate CPM–data set matches remained (Figure 5). These 42 CPM–data set matches included 24 unique CPMs: 14 CPMs derived from a population admitted to the hospital with acute decompensated HF and 10 CPMs derived from outpatients with chronic HF.

Figure 5
Selection of Acute and Chronic HF Models for External Validation in Trial Data Sets.
Discrimination
Fourteen acute HF CPMs were matched with 1 validation data set (Efficacy of Vasopressin Antagonism in Heart Failure Outcome Study With Tolvaptan [EVEREST]61). Derivation AUROC was reported in 10 acute HF CPMs (71%), ranging from 0.69 to 0.86 (median, 0.76; IQR, 0.75-0.8) (Table 13). For these 10 acute HF CPMs, the median percentage decrement in discrimination was −40% (IQR, −49% to −19%). For most models, the decrement in discrimination was largely due to less case-mix heterogeneity in the EVEREST cohort (the median percentage change between derivation and MB-C was −24% [IQR, −33% to −5%]) and, to a lesser extent, to model validity (the median percentage decrement between the MB-C and the validation cohort was −8% [IQR, −31% to 5%]). In Figure 6, the percentage change in the validation C statistic vs the MB-C is shown for HF models in general (ie, both acute and chronic), indicating that the decrement in discrimination was more pronounced in the distantly related models than in the related models. CPMs developed in smaller cohorts tended to have the greatest decrement in discrimination between both derivation and validation, as well as between validation and MB-C, suggesting that the decrement was mostly due to model invalidity (perhaps due to too few outcomes per predictor) in the external population and not the case mix.
Table 13
Discrimination, Calibration, and NB of Acute HF Models (n = 14) on External Validation in Trial Data Sets.

Figure 6
Percentage Change in Validation C Statistic Performance vs MB-C for Acute and Chronic HF Models.
Calibration
The median calibration slope for the 14 acute HF CPM models was 0.87 (IQR, 0.57-1.0), the median EAVG standardized to the outcome rate was 0.5 (IQR, 0.4-2.2), and the median standardized E90 was 1.1 (IQR, 0.5-3.4) (Table 13). Calibration was worse for the 4 models predicting in-hospital mortality, and better for models predicting outcomes over a longer time frame (3-18 months).
Net benefit
In DCA, using a decision threshold set to the outcome rate, 29% of acute HF CPMs were harmful and 71% were beneficial. At a threshold of half the outcome rate, 29% of acute HF CPMs were harmful, 14% were beneficial, and 57% were neutral. At a threshold of twice the outcome rate, 57% were harmful and 29% were beneficial (Table 14).
Table 14
NB of Acute HF Models (n = 14) Compared With Default Strategy at 3 Decision Thresholds.
Ten chronic HF CPMs were matched to 7 validation data sets (Treatment of Preserved Cardiac Function Heart Failure with an Aldosterone Antagonist, [TOPCAT]44 Heart Failure Endpoint Evaluation of Angiotensin II Antagonist Losartan [HEAAL],60 Heart Failure: A Controlled Trial Investigating Outcomes of Exercise Training, [HF-ACTION]45 Sudden Cardiac Death in Heart Failure Trial [SCD-HeFT],46 Beta-Blocker Evaluation of Survival Trial Investigators [BEST],47 Digitalis Investigation Group [DIG],48 and Studies of Left Ventricular Dysfunction [SOLVD]49), for a total of 28 validation studies.
Discrimination
Derivation AUROC was reported in 8 chronic HF CPMs (80%) and ranged from 0.73 to 0.81 (median, 0.76; IQR, 0.74-0.8) (Table 15). Changes in discrimination for HF models in general (ie, both acute and chronic) are shown in Figure 6. In those 8 chronic HF CPMs, the median percentage decrement between the derivation and validation cohort AUROCs was −55% (IQR, −62% to −48%). Unlike the acute HF CPMs, for most chronic HF CPMs, the decrement in discrimination appeared to be due to both case-mix heterogeneity and model invalidity.
Table 15
Discrimination, Calibration, and NB of Chronic HF Models (n = 28) on External Validation in Trial Data Sets.
Calibration (see Table 1 for various measures)
The median calibration slope for the 10 chronic HF CPMs was 0.46 (IQR, 0.33-0.58), the median EAVG standardized to the outcome rate was 0.5 (IQR, 0.3-0.7), and the median standardized E90 was 0.7 (IQR, 0.5-1.0) (Table 15). Five of 28 chronic HF CPM–data set matches were related and the rest were distantly related. In the CPMs with related and distantly related matches, there was no signal for better model performance in related CPM-data set matches. In CPMs with multiple data set matches, there was a range of discrimination and calibration results, and no CPMs had consistently good discrimination and calibration across validation samples.
Net benefit
In DCA, at a decision threshold set to the outcome rate, 4% of chronic HF CPMs were harmful and 86% were beneficial. At a threshold of half the outcome rate, 64% of chronic HF CPMs were harmful, 7% were beneficial, and 29% were neutral. At a threshold of twice the outcome rate, 43% were harmful, 14% were beneficial, and 43% were neutral (Table 16).
Table 16
NB of Chronic HF Models (n = 28) Compared With Default Strategy at 3 Decision Thresholds.
Population Sample for Predicting Incident CVD
From a set of 195 potential CPMs in the registry, 157 (80.5%) were screened as potential matches to 4 publicly available clinical trial data sets (Action to Control Cardiovascular Risk in Diabetes [ACCORD],55 Antihypertensive and Lipid-Lowering Treatment to Prevent Heart Attack Trial [ALLHAT-HTN]56, Antihypertensive and Lipid-Lowering Treatment to Prevent Heart Attack Trial–Lipid-Lowering Therapy [ALLHAT-LLT]57, and Women's Health Initiative [WHI]58), including widely used scores such as the Framingham Risk Score69 and the Atherosclerotic Vascular Disease PCE.23 After screening, 63 unique CPMs (32%) were matched to at least 1 data set, yielding a total of 88 CPM-data set pairs (Figure 7), 55 (63%) of which included a derivation AUROC, and 62 (70%) of which had similar time horizons to be evaluable for calibration and NB.

Figure 7
Selection of Population Sample Models for External Validation in Trial Data Sets.
Discrimination
Among these 55 pairs, the median derivation AUROC was 0.77 (IQR, 0.72-0.78), and the median validation AUROC was 0.63 (IQR, 0.58-0.66). Discrimination decreased by a median of 60% (IQR, 42%-73%). Approximately half the loss in discriminatory power was attributable to a decrease in case-mix heterogeneity, and half was attributable to model invalidity (Table 17).
Table 17
Discrimination, Calibration, and NB of Population Sample Models on External Validation in Trial Data Sets (n = 88).
When stratified by relatedness, 37 pairs (42%) were categorized as related and 51 (58%) were categorized as distantly related. CPM-data set pairs that were related (eg, the Framingham Risk Score was “related” to the WHI) had a significantly higher MB-C and validation AUROC than pairs that were distantly related. The median percentage decrement in discrimination among related pairs was 42% (IQR, 23%-46%), of which approximately two-thirds was due to a decrease in case-mix heterogeneity and one-third was due to model invalidity. In contrast, CPM-trial pairs that were distantly related had a median percentage decrement in discrimination of 67% (IQR, 60%-80%; P < .001 vs related pairs), approximately half of which was due to case-mix heterogeneity and half due to model invalidity (Figure 8).

Figure 8
Median Percentage Change in C Statistic in External Validation Cohorts.
Calibration
The median calibration slope in external validations was 0.62 (IQR, 0.50-0.77). The median calibration slope among related pairs was 0.69 (IQR, 0.59-0.84), and the median calibration slope among distantly related pairs was 0.58 (IQR, 0.43-0.63; P < 0.001 vs related pairs), indicating substantial overfitting. The median EAVG and median E90, standardized to the outcome incidence among all pairs, were 0.60 (IQR, 0.40-0.70) and 1.0 (IQR, 0.7-1.2), respectively, and did not differ significantly between related and distantly related pairs (Table 17).
Net benefit
Recall that NB can be calculated based on selecting a decision threshold, which determines the sensitivity and specificity of the prediction and also reveals the implicit relative utility weights of TP and FP predictions. Specifically, (as described in Box 2) NB is equal to the number of decisions based on a TP prediction (the benefit of using the model) minus the decisions made based on an FP prediction (the harm of using the model), where the relative weight of harms to benefits is calculated also by the decision-threshold probability (pt) [(ie, NB = (TP – w FP) / N, where w is (pt / (1-pt)]. At a threshold equal to the outcome incidence, 51 of 62 tested CPMs (82%) resulted in increased NB compared with the best average default strategy; 5 of 62 (8%) resulted in decreased NB. At a decision threshold of half the outcome incidence, 41 of 62 evaluable CPM-data set pairs (66%) resulted in NB below the default strategy of treating all patients when applied to external validation cohorts (ie, the population-level impact of using the CPM to target treatment according to predicted risk was less favorable than that of simply treating all patients), whereas only 20 (32%) were beneficial relative to the default strategy (Table 18). At a threshold of twice the outcome rate, 31 tested CPMs (50%) resulted in NB below the default strategy of treating no patients, and only 12 (19%) were beneficial relative to the default strategy.
Table 18
NB of Population Sample Models (n = 62) Compared With Default Strategy at 3 Decision Thresholds.
Aggregated Summary Comparing CPM Performance on Related vs Distantly Related External Data Sets
Measures of change in discrimination, calibration, and NB are shown in Appendix F, Table 1. The median percentage decrease in the C statistic among 57 related validation data sets was 30% (IQR, −45% to −16%) and, among 101 distantly related data sets, the decrease was 55% (IQR, −68% to −40%; P < .001). The decrease was due both to a narrower case mix and to model invalidity: compared with the MB-C, the median decrease in the validation C statistic was 11% (IQR, −25% to 0%) in related data sets and 33% (IQR, −50% to −13%) in distantly related data sets. The median standardized EAVG was 0.5 (IQR, 0.4-0.7), indicating an average error that is half the average risk, and was not different based on relatedness. NB also did not differ significantly between related and unrelated data sets.
Aim 3: Statistical Remedies: Effects of Updating
Acute Coronary Syndrome
The median EAVG improved from 0.4 to 0.16 (an improvement of 60%; IQR, 36%-85%) with updating of the intercept alone. Calibration error further improved to 0.10 (an improvement of 76%; IQR, 40%-94% over baseline) with updating of the intercept and slope, and 0.06 (an improvement of 85%; IQR, 68%-91%) with reestimation of the regression coefficients (Table 19). NB generally improved with CPM updating. Updating the intercept alone was sufficient to protect against harm for all but 1 or 2 models at the selection thresholds. After updating the intercept and slope, we found no evidence of harm compared with the default strategies at thresholds at the outcome rate or at half the outcome rate, and only 1 model demonstrated harm with a threshold at double the outcome rate. No CPMs were harmful at any of the threshold values (half the outcome rate, outcome rate, double the outcome rate) after reestimation of the model regression coefficients (Table 12).
Table 19
Validation Performance of ACS Models After Updating (n = 28).
Heart Failure
For acute HF CPMs, most of the calibration error was corrected by updating the model intercept (median % change in E, −81%; IQR, −93% to −19%), with slight incremental improvement in calibration with updating both the intercept in the slope (incremental median % change in E, −22%; IQR, −73 to 0) and from reestimating the model (incremental median % change in E, −13%; IQR, −79% to 50%) (Table 20). The NB of using a CPM improved with updating the model intercept alone: 100% of CPMs were beneficial at the outcome rate, and a smaller percentage of CPMs were harmful at the other thresholds (Table 14 and Table 20).
Table 20
Validation Performance of Acute HF Models After Updating (n = 14).
For chronic HF models (unlike with the acute HF CPMs), updating the intercept only slightly improved calibration (median % change in EAVG, −23%; IQR, −54% to 0%), but updating both the intercept and the slope led to incremental improvement in calibration (incremental median % change in EAVG, −94%; IQR, −99% to −80%) (Table 21). There was marginal improvement in calibration from reestimating the model (incremental median % change in EAVG, 0%; IQR, −50% to 0). The NB of using a CPM improved with model updating, but updates to both the intercept and slope were required to substantially decrease net harm at half the outcome rate and twice the outcome rate (Table 16 and Table 21).
Table 21
Validation Performance of Chronic HF Models After Updating (n = 28).
Population Sample
The EAVG improved by a median of 57% (IQR, 43%-78%) across all CPM-trial pairs with updating of the intercept and by a median of 97% (IQR, 88%-100%) after updating the intercept and slope (Table 22). Similar results were seen for E90. No further improvement in calibration error was seen with reestimation. Although updating the intercept alone eliminated the likelihood of harm relative to the default strategy at a decision threshold equivalent to the outcome incidence, this did little to reduce the likelihood of harm at more extreme decision thresholds (Table 18). At decision thresholds of half or twice the outcome incidence, likelihood of harm remained >20%, even after complete reestimation of the model coefficients using patient-level data from the clinical trial populations.
Table 22
Validation Performance of Population Sample Models After Updating (n = 88).
Exploratory analysis: association of discrimination and calibration with NB
Of the 132 unique validations (of 89 unique CPMs) for which we were able to compute calibration, discrimination, and NB metrics, 110 validations (83%) showed the potential for harm at ≥1 treatment thresholds and 22 (17%) were nonharmful at all 3 thresholds. A scatterplot showing the relationship between potentially harmful vs nonharmful models as a function of calibration and discrimination is shown in Figure 9. Logistic regression demonstrated strong independent predictive effects of the validation C statistic (P = .01) and the validation standardized EAVG (P = .002). Among validations with a C statistic ≥0.7, 50% were nonharmful across all 3 thresholds, compared with just 9% of validations with C statistics <0.7 (n = 26). Among validations with a standardized EAVG <0.3 (n = 21), 52% were not harmful compared with just 10% when the standardized EAVG was ≥0.3 (n = 111). Only 8 CPM validations in this sample had both a C statistic >0.7 and a standardized EAVG >0.3; 7 (88%) were nonharmful.

Figure 9
Potentially Harmful vs Nonharmful CPMs as a Function of Calibration Error and Discrimination.
Updating procedures substantially increased the proportion of nonharmful CPMs: when the intercept alone was updated, 53 CPMs (40%) showed nonharmful NB results across all 3 thresholds. When both the intercept and slope were updated, 87 CPMs (66%) showed nonharmful NB results. This was not further improved by refitting model coefficients.
Discussion
Key Findings
Our Tufts PACE CPM Registry documents the tremendous growth in the number of CPMs being developed and published, very frequently without regard to previously published CPMs for the same index condition and outcome. Approximately 60% of published CPMs, however, have never been validated. Approximately half of those CPMs that have been validated have been validated only once. A small minority of models have been validated numerous times. The value of these single validations is unclear, because good (or poor) performance on a single validation does not appear to reliably forecast performance on subsequent validations. For example, the 10 most validated CPMs have each been validated >20 times; all show substantial variation in discrimination across these validation studies, from virtually useless (ie, C statistic = ~0.5) to very good (C statistic ≥~0.8). This demonstrates the difficulty of defining the quality of a model generically, because performance greatly depends on characteristics of the data set on which a model is tested.
Although there have been recent efforts to disseminate consensus guidelines to improve the methodological rigor of model development and model reporting,12,32-37 the vast majority (95%) of models in our database that have been validated did not adhere to good reporting or methodological practices as described in the PROBAST instrument. We developed a shortened version of the instrument that achieved near-perfect classification of CPMs into high vs low ROB (as measured by agreement with full PROBAST). This classification was predictive of the change in discrimination in an external data set, with the very few models classified as low ROB having discriminatory performance on external validation that was very similar to that measured in the derivation data set, whereas high-ROB CPMs showed substantial declines in discrimination. This is the first demonstration that PROBAST-based classification has a measurable impact on model performance.
The other main predictor of the change in discriminatory performance was the clinical relatedness of the validation data set to the derivation data set. However, judging the relatedness of the populations is laborious and requires substantial clinical expertise. Differences that may appear subtle can be very influential. For example, a CPM developed to predict 30-day mortality for patients with ACS might not be expected to validate well if it was developed from data on patients with ACS in the emergency department but is tested instead on similar patients admitted to the hospital (because a large proportion of the mortality outcome occurs in the first 24 to 48 hours). Changes in treatments received (eg, different ACS revascularization approaches,70 stent types71, or outcome definitions72,73) likely affect model validation performance. If the model was derived from data on patients receiving lytic therapy and validated using data from a more contemporary percutaneous coronary intervention trial, it should not be surprising that model performance appears worse than expected. For example, at first glance it would seem reasonable (and appropriate) to evaluate the performance of a model predicting outcomes for patients with AMI on a nonoverlapping group of patients with MI. However, when the model74 (derived from data on patients from the Global Utilization of Streptokinase and TPA for Occluded Coronary Arteries [GUSTO-I] trial in which all patients received lytic therapy) was evaluated using data from the ENRICHD randomized trial54 (in which patients could be enrolled after discharge from the hospital and fewer than half received lytic therapy), the AUROC decreased by 24%. Many other study-level characteristics examined did not greatly influence model performance. However, our analysis does show a positive effect on model performance when CPMs were tested by investigators involved in model development, suggesting that bias in measurement may seep into model evaluation when the evaluators have a reputational stake in model performance.18
A major limitation of our literature review (aim 1) is that model performance is not generally presented in a way that makes it clear whether a given CPM is likely to improve or worsen decision-making. Our main metric for model performance on external validation was the decrement in discrimination. This was selected because it is the most commonly reported measure and is typically presented as a quantitative result—not because it is the most important or clinically relevant measure of model performance. The clinical significance of change in discrimination is highly dependent on other factors, including the discrimination in the original derivation cohort, calibration (which is frequently not reported), and the clinical context. More recently, measures of NB (as described in Box 2), which provide more comprehensive evaluation of the benefits and harms of CPM use, have been proposed, but these are rarely reported.
These limitations in part motivated our aims 2 and 3; we performed 158 unique validations of 108 unique models focusing on 3 index conditions, using our own standardized evaluation framework to produce a more complete and granular evaluation than can be gleaned from a literature review. The major finding of these independent evaluations is that applying “off-the-shelf” CPMs to new populations very frequently results in potential for net harm. Indeed, only 22 of 132 (17%) of the unique evaluations we performed were either beneficial or neutral at all 3 decision thresholds examined. In contrast to what is often assumed, use of an explicit data-driven CPM is often not likely to be “better than nothing.”
The risk of harm of using CPMs in clinical practice is most salient when decision thresholds depart substantially from the average risk in the patient population of interest. For example, risk of harm would be substantial when trying to deselect a very-low-risk population for a test or treatment that is clearly beneficial on average, or when trying to select a very-high-risk population for a test or treatment that is clearly not indicated for those at average risk. However, when the point of indifference lies closer to the average risk, CPMs appear to be more likely to yield net clinical benefit and to be tolerant of some miscalibration. These findings were consistent across the 3 index conditions we tested.
That the decision threshold emerged as an important determinant of the utility of applying the CPMs in this sample emphasizes the importance of selecting the right decision context for CPM application—an often-neglected issue. Based on our results, CPMs yielding typical (ie, non-excellent) performance should generally be reserved for applications where the decision threshold is near the population average risk, particularly when model updating is not feasible (as it often is not). Intuitively, the value of risk information is highest when the decision threshold is near the average risk, because even relatively small shifts from the average risk due to using a CPM can reclassify patients into more appropriate decisions.
From our literature review (aim 1), we were unable to examine calibration because it is frequently unreported and, when reported, the metrics used vary from study to study and are largely uninformative regarding the magnitude of miscalibration (eg, Hosmer-Lemeshow). The validations (aim 2) we performed ourselves revealed that CPM-predicted outcome rates frequently deviated from observed outcome rates even when discrimination was good. The typical standardized EAVG was 0.5 (IQR, 0.4-0.7), which means that the absolute error is half the average risk. In exploratory analysis, we found that when the standardized EAVG was >0.3 (the average prediction was off by at least 30%), most models yielded harmful decisions at least at 1 threshold within the range examined (half the outcome rate to 2-fold the outcome rate). Similarly, it was unusual to find models that were consistently nonharmful at all examined thresholds when the validation C statistic dropped below 0.7.
We found that the risk of harm can be substantially mitigated, often simply by adjusting the intercept alone. Indeed, updating the intercept alone resulted in 100% of the models yielding positive NB when the decision threshold was set at the average risk. Yet for the more extreme thresholds, there was still substantial risk of harm: 60% of the CPMs that we tested yielded harmful predictions at ≥1 of the extreme thresholds, even after intercept updating. When both the slope and the intercept were updated, 87 of 132 models (66%) were consistently beneficial or nonharmful across all examined thresholds. This underscores the importance of calibration in determining the risk of harm, as well as the importance of clear and consistent reporting of calibration, which is largely absent from the literature. Unfortunately, in many clinical settings, recalibration may not be possible.
Among other notable findings, we discovered that the vast majority of CPMs were impossible to validate on publicly available, patient-level trial data sets. The most common reason was a mismatch between the variables in the models and those collected in the publicly available data sets. Among the CPMs that we were able to validate, discrimination and calibration deteriorated substantially when compared with the derivation cohorts. Interestingly, much of the decrease in discrimination was due to a narrower case mix in the validation cohorts and to model invalidity, although this varied somewhat across the different index conditions. For example, in our examination of ACS models, the median derivation C statistic was 0.76. This decreased on validation to 0.70. Almost all this decrease, however, was due to changes in case mix, not model performance (median MB-C = 0.71). In HF and population models, the decrement in discriminatory performance appeared more evenly, due to case mix and model invalidity.
Our analysis showed the potential usefulness of the MB-C. This is the first large-scale evaluation to apply this rarely used tool. By permitting an estimation of the C statistic based on the variation of predictions only (ie, independent of the actual outcomes), the MB-C permits comparison of the actual C statistic to a more appropriate baseline determined by the case mix in the validation sample, rather than to that in the derivation population. This was particularly germane for our study because the populations used for validation were from publicly available clinical trials, which are generally assumed to have a narrower case mix than registry or real-world populations derived from electronic health records—an assumption suggested by our results.
Similar to aim 1, our analyses in aim 2 showed a larger decrement in discrimination when externally validating a CPM on data from a distantly related cohort than if the cohort were more closely related. Furthermore, the proportion of decrement in model discrimination attributable to model invalidity was somewhat higher when the cohorts were distantly related. Again, relatedness often hinged on subtle but clinically relevant differences between cohorts, such as years of enrollment or the distribution of baseline comorbidities, that required careful review from expert clinicians to identify.
Relevance to Stakeholders
Our findings have important significance for a variety of stakeholders. As major funders of patient-centered clinical research, PCORI should consider developing guidance on clinical prediction as part of its methodology standards. The field has matured so that there is a strong foundation on which to build this guidance, including Prognosis Research Strategy (PROGRESS),12,32-34 Transparent Reporting of a Multivariable Prediction Model for Individual Prognosis or Diagnosis (TRIPOD),35,36 and PROBAST.37,38 Our analyses specifically showed that adherence to items in the analytical domain of PROBAST appears to predict less loss of discriminatory performance when CPMs are tested in external populations.
Prediction is gaining traction in many economic sectors, with the growing prominence of machine learning and artificial intelligence. Although machine learning shows promise for specific applications in medicine (eg, image analysis), results thus far show that these new approaches perform similarly to and have the same limitations as traditional statistical methods.75 Guidance for prediction should apply across these methodologies. Regulatory bodies such as the FDA have become interested in prediction tools, particularly those proposed for use across (rather than within) health care delivery systems and those using less transparent or less interpretable prediction methods. Our results justify the increased scrutiny of model transportability and generalizability, because poor calibration in new data increased the risk of harm and was substantially remedied by recalibration. Coordination with the FDA on prediction standards may be an important opportunity to influence the development of patient-centered prediction tools.
Our results also have relevance for clinicians and patients. In particular, the clinical community assumes that CPMs that withstand peer review are likely to improve prediction and patient care, or that a single evaluation on an external data set means that a model has been validated. Our study shows that many published “validated” models still pose substantial risk of harm in new settings. No risk information may be better than misinformation. If the benefits of improved prediction and evidence personalization are to be realized, the importance of model updating should not be lost on any stakeholder group; patients, providers, and payers should all advocate for the development of an informatics infrastructure that permits routine updating in specific settings in order to provide more trustworthy prognostication in clinical care.
Limitations
Our analysis has several limitations. The sample of data sets used in aims 2 and 3 was a convenience sample, and this sample determined the CPMs selected, because many models were not compatible with the available data sets, usually due to incongruence between variables required for prediction and those collected in the trial. The validation data sets generally represent older therapeutic eras, as this work reflects data sets that are currently available through BioLINCC. Contemporaneous validation cohorts with a broader case mix may have shown smaller decrements in discrimination, though such a finding would underscore our conclusion about the importance of considering the degree of similarity to the derivation cohort when applying a CPM and the importance of using the MB-C for appropriate comparison.
One of the major contributions of this work was developing a shortened version of the PROBAST, suitable for large-scale application, which permitted replication of the full PROBAST classification of CPMs into those having high vs low ROB, with a much lighter burden of assessment. Nevertheless, the short form inherits the limitations of the original PROBAST—most specifically, the vast majority of CPMs are identified as having a high ROB (~95%). Although the high percentage of models with a high ROB might be interpreted as a reflection of the low overall quality of the literature, it might alternatively reflect limitations of the tool. This is suggested by the results of our analysis, which showed substantial variation in both the number of items violated by the individual high-ROB CPMs (suggesting variation in methodological rigor among high-ROB CPMs), and also by the substantial variation in discriminatory performance within this high-ROB group. Future work with the short form might explore whether it is useful to identify an “intermediate” ROB category, which might provide a more graded, less draconian assessment.
Many potential CPM–validation data set matches were not possible because of missing or differently defined variables in the validation data sets. Given the small number of CPM–validation data set matches, we were seldom able to match a CPM to >1 validation data set. A given CPM may perform differently when validated against different cohorts, and more research is required to understand the sources of this variation before validation performance can be used to grade the quality of a model. Our relatedness categorization was one such attempt, but it requires content-area expertise, is inherently subjective, and is difficult to generalize to CPMs for other clinical domains.
Furthermore, our NB analyses focused on 3 arbitrary decision thresholds and were not informed by the relative cost of overtreatment vs undertreatment in the specific clinical context. For decision thresholds even further from the outcome rate than those examined here, we would anticipate even greater risk of harm. Finally, our review did not include any evaluation of randomized trials examining the clinical impact of CPMs.
Future Work and Directions
We have compiled the most comprehensive database on CPMs to date, including comprehensive information on their performance. The information available in this database is summarized in this report and in a searchable, publicly available registry (pacecpmregistry.org). Indeed, consistent with the goal of open science, some of our key findings have already been reported in a recent JAMA viewpoint17 by other investigators based on easily accessible data from our website.
The broad picture we present is of an unregulated and poorly understood landscape in need of “domestication,” if not regulation.76,77 Together with other efforts from the prediction-modeling community to build consensus around best practice and reporting,12,35,37 our work is intended to encourage greater rigor by providing a comprehensive evidence base that can be used to evaluate models for trustworthiness. Nevertheless, synthesis of these measures into a comprehensive rating system remains unfulfilled and an area for future work. There are several important barriers to developing such an evaluation system if the goal is to identify models that can improve clinical decisions. First, it is generally not possible to anticipate net clinical benefit from information presented in most external validations, both because reporting even of conventional performance measures is poor and because NB approaches to evaluate CPMs are rarely used. But just as important is the fact that the suitability and usefulness of a CPM depends on factors that are external to the CPM and to any single evaluation. CPM performance may vary substantially from 1 data set to another. Therefore, there will always be limitations to any general evaluation of CPM performance, because the performance of a CPM can only be evaluated with respect to a specific population, and the relatedness to other populations of interest is typically unknown. Finally, CPMs also need to be evaluated with respect to specific decisions, because what determines a model's usefulness is its performance and classification with respect to a clinically important decision threshold.
Based on our findings here, however, a number of useful criteria can be identified. PROBAST-identified, low-ROB CPMs that have been externally validated in multiple settings, with consistently reasonable discrimination and calibration (eg, a C statistic consistently above ~0.7 and observed outcome rates within 30% of predicted on average) might be preliminary criteria that are feasible to assess and informative. However, these criteria might be very stringent, because very few CPMs would satisfy all these criteria. Indeed, because of the issues discussed, better evaluation of CPMs might require us to rethink the basic paradigm of CPM development and dissemination so that it can be evaluated with respect to specific populations and decisions. An integrated infrastructure that permits CPMs to be regularly updated and that also permits end users to test performance on their own data may be needed to ensure that models are providing helpful information rather than harmful misinformation. Among other requirements supported by the rapid dissemination of linked electronic health records, an integrated infrastructure would require reasonably accurate outcome ascertainment in routine care for local recalibration. This infrastructure is being implemented in many health systems but is far from universal.
Conclusions
Many published cardiovascular CPMs have never been externally validated. Many of those that have been validated have only been validated once or twice, which is not likely to reliably forecast performance. Discrimination and calibration often decrease significantly when CPMs for CVD are tested using data from external populations, leading to substantial risk of net harm, particularly when decision thresholds are not near the population average risk. Model updating can reduce this risk substantially and will likely be needed to realize the full potential of risk-based decision-making.
References
- 1.
- Pauker SG, Kassirer JP. Decision analysis. N Engl J Med. 1987;316(5):250-258. [PubMed: 3540670]
- 2.
- Kohane IS. The twin questions of personalized medicine: who are you and whom do you most resemble? Genome Med. 2009;1(1):4. doi:10.1186/gm4 [PMC free article: PMC2651581] [PubMed: 19348691] [CrossRef]
- 3.
- PCORI Board of Governors. Patient-centered outcomes research. Patient-Centered Outcomes Research Institute. Published 2012. Updated November 7, 2013. Accessed October 30, 2015. https://www
.pcori.org /research-results/about-our-research /patient-centered-outcomes-research - 4.
- Kent DM, Hayward RA. Limitations of applying summary results of clinical trials to individual patients: the need for risk stratification. JAMA. 2007;298(10):1209-1212. [PubMed: 17848656]
- 5.
- Kent DM, Rothwell PM, Ioannidis JP, Altman DG, Hayward RA. Assessing and reporting heterogeneity in treatment effects in clinical trials: a proposal. Trials. 2010;11:85. doi:10.1186/1745-6215-11-85 [PMC free article: PMC2928211] [PubMed: 20704705] [CrossRef]
- 6.
- Rothwell PM, Warlow CP. Prediction of benefit from carotid endarterectomy in individual patients: a risk-modelling study. European Carotid Surgery Trialists' Collaborative Group. Lancet. 1999;353(9170):2105-2110. [PubMed: 10382694]
- 7.
- Kent DM, Paulus JK, van Klaveren D, et al. The Predictive Approaches to Treatment effect Heterogeneity (PATH) statement. Ann Intern Med. 2020;172(1):35-45. [PMC free article: PMC7531587] [PubMed: 31711134]
- 8.
- Kent DM, van Klaveren D, Paulus JK, et al. The Predictive Approaches to Treatment effect Heterogeneity (PATH) statement: explanation and elaboration. Ann Intern Med. 2020;172(1):W1-W25. doi:10.7326/M18-3668 [PMC free article: PMC7750907] [PubMed: 31711094] [CrossRef]
- 9.
- Bouwmeester W, Zuithoff NP, Mallett S, et al. Reporting and methods in clinical prediction research: a systematic review. PLoS Med. 2012;9(5):1-12. [PMC free article: PMC3358324] [PubMed: 22629234]
- 10.
- Wessler BS, Lai Yh L, Kramer W, et al. Clinical prediction models for cardiovascular disease: Tufts Predictive Analytics and Comparative Effectiveness Clinical Prediction Model Database. Circ Cardiovasc Qual Outcomes. 2015;8(4):368-375. [PMC free article: PMC4512876] [PubMed: 26152680]
- 11.
- Wessler BS, Paulus JK, Lundquist CM, et al. Tufts PACE Clinical Predictive Model Registry: update 1990 through 2015. Diagn Progn Res. 2017;1:20. doi:10.1186/s41512-017-0021-2 [PMC free article: PMC6460840] [PubMed: 31093549] [CrossRef]
- 12.
- Steyerberg EW, Moons KG, van der Windt DA, et al. Prognosis Research Strategy (PROGRESS) 3: prognostic model research. PLoS Med. 2013;10(2):e1001381. doi:10.1371/journal.pmed.1001381 [PMC free article: PMC3564751] [PubMed: 23393430] [CrossRef]
- 13.
- Yu T, Vollenweider D, Varadhan R, Li T, Boyd C, Puhan MA. Support of personalized medicine through risk-stratified treatment recommendations – an environmental scan of clinical practice guidelines. BMC Med. 2013;11:7. doi:10.1186/1741-7015-11-7 [PMC free article: PMC3565912] [PubMed: 23302096] [CrossRef]
- 14.
- Steyerberg EW, Vickers AJ, Cook NR, et al. Assessing the performance of prediction models: a framework for traditional and novel measures. Epidemiology. 2010;21(1):128-138. [PMC free article: PMC3575184] [PubMed: 20010215]
- 15.
- Gerds TA, Cai T, Schumacher M. The performance of risk prediction models. Biom J. 2008;50(4):457-479. [PubMed: 18663757]
- 16.
- Vickers A. Prediction models in urology: are they any good, and how would we know anyway? Eur Urol. 2010;57(4):571-573. [PMC free article: PMC2891896] [PubMed: 20071072]
- 17.
- Adibi A, Sadatsafavi M, Ioannidis JPA. Validation and utility testing of clinical prediction models: time to change the approach. JAMA. 2020;162(3):235-236. [PubMed: 32134437]
- 18.
- Siontis GC, Tzoulaki I, Castaldi PJ, Ioannidis JP. External validation of new risk prediction models is infrequent and reveals worse prognostic discrimination. J Clin Epidemiol. 2015;68(1):25-34. [PubMed: 25441703]
- 19.
- Collins GS, de Groot JA, Dutton S, et al. External validation of multivariable prediction models: a systematic review of methodological conduct and reporting. BMC Med Res Methodol. 2014;14:40. [PMC free article: PMC3999945] [PubMed: 24645774]
- 20.
- Van Calster B, McLernon DJ, van Smeden M, Wynants L, Steyerberg EW. Calibration: the Achilles' heel of predictive analytics. BMC Med. 2019;17(1):230. doi:10.1186/s12916-019-1466-7 [PMC free article: PMC6912996] [PubMed: 31842878] [CrossRef]
- 21.
- Van Calster B, Vickers AJ. Calibration of risk prediction models: impact on decision-analytic performance. Med Decis Making. 2015;35(2):162-169. [PubMed: 25155798]
- 22.
- Wessler BS, Lundquist CM, Koethe B, et al. Clinical prediction models for valvular heart disease. J Am Heart Assoc. 2019;8(20):e011972. doi:10.1161/JAHA.119.011972 [PMC free article: PMC6818049] [PubMed: 31583938] [CrossRef]
- 23.
- Goff DC Jr, Lloyd-Jones DM, Bennett G, et al. 2013 ACC/AHA guideline on the assessment of cardiovascular risk: a report of the American College of Cardiology/American Heart Association Task Force on Practice Guidelines. J Am Coll Cardiol. 2014;63(25 Pt B):2935-2959. [PMC free article: PMC4700825] [PubMed: 24239921]
- 24.
- Stone NJ, Robinson JG, Lichtenstein AH, et al. 2013 ACC/AHA guideline on the treatment of blood cholesterol to reduce atherosclerotic cardiovascular risk in adults: a report of the American College of Cardiology/American Heart Association Task Force on Practice Guidelines. J Am Coll Cardiol. 2014;63(25 Pt B):2889-2934. [PubMed: 24239923]
- 25.
- Ridker PM, Cook NR. Statins: New American guidelines for prevention of cardiovascular disease. Lancet. 2013;382(9907):1762-1765. [PubMed: 24268611]
- 26.
- Cook NR. Use and misuse of the receiver operating characteristic curve in risk prediction. Circulation. 2007;115(7):928-935. [PubMed: 17309939]
- 27.
- Pencina MJ, D'Agostino RB, D'Agostino RB Jr, Vasan RS. Evaluating the added predictive ability of a new marker: from area under the ROC curve to reclassification and beyond. Stat Med. 2008;27(2):157-172. [PubMed: 17569110]
- 28.
- Vickers AJ, Elkin EB. Decision curve analysis: a novel method for evaluating prediction models. Med Decis Making. 2006;26(6):565-574. [PMC free article: PMC2577036] [PubMed: 17099194]
- 29.
- Vickers AJ, Cronin AM, Elkin EB, Gonen M. Extensions to decision curve analysis, a novel method for evaluating diagnostic tests, prediction models and molecular markers. BMC Med Inform Decis Mak. 2008;8:53. doi:10.1186/1472-6947-8-53 [PMC free article: PMC2611975] [PubMed: 19036144] [CrossRef]
- 30.
- Steyerberg EW, Vickers AJ. Decision curve analysis: a discussion. Med Decis Making. 2008;28(1):146-149. [PMC free article: PMC2577563] [PubMed: 18263565]
- 31.
- Peirce CS. The numerical measure of the success of predictions. Science. 1884;4(93):453-454. [PubMed: 17795531]
- 32.
- Hemingway H, Croft P, Perel P, et al. Prognosis Research Strategy (PROGRESS) 1: a framework for researching clinical outcomes. BMJ. 2013;346:e5595. doi:10.1136/bmj.e5595 [PMC free article: PMC3565687] [PubMed: 23386360] [CrossRef]
- 33.
- Riley RD, Hayden JA, Steyerberg EW, et al. Prognosis Research Strategy (PROGRESS) 2: prognostic factor research. PLoS Med. 2013;10(2):e1001380. doi:10.1371/journal.pmed.1001380 [PMC free article: PMC3564757] [PubMed: 23393429] [CrossRef]
- 34.
- Hingorani AD, Windt DA, Riley RD, et al. Prognosis Research Strategy (PROGRESS) 4: stratified medicine research. BMJ. 2013;346:e5793. doi:10.1136/bmj.e5793 [PMC free article: PMC3565686] [PubMed: 23386361] [CrossRef]
- 35.
- Collins GS, Reitsma JB, Altman DG, Moons KG; TRIPOD Group. Transparent Reporting of a Multivariable Prediction Model for Individual Prognosis or Diagnosis (TRIPOD): the TRIPOD statement. Circulation. 2015;131(2):211-219. [PMC free article: PMC4297220] [PubMed: 25561516]
- 36.
- Tangri N, Kent DM. Toward a modern era in clinical prediction: the TRIPOD statement for reporting prediction models. Am J Kidney Dis. 2015;65(4):530-533. [PubMed: 25600952]
- 37.
- Wolff RF, Moons KGM, Riley RD, et al. PROBAST: a tool to assess the risk of bias and applicability of prediction model studies. Ann Intern Med. 2019;170(1):51-58. [PubMed: 30596875]
- 38.
- Moons KGM, Wolff RF, Riley RD, et al. PROBAST: a tool to assess risk of bias and applicability of prediction model studies: explanation and elaboration. Ann Intern Med. 2019;170(1):W1-W33. doi:10.7326/M18-1377 [PubMed: 30596876] [CrossRef]
- 39.
- Hess EP, Wyatt KD, Kharbanda AB, et al. Effectiveness of the head CT choice decision aid in parents of children with minor head trauma: study protocol for a multicenter randomized trial. Trials. 2014;15:253. doi:10.1186/1745-6215-15-253 [PMC free article: PMC4081461] [PubMed: 24965659] [CrossRef]
- 40.
- Hess EP, Knoedler MA, Shah ND, et al. The Chest Pain Choice decision aid: a randomized trial. Circ Cardiovasc Qual Outcomes. 2012;5(3):251-259. [PubMed: 22496116]
- 41.
- Anderson RT, Montori VM, Shah ND, et al. Effectiveness of the Chest Pain Choice decision aid in emergency department patients with low-risk chest pain: study protocol for a multicenter randomized trial. Trials. 2014;15:166. doi:10.1186/1745-6215-15-166 [PMC free article: PMC4031497] [PubMed: 24884807] [CrossRef]
- 42.
- van Klaveren D, Gönen M, Steyerberg EW, Vergouwe Y. A new concordance measure for risk prediction models in external validation settings. Stat Med. 2016;35(23):4136-4152. [PMC free article: PMC5550798] [PubMed: 27251001]
- 43.
- Beta-Blocker Heart Attack Trial Research Group. A randomized trial of propranolol in patients with acute myocardial infarction. I. Mortality results. JAMA. 1982;247(12):1707-1714. [PubMed: 7038157]
- 44.
- Pitt B, Pfeffer MA, Assmann SF, et al. Spironolactone for heart failure with preserved ejection fraction. N Engl J Med. 2014;370(15):1383-1392. [PubMed: 24716680]
- 45.
- O'Connor CM, Whellan DJ, Lee KL, et al. Efficacy and safety of exercise training in patients with chronic heart failure: HF-ACTION randomized controlled trial. JAMA. 2009;301(14):1439-1450. [PMC free article: PMC2916661] [PubMed: 19351941]
- 46.
- Bardy GH, Lee KL, Mark DB, et al. Amiodarone or an implantable cardioverter-defibrillator for congestive heart failure. N Engl J Med. 2005;352(3):225-237. [PubMed: 15659722]
- 47.
- Beta-Blocker Evaluation of Survival Trial Investigators; Eichhorn EJ, Domanski MJ, Krause-Steinrauf H, Bristow MR, Lavori PW. A trial of the beta-blocker bucindolol in patients with advanced chronic heart failure. N Engl J Med. 2001;344(22):1659-1667. [PubMed: 11386264]
- 48.
- Digitalis Investigation Group. The effect of digoxin on mortality and morbidity in patients with heart failure. N Engl J Med. 1997;336(8):525-533. [PubMed: 9036306]
- 49.
- SOLVD Investigators; Yusuf S, Pitt B, Davis CE, Hood WB, Cohn JN. Effect of enalapril on survival in patients with reduced left ventricular ejection fractions and congestive heart failure. N Engl J Med. 1991;325(5):293-302. [PubMed: 2057034]
- 50.
- Aspirin Myocardial Infarction Study Research Group. A randomized, controlled trial of aspirin in persons recovered from myocardial infarction. JAMA. 1980;243(7):661-669. [PubMed: 6985998]
- 51.
- Cannon CP, Weintraub WS, Demopoulos LA, et al. Comparison of early invasive and conservative strategies in patients with unstable coronary syndromes treated with the glycoprotein IIb/IIIa inhibitor tirofiban. N Engl J Med. 2001;344(25):1879-1887. [PubMed: 11419424]
- 52.
- Effects of tissue plasminogen activator and a comparison of early invasive and conservative strategies in unstable angina and non-Q-wave myocardial infarction. Results of the TIMI IIIB Trial. Thrombolysis in Myocardial Ischemia. Circulation. 1994;89(4):1545-1556. [PubMed: 8149520]
- 53.
- Magnesium in Coronaries (MAGIC) Trial Investigators. Early administration of intravenous magnesium to high-risk patients with acute myocardial infarction in the Magnesium in Coronaries (MAGIC) Trial: a randomised controlled trial. Lancet. 2002;360(9341):1189-1196. [PubMed: 12401244]
- 54.
- Berkman LF, Blumenthal J, Burg M, et al. Effects of treating depression and low perceived social support on clinical events after myocardial infarction: the Enhancing Recovery in Coronary Heart Disease Patients (ENRICHD) randomized trial. JAMA. 2003;289(23):3106-3116. [PubMed: 12813116]
- 55.
- Action to Control Cardiovascular Risk in Diabetes Study Group; Gerstein HC, Miller ME, et al. Effects of intensive glucose lowering in type 2 diabetes. N Engl J Med. 2008;358(24):2545-2559. [PMC free article: PMC4551392] [PubMed: 18539917]
- 56.
- ALLHAT Officers and Coordinators for the ALLHAT Collaborative Research Group. Major outcomes in high-risk hypertensive patients randomized to angiotensin-converting enzyme inhibitor or calcium channel blocker vs diuretic: The Antihypertensive and Lipid-Lowering Treatment to Prevent Heart Attack Trial (ALLHAT). JAMA. 2002;288(23):2981-2997. [PubMed: 12479763]
- 57.
- ALLHAT Officers and Coordinators for the ALLHAT Collaborative Research Group. Major outcomes in moderately hypercholesterolemic, hypertensive patients randomized to pravastatin vs usual care: the Antihypertensive and Lipid-Lowering Treatment to Prevent Heart Attack Trial (ALLHAT-LLT). JAMA. 2002;288(23):2998-3007. [PubMed: 12479764]
- 58.
- Rossouw JE, Anderson GL, Prentice RL, et al. Risks and benefits of estrogen plus progestin in healthy postmenopausal women: principal results from the Women's Health Initiative randomized controlled trial. JAMA. 2002;288(3):321-333. [PubMed: 12117397]
- 59.
- TIMI II Study Group. Comparison of invasive and conservative strategies after treatment with intravenous tissue plasminogen activator in acute myocardial infarction. Results of the thrombolysis in myocardial infarction (TIMI) phase II trial. N Engl J Med. 1989;320(10):618-627. [PubMed: 2563896]
- 60.
- Konstam MA, Neaton JD, Dickstein K, et al. Effects of high-dose versus low-dose losartan on clinical outcomes in patients with heart failure (HEAAL study): a randomised, double-blind trial. Lancet. 2009;374(9704):1840-1848. [PubMed: 19922995]
- 61.
- Konstam MA, Gheorghiade M, Burnett JC Jr, et al. Effects of oral tolvaptan in patients hospitalized for worsening heart failure: the EVEREST outcome trial. JAMA. 2007;297(12):1319-1331. [PubMed: 17384437]
- 62.
- Damen J, Hooft L, Schuit E, et al. Prediction models for cardiovascular disease risk in the general population: systematic review. BMJ. 2016;353:i2416. doi:10.1136/bmj.i2416 [PMC free article: PMC4868251] [PubMed: 27184143] [CrossRef]
- 63.
- Wessler BS, Ruthazer R, Udelson JE, et al. Regional validation and recalibration of clinical predictive models for patients with acute heart failure. J Am Heart Assoc. 2017;6(11):e006121. doi:10.1161/JAHA.117.006121 [PMC free article: PMC5721739] [PubMed: 29151026] [CrossRef]
- 64.
- Kerr KF, Brown MD, Zhu K, Janes H. Assessing the clinical impact of risk prediction models with decision curves: guidance for correct interpretation and appropriate use. J Clin Oncol. 2016;34(21):2534-2540. [PMC free article: PMC4962736] [PubMed: 27247223]
- 65.
- Steyerberg EW, Borsboom GJ, van Houwelingen HC, Eijkemans MJ, Habbema JD. Validation and updating of predictive logistic regression models: a study on sample size and shrinkage. Stat Med. 2004;23(16):2567-2586. [PubMed: 15287085]
- 66.
- Kent DM, Wessler BS, Lundquist CM, Lutz JS. How well do clinical prediction models (CPMs) validate? A large-scale evaluation of cardiovascular clinical prediction models. PROSPERO 2017 CRD42017060913. Accessed October 21, 2021. https://www
.crd.york .ac.uk/prospero/display_record .php?ID=CRD42017060913 - 67.
- Roques F, Michel P, Goldstone AR, Nashef SA. The logistic EuroSCORE. Eur Heart J. 2003;24(9):881-882. [PubMed: 12727160]
- 68.
- Steyerberg EW. Clinical Prediction Models: A Practical Approach to Development, Validation, and Updating. Springer; 2009.
- 69.
- Wilson PW, D'Agostino RB, Levy D, Belanger AM, Silbershatz H, Kannel WB. Prediction of coronary heart disease using risk factor categories. Circulation. 1998;97(18):1837-1847. [PubMed: 9603539]
- 70.
- Mehta S, Wood D, Storey R, et al. Complete revascularization with multivessel PCI for myocardial infarction. N Engl J Med. 2019;381(15):1411-1421. [PubMed: 31475795]
- 71.
- Piccolo R, Bonaa K, Efthimiou O, et al. Drug-eluting or bare-metal stents for percutaneous coronary intervention: a systematic review and individual patient data meta-analysis of randomised clinical trials. Lancet. 2019;393(10190):2503-2510. [PubMed: 31056295]
- 72.
- Kip K, Hollabaugh K, Marroquin O, Williams D. The problem with composite end points in cardiovascular studies: the story of major adverse cardiac events and percutaneous coronary intervention. J Am Coll Cardiol. 2008;51(7):701-707. [PubMed: 18279733]
- 73.
- Mehran R, Rao S, Bhatt D, et al. Standardized bleeding definitions for cardiovascular clinical trials: a consensus report from the Bleeding Academic Research Consortium. Circulation. 2011;123(23):2736-2747. [PubMed: 21670242]
- 74.
- Califf RM, Pieper KS, Lee KL, et al. Prediction of 1-year survival after thrombolysis for acute myocardial infarction in the Global Utilization of Streptokinase and TPA for Occluded Coronary Arteries trial. Circulation. 2000;101(19):2231-2238. [PubMed: 10811588]
- 75.
- Christodoulou E, Ma J, Collins GS, Steyerberg EW, Verbakel JY, Van Calster B. A systematic review shows no performance benefit of machine learning over logistic regression for clinical prediction models. J Clin Epidemiol. 2019;110:12-22. [PubMed: 30763612]
- 76.
- Parikh RB, Obermeyer Z, Navathe AS. Regulation of predictive analytics in medicine: algorithms must meet regulatory standards of clinical benefit. Science. 2019;363(6429):810-812. [PMC free article: PMC6557272] [PubMed: 30792287]
- 77.
- Shah N, Steyerberg E, Kent D. Big data and predictive analytics: recalibrating expectations. JAMA. 2018;320(1):27-28. [PubMed: 29813156]
Related Publications
- Upshaw JN, Nelson J, Koethe B, et al. Performance of heart failure clinical prediction models: a systematic external validation study. medRxiv. Preprint posted online February 1, 2021. doi:10.1101/2021.01.31.21250875 [CrossRef]
- Wessler BS, Nelson J, Park JG, et al. External validations of cardiovascular clinical prediction models: a large-scale review of the literature. Circ Cardiovasc Qual Outcomes. 2021;14(8):e007858. doi:10.1161/CIRCOUTCOMES.121.007858 [PMC free article: PMC8366535] [PubMed: 34340529] [CrossRef]
- Venema E, Wessler BS, Paulus JK, et al. Large-scale validation of the prediction model risk of bias assessment Tool (PROBAST) using a short form: high risk of bias models show poorer discrimination. J Clin Epidemiol. 2021;138:32-39. doi:10.1016/j.jclinepi.2021.06.017 [PubMed: 34175377] [CrossRef]
- Carrick RT, Park JG, Lundquist CM, et al. Clinical predictive models of sudden cardiac arrest: a survey of the current science and analysis of model performances. J Am Heart Assoc. 2020;9(16):e017625. doi:10.1161/JAHA.119.017625 [PMC free article: PMC7660807] [PubMed: 32787675] [CrossRef]
- Paulus J, Kent DM. Predictably unequal: understanding and addressing concerns that algorithmic clinical prediction may increase health disparities. NPJ Digit Med. 2020;3,99. doi:10.1038/s41746-020-0304-9 [PMC free article: PMC7393367] [PubMed: 32821854] [CrossRef]
- Wessler BS, Kent DM, Konstam MA. Fear of coronavirus disease 2019—an emerging cardiac risk. JAMA Cardiol. 2020;5(9):981-982. [PMC free article: PMC7999782] [PubMed: 32936280]
- Kent DM, Paulus JK, Sharp RR, Hajizadeh N. When predictions are used to allocate scarce health care resources: three considerations for models in the era of Covid-19. Diagn Progn Res. 2020;4:11. doi:10.1186/s41512-020-00079-y [PMC free article: PMC7238723] [PubMed: 32455168] [CrossRef]
- Austin PC, Harrell FE Jr, van Klaveren D. Graphical calibration curves and the integrated calibration index (ICI) for survival models. Stat Med. 2020;39(21):2714-2742. [PMC free article: PMC7497089] [PubMed: 32548928]
- Luijken K, Wynants L, van Smeden M, Van Calster B, Steyerberg EW, Groenwold RH. Changing predictor measurement procedures affected the performance of prediction models in clinical examples. J Clin Epidemiol. 2020;119:7-18. [PubMed: 31706963]
- Wessler BS, Lundquist CM, Koethe B, et al. Clinical prediction models for valvular heart disease. J Am Heart Assoc. 2019;8(20):e011972. doi:10.1161/JAHA.119.011972 [PMC free article: PMC6818049] [PubMed: 31583938] [CrossRef]
- Van Klaveren D, Balan TA, Steyerberg EW, Kent DM. Models with interactions overestimated heterogeneity of treatment effects and were prone to treatment mistargeting. J Clin Epidemiol. 2019;114:72‐83. [PMC free article: PMC7497896] [PubMed: 31195109]
- van Klaveren D, Steyerberg EW, Gönen M, Vergouwe Y. The calibrated model-based concordance improved assessment of discriminative ability in patient clusters of limited sample size. Diagn Progn Res. 2019;3:11. doi:10.1186/s41512-019-0055-8 [PMC free article: PMC6551913] [PubMed: 31183411] [CrossRef]
- Luijken K, Groenwold RHH, van Calster B, Steyerberg EW, van Smeden M. Impact of predictor measurement heterogeneity across settings on performance of prediction models: a measurement error perspective. Stat Med. 2019;38(18):3444-3459. [PMC free article: PMC6619392] [PubMed: 31148207]
- Steyerberg EW, Nieboer D, Debray TPA, van Houwelingen HC. Assessment of heterogeneity in an individual participant data meta-analysis of prediction models: an overview and illustration. Stat Med. 2019;38(22):4290-4309. [PMC free article: PMC6772012] [PubMed: 31373722]
- Austin PC, Steyerberg EW. The Integrated Calibration Index (ICI) and related metrics for quantifying the calibration of logistic regression models. Stat Med. 2019;8(21):4051-4065. [PMC free article: PMC6771733] [PubMed: 31270850]
- Wynants L, Kent D, Timmerman D, Lundquist C, Van Calster B. Untapped potential of multicenter studies: a review of cardiovascular risk prediction models revealed inappropriate analyses and wide variation in reporting. Diagn Progn Res. 2019;3:6. doi:10.1186/s41512-019-0046-9 [PMC free article: PMC6460661] [PubMed: 31093576] [CrossRef]
- Van Calster B, Wynants L, Verbeek JFM, et al. Reporting and interpreting decision curve analysis: a guide for investigators. Eur Urol. 2018;74(6):796-804. [PMC free article: PMC6261531] [PubMed: 30241973]
- Fusar-Poli P, Hijazi Z, Stahl D, Steyerberg E. The science of prognosis in psychiatry: a review. JAMA Psychiatry. 2018;75(12):1289-1297. [PubMed: 30347013]
- Steyerberg EW. Validation in prediction research: the waste by data splitting. J Clin Epidemiol. 2018;103:131-133. [PubMed: 30063954]
- Paulus JK, Wessler BS, Lundquist CM, Kent DM. Effects of race are rarely included in clinical prediction models for cardiovascular disease. J Gen Intern Med. 2018p;33(9):1429-1430. [PMC free article: PMC6109012] [PubMed: 29766380]
- Steyerberg EW, Uno H, Ioannidis JPA, van Calster B. Poor performance of clinical prediction models: the harm of commonly applied methods. J Clin Epidemiol. 2018;98:133-143. [PubMed: 29174118]
- Steyerberg EW, Claggett B. Towards personalized therapy for multiple sclerosis: limitations of observational data. Brain. 2018;141(5):e38. doi:10.1093/brain/awy055 [PubMed: 29514218] [CrossRef]
- Shah ND, Steyerberg EW, Kent DM. Big data and predictive analytics: recalibrating expectations. JAMA. 2018;320(1):27-28. [PubMed: 29813156]
- Wessler BS, Paulus J, Lundquist CM, et al. Tufts PACE Clinical Predictive Model Registry: update1990 through 2015. Diagn Progn Res. 2017;1:20. doi:10.1186/s41512-017-0021-2 [PMC free article: PMC6460840] [PubMed: 31093549] [CrossRef]
- Paulus JK, Kent DM. Race and ethnicity: a part of the equation for personalized decision making? Circ Cardiovasc Qual Outcomes. 2017;10(7):e003823. doi:10.1161/CIRCOUTCOMES.117.003823 [PMC free article: PMC5558842] [PubMed: 28687570] [CrossRef]
- Wessler BS, Ruthazer R, Udelson JE, et al. Regional validation and recalibration of clinical predictive models for patients with acute heart failure. J Am Heart Assoc. 2017;6(11):e006121. doi:10.1161/JAHA.117.006121 [PMC free article: PMC5721739] [PubMed: 29151026] [CrossRef]
- Wessler BS, Nelson J, Lundquist CM, et al. The generalizability of clinical prediction models for patients with acute coronary syndromes: results from independent external validations. medRxiv. Preprint posted online January 22, 2021. doi:10.1101/2021.01.21.21250234 [CrossRef]
- Brazil R, Gulati G, Nelson J, et al. The generalizability of clinical predictive models for primary prevention of cardiovascular disease: results from independent external validations. J Am Coll Cardiol. 2020;71(11 suppl 1):1925. Accessed September 14, 2021. https://www
.jacc.org/doi/full/10 .1016/S0735-1097 %2820%2932552-3 - Wessler BS, Nelson J, Park JG, et al. External validations of cardiovascular clinical prediction models: a large-scale review of the literature. medRxiv. Preprint posted online January 21, 2021. https://www
.medrxiv.org/content/10 .1101/2021 .01.19.21250110v1 [PMC free article: PMC8366535] [PubMed: 34340529]
- Gulati G, Brazil RJ, Nelson J, et al. Clinical prediction models for primary prevention of cardiovascular disease: validity in independent cohorts. medRxiv. Preprint posted online February 3, 2021. doi:10.1101/2021.01.31.21250871 [CrossRef]
- Upshaw JN, Nelson J, Koethe B, et al. Performance of heart failure clinical prediction models: a systematic external validation study. medRxiv. Preprint posted online February 1, 2021. doi:10.1101/2021.01.31.21250875 [CrossRef]
- Venema E, Wessler BS, Paulus JK, et al. Large-scale validation of the prediction model risk of bias assessment Tool (PROBAST) using a short form: high risk of bias models show poorer discrimination. J Clin Epidemiol. 2021;138:32-39. doi:10.1016/j.jclinepi.2021.06.017 [PubMed: 34175377] [CrossRef]
- Wessler BS, Nelson J, Park JG, et al. External validations of cardiovascular clinical prediction models: a large-scale review of the literature. Circ Cardiovasc Qual Outcomes. 2021;14(8):e007858. doi:10.1161/CIRCOUTCOMES.121.007858 [PMC free article: PMC8366535] [PubMed: 34340529] [CrossRef]
- Wessler BS, Nelson J, Park J, et al. The generalizability of clinical prediction models for patients with acute coronary syndromes: results from independent external validations. medRxiv. Preprint posted online January 22, 2021. doi:10.1101/2021.01.21.21250234 [CrossRef]
Note: Much of the material presented in this report is adapted from the following publications:
Acknowledgments
This report borrowed heavily from the publications listed in the Related Publications section. We are indebted to all coauthors of these articles. We are also indebted to the investigators and participants of the randomized trials in the data sets for aims 2 and 3. We thank our PCORI Program Officers (Drs Jason Gerson and Emily Evans) for discussion and feedback regarding the design and conduct of this research. We are also indebted to the stakeholders who provided critical feedback that shaped the direction of this work.
Research reported in this report was funded through a Patient-Centered Outcomes Research Institute® (PCORI®) Award (ME-1606-35555). Further information available at: https://www.pcori.org/research-results/2016/using-different-data-sets-test-how-well-clinical-prediction-models-work
Appendices
Appendix A. Search Strategy
Appendix B. Population Relatedness
Appendix B Table 3. Arrhythmia Rubric and Guide (PDF, 116K)
Appendix B Table 8. Stroke Rubric and Guide (PDF, 93K)
Appendix C. Short-Form Guidelines
Appendix C Item 1. Short Form Guidelines (PDF, 139K)
Appendix D. Clinical Trials for Aims 2 and 3
Appendix E. Relatedness Rubrics for Aims 2 and 3
Appendix E Table 1. ACS relatedness rubric (PDF, 93K)
Appendix E Table 2. HF relatedness rubric (PDF, 84K)
Appendix F. Aim 2 Original Model Validation
Appendix G.
Abbreviations (PDF, 101K)
Suggested citation:
Kent DM, Nelson J, Upshaw JN, et al. (2021). Using Different Data Sets to Test How Well Clinical Prediction Models Work to Predict Patients' Risk of Heart Disease. Patient-Centered Outcomes Research Institute (PCORI). https://doi.org/10.25302/09.2021.ME.160635555
Disclaimer
The [views, statements, opinions] presented in this report are solely the responsibility of the author(s) and do not necessarily represent the views of the Patient-Centered Outcomes Research Institute® (PCORI®, its Board of Governors or Methodology Committee.
- Review External Validations of Cardiovascular Clinical Prediction Models: A Large-Scale Review of the Literature.[Circ Cardiovasc Qual Outcomes....]Review External Validations of Cardiovascular Clinical Prediction Models: A Large-Scale Review of the Literature.Wessler BS, Nelson J, Park JG, McGinnes H, Gulati G, Brazil R, Van Calster B, van Klaveren D, Venema E, Steyerberg E, et al. Circ Cardiovasc Qual Outcomes. 2021 Aug; 14(8):e007858. Epub 2021 Aug 3.
- Generalizability of Cardiovascular Disease Clinical Prediction Models: 158 Independent External Validations of 104 Unique Models.[Circ Cardiovasc Qual Outcomes....]Generalizability of Cardiovascular Disease Clinical Prediction Models: 158 Independent External Validations of 104 Unique Models.Gulati G, Upshaw J, Wessler BS, Brazil RJ, Nelson J, van Klaveren D, Lundquist CM, Park JG, McGinnes H, Steyerberg EW, et al. Circ Cardiovasc Qual Outcomes. 2022 Apr; 15(4):e008487. Epub 2022 Mar 31.
- Folic acid supplementation and malaria susceptibility and severity among people taking antifolate antimalarial drugs in endemic areas.[Cochrane Database Syst Rev. 2022]Folic acid supplementation and malaria susceptibility and severity among people taking antifolate antimalarial drugs in endemic areas.Crider K, Williams J, Qi YP, Gutman J, Yeung L, Mai C, Finkelstain J, Mehta S, Pons-Duran C, Menéndez C, et al. Cochrane Database Syst Rev. 2022 Feb 1; 2(2022). Epub 2022 Feb 1.
- Large-scale validation of the prediction model risk of bias assessment Tool (PROBAST) using a short form: high risk of bias models show poorer discrimination.[J Clin Epidemiol. 2021]Large-scale validation of the prediction model risk of bias assessment Tool (PROBAST) using a short form: high risk of bias models show poorer discrimination.Venema E, Wessler BS, Paulus JK, Salah R, Raman G, Leung LY, Koethe BC, Nelson J, Park JG, van Klaveren D, et al. J Clin Epidemiol. 2021 Oct; 138:32-39. Epub 2021 Jun 24.
- Review Tufts PACE Clinical Predictive Model Registry: update 1990 through 2015.[Diagn Progn Res. 2017]Review Tufts PACE Clinical Predictive Model Registry: update 1990 through 2015.Wessler BS, Paulus J, Lundquist CM, Ajlan M, Natto Z, Janes WA, Jethmalani N, Raman G, Lutz JS, Kent DM. Diagn Progn Res. 2017; 1:20. Epub 2017 Dec 21.
- Using Different Data Sets to Test How Well Clinical Prediction Models Work to Pr...Using Different Data Sets to Test How Well Clinical Prediction Models Work to Predict Patients' Risk of Heart Disease
Your browsing activity is empty.
Activity recording is turned off.
See more...