NCBI Bookshelf. A service of the National Library of Medicine, National Institutes of Health.
Varki A, Cummings RD, Esko JD, et al., editors. Essentials of Glycobiology [Internet]. 3rd edition. Cold Spring Harbor (NY): Cold Spring Harbor Laboratory Press; 2015-2017. doi: 10.1101/glycobiology.3e.030
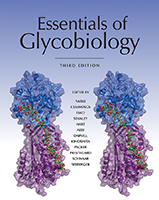
Essentials of Glycobiology [Internet]. 3rd edition.
Show detailsThe biological effects that glycans elicit are frequently dependent on recognition of specific glycan features by the proteins with which they interact. In this chapter, some of the key structural features underlying glycan–protein interactions, as well as the primary experimental methods that have led to an understanding of these features, are discussed, specifically X-ray crystallography, nuclear magnetic resonance (NMR), and computational modeling.
BACKGROUND
As emphasized in previous chapters, the numbers of distinct glycans produced by various organisms is enormous, but at the same time, glycans lack the diversity in functional groups displayed by other molecules. To achieve specificity in glycan recognition, proteins rely as much on the actual stereospecific placement of glycan hydroxyl groups at chiral centers, use of different linkage sites, and extensive branching as they rely on specific modifications of hydroxyl groups by processes such as sulfation, phosphorylation, and esterification. This puts placement of various residues and functional groups in three dimensions at a premium. Building a three-dimensional picture of how recognition of glycans by proteins occurs is therefore essential if we are to understand how glycans are synthesized and recognized in the many physiological and pathological processes they control. It is also essential if we are to use knowledge of glycan recognition as a basis for the production of therapeutic agents that can control these processes in the event of disease. Building a structure depicting glycan recognition is not without its challenges. Most glycans are highly dynamic in solution, sampling many conformations. Often, a single or a small subset of conformations is selected when a complex forms. This works against the formation of stable complexes for structural study and the direct use of solution conformational data in defining conformations of bound glycans.
The search for a structural basis of glycan recognition by proteins is not new. The concept of glycans fitting into pockets on protein surfaces dates back to Emil Fischer, who used the phrase “lock and key” to refer to enzymes that recognize specific glycan substrates. Lysozyme was the first “carbohydrate-binding protein” to be crystallized and have its three-dimensional structure determined. Subsequent work in the late 1960s and early 1970s led to a structure complexed with a tetrasaccharide that confirmed the existence of specific interactions occurring between sugars and proteins, and the ability of proteins to select the appropriate “key” from numerous possibilities.
Today, protein crystallography has reached a very high degree of sophistication and is responsible for the vast majority of the more than 100,000 structures deposited in the Protein Data Bank (PDB); however, producing a structure with ligands in place is still challenging. The structures that exist tend to have relatively small ligands and ligands with particularly high binding constants. Glycan recognition frequently involves contacts with multiple residues to achieve specificity. So, native glycan ligands are often larger than other types of ligands. Often, high avidity is achieved through multivalent interactions, in which case the affinity for an isolated ligand–protein interaction is small. Nevertheless, there are a significant number of crystal structures for glycan–protein complexes, and these have contributed greatly to our understanding of the types of interactions that make glycan recognition possible.
Structural information on bound glycan ligands that is complementary to that from X-ray crystallography is increasingly coming from NMR methods. This is particularly valuable in that it is applicable to ligands with a broader range of affinities, including many that have the lower affinities amplified in multivalent interactions. It is also applicable in solution under near physiological conditions in which concerns about the effects of crystal lattice contacts and occlusion of some interaction sites are absent. It is even possible to conduct some experiments on assemblies that mimic a membrane surface environment, an environment where many protein–glycan interactions occur.
Although we will not discuss specific contributions in this chapter, it is important to note that structural methodology is continually evolving, with additional information coming from techniques like small-angle X-ray scattering (SAXS) and cryo-electron microscopy (cryo-EM). Recent advances in cryo-EM have been particularly noteworthy with resolution beginning to push that of X-ray crystallography without the need for crystallization or large amounts of material.
The fundamental understanding of glycan–protein interactions, as enriched by experimental studies of all types, has now been encoded in powerful molecular simulation programs that provide a computational approach to generating three dimensional pictures of glycan–protein interactions. These are important because it is difficult to produce complex glycan ligands in the amounts and purity required for most experimental approaches. These methods, although still evolving toward reasonable confidence in outcomes, provide models for experimentally inaccessible systems that can be tested with a variety of nonstructural approaches. They can also be leveraged with sparse structural data that alone could not provide detailed structural information.
CRYSTALLOGRAPHY
X-ray crystallography is a very powerful method for obtaining details of protein–ligand interactions. It excels in terms of the size range of molecules that can be studied (from small compounds to large multiprotein complexes) and in efficiency of data collection when high-energy light beams at synchrotron sources are used. One of the limitations is still the crystallization step. Crystals of protein–carbohydrate complexes can be obtained by cocrystallizing the two partners or by soaking the carbohydrate ligand into an existing protein crystal. Because the quality of the crystal defines the limit of the diffraction pattern, and therefore the resolution of the structure, flexible oligosaccharide ligands may create structural heterogeneity and therefore limit the quality of the crystal. High-quality crystals of lectins are generally obtained with glycans ranging from mono- to trisaccharides; glycosaminoglycan (GAG)-binding proteins or antibodies, which can bind much larger ligands, are more rarely crystallized in complex with carbohydrate ligands.
Diffraction data are now typically collected at very low temperature, to protect molecules from radiation damage on high-energy synchrotron beam lines. Because freezing may damage the crystals owing to ice formation, glycerol is often used as cryoprotectant. Glycerol, with its carbohydrate-like hydroxylated carbons, is therefore frequently observed in glycan-binding sites, providing information about the amino acids involved in binding but sometimes competing with the carbohydrate ligand. When data with sufficient resolution can be collected, other obstacles, such as the lack of phase information in scattered X-ray intensities are encountered. This problem is particularly challenging when studying proteins with a novel fold, as molecular replacement cannot be used to assign phases. Carbohydrate chemistry can bring a solution to the problem, because selenium derivatives of monosaccharides can be incorporated in ligands and anomalous scattering from elements like selenium can provide phasing information.
Databases of Crystal Structures
Crystal structures of protein–carbohydrate complexes can be retrieved from different sources, including the PDB, but also from more specialized databases. The Carbohydrate-Active Enzymes (CAZY) database provides links to the PDB page for all crystal structures of glycosylhydrolases, glycosyltransferases, and their associated carbohydrate-binding modules. The Glyco 3D portal is a suite of searchable databases covering the three-dimensional features of bioactive carbohydrates but also of glycosyltransferases, lectins, monoclonal antibodies against carbohydrates, and GAG-binding proteins. As an example from this portal, the Lectin-3D database includes more than 1500 lectin three-dimensional structures (285 different proteins), 64% of these structures being determined in complex with a carbohydrate ligand. For each structure, links for coordinates, references, and taxonomy are provided, as well as glycan array data when available at the Consortium for Functional Glycomics. Mining for structural data is therefore possible, and structures can be analyzed at different levels revealing not only atomic details of the binding sites but also protein folds and oligomeric states. Examples are given below that illustrate how convergent evolution has built robust systems for efficient recognition of glycans by lectins.
Interactions in Carbohydrate-Binding Sites
The interactions between carbohydrates and amino acids include hydrogen bonds, van der Waals contacts, ionic bonds, and a number of more specialized interactions. CH-π interactions, for example, are associated with the frequent occurrence of aromatic amino acids in carbohydrate-binding sites. Water molecules are often observed that bridge between carbohydrate hydroxyl groups and amino acids. Interestingly, a significant number of enzyme or lectins use divalent ions that directly coordinate to the hydroxyl groups of carbohydrates and to side chains of amino acids. Among the 260 different lectins crystallized to date, more than 20 involve calcium ions in their binding sites. Most of them belong to the C-type lectin families (including selectins and DC-SIGN [dendritic cell–specific intercellular adhesion molecule-3-grabbing integrin]), but other types of lectins, including human intelectin, fungal adhesin, sea cucumber β-trefoil lectin, and bacterial LecA from Pseudomonas aeruginosa, also are found to have a calcium ion in their binding site (Figure 30.1). LecB, the other lectin from P. aeruginosa, requires the presence of two closely located calcium ions. Calcium ions contribute to the specificity of lectins by selecting for precise stereochemistries of hydroxyl groups; the two calcium ions of LecB, for example, only coordinate monosaccharides with two equatorial and one axial hydroxyl group as present in “fuco” and “manno” configurations. The ions also play a role in enhanced affinity through delocalization of charge as evaluated by quantum chemical calculations, and through compensation for binding entropy losses by releasing strongly coordinated water molecules.

FIGURE 30.1.
Graphical representation of six different calcium-dependent carbohydrate-binding sites found in crystal structures of lectins. (A) Human MPB-A complexed with mannoside (1KWU), (B) Pseudomonas aeruginosa LecA complexed with galactose (1OKO), (C) sea cucumber (more...)
Folding and Oligomerization Facilitate Binding to Cell Surfaces
Lectin structures adopt a limited number of folds (Figure 30.2). Among them, there is a strong predominance of β-sheet-containing domains, such as β-sandwich, β-prism, β-trefoil, or β-propeller. The β-sandwich fold, which is an assembly of two β-sheets, characterizes a large family with different structures that vary in size and localization of binding sites. For example, fimbrial adhesins are very different from galectins in that they use a site near the edge of a sheet as opposed to the concave surface of a sheet. Some structural convergence is nevertheless observed. Intracellular animal lectins, which are involved in the quality control of glycoprotein synthesis, share the same protein fold with legume lectins.

FIGURE 30.2.
(A) Distribution of the lectins with structures available in the 3D-Lectin database as a function of fold family. (B) Graphical representation of the convergent β-propeller folds for lectins. The polypeptide chains are represented as ribbons and (more...)
Convergence is also observed for the β-propeller fold which is a circular arrangement of small β-sandwiches, called blades. Structures with five, six, or seven blades have been observed for lectins. With the exception of bacterial and fungal fucose-binding six-blade β-propellers which are evolutionary related, these structures do not present sequence similarities. However, they share the same global shape allowing for the presentation of all binding sites on the same side of the “donut,” providing for very efficient multivalent binding to glycoconjugates on cell surfaces. This multivalent effect results in high avidity: PVL from the fungus Psathyrella velutina has an affinity of only 100 µm for GlcNAc at each binding site but an apparent avidity of 10 nm for GlcNAc presented on chips. This high avidity makes PVL an excellent tool for identifying tumor cells presenting truncated glycans with exposed GlcNAc.
NUCLEAR MAGNETIC RESONANCE
NMR can provide de novo high-resolution structures of proteins and glycan–protein complexes. It can also provide dynamic information when parts of bound glycans retain some of the mobility displayed in solution. However, NMR-based structure determination usually requires uniform isotopic labeling with magnetic nuclei such as 13C and 15N, to complement data from the highly abundant nucleus, 1H. Isotopic labeling can be accomplished when proteins can be expressed in bacterial hosts, but even then application is largely restricted to proteins of <20 kDa, or of <40 kDa when perdeuteration can be used to improve resolution. The need for uniform isotopic labeling excludes application to many additional proteins of interest. In particular, production of glycoproteins is typically attempted only when expression in eukaryotic hosts is possible, or glycosylation machinery is introduced into a bacterial expression host. Hence, only a few complete structures of glycoproteins with native glycosylation have been produced by NMR methods. However, NMR has fewer restrictions when it builds on protein structures available from X-ray crystallography or computational modeling, and capitalizes on its ability to focus on data involving actual glycan–protein interaction sites. We illustrate this potential in the following sections.
Chemical-Shift Mapping of Protein-Binding Sites for Glycans
The initial step on the route to produce a three-dimensional structure of a protein by NMR methods is usually the assignment of backbone resonances, including the proton and nitrogen resonances of all amide 1H-15N pairs. This step is quite robust and can be accomplished in much less time, and on much larger targets, than a complete structure determination. These assignments are based on a series of multi-dimensional experiments that correlate chemical shifts of directly bonded nuclear pairs. Among these is the two-dimensional 1H-15N heteronuclear single quantum coherence (HSQC) experiment, which correlates an amide 1H-15N pair through the appearance of a cross peak at the chemical shifts of the amide proton and nitrogen of a particular protein residue. Once cross peaks in this experiment are assigned, changes in chemical shift on addition of a glycan ligand can be used to identify a binding site. These changes often arise from small perturbations in residue geometry rather than a direct effect of the ligand on chemical shift, but the effects are usually sufficiently localized to identify the binding site. Figure 30.3 shows an example of changes occurring on the interaction of a hexamer of chondroitin sulfate (CS), sulfated at the O4 position of each GalNAc residue (Chapters 3 and 17). There are actually two types of perturbations observed; gradual changes in chemical shift as ligand is added (arrows in Figure 30.3A) and the disappearance of one peak while another appears (ellipses in Figure 30.3A). These correspond to fast exchange on and off a weak binding site and slow exchange on and off a strong binding site, respectively. Perturbed residues can be mapped onto an existing structure of the protein as shown in Figure 30.3B for the strong binding site. As with many complexes involving a sulfated GAG, positively charged residues are involved; in this case histidine residues and a lysine residue are among those showing chemical shift changes. The advantage of these experiments is that a range of ligands can be examined, regardless of whether cocrystallization with a protein can be accomplished. A limitation is that the backbone resonances of the protein need to be assigned first.

FIGURE 30.3.
Chemical shift mapping of slow and fast exchange binding sites for a 4-sulfated chondroitin sulfate (CS) hexamer on the Link module of TSG6. (A) Cross peaks from spectra with increasing amounts of hexamer are superimposed. Those from residues experiencing (more...)
Identification of Ligand Interaction Surfaces and Bound Ligand Geometry
NMR also offers the potential for characterization of the parts of a ligand that make contact with a protein and the geometry the ligand adopts on binding to a protein's surface. In both cases, the characterization stems from transfer of magnetization from one NMR active spin to another NMR active spin (usually protons) in a distance dependent manner. In the case of bound ligand geometry, the experiment relies on a transferred nuclear Overhauser effect (trNOE). The basis is the same as for the NOE that is used in protein structure determination by NMR; however, only the ligand spectrum is observed. Measurements are usually made from cross peaks in two-dimensional experiments similar to the HSQC experiment mentioned above, except that both dimensions are proton chemical shift, and cross peaks have intensities dependent on the inverse sixth power of the distance between proton pairs (1/r6) rather than direct bonding. An average over both bound and free ligands is observed, but contributions are heavily weighted by those coming from the ligand in a complex because of scaling in proportion to molecular weight. This makes it possible to conduct trNOE experiments with a large excess of ligand (>10:1) and very little protein. Also, there is no requirement for isotopic labeling of either ligand or protein, and having a high-molecular-weight complex is an advantage. The geometry of the bound ligand is derived primarily from distances measured between protons that fall on opposite sides of a glycosidic bond. This distance then restrains glycosidic torsion angles accessible to structural models. Although there are many cases in which the bound geometry is similar to that of the dominant conformer found in solution, there are cases in which the geometry differs. Here, trNOE experiments offer unique insight that can guide synthesis of competitive inhibitors.
Transfer of magnetization from protons on a protein to protons on a ligand in an NOE-like fashion can also provide information on the parts of a ligand in contact with amino acids in a protein's binding pocket (the ligand's binding epitope). In some cases, NOEs between a ligand proton and a specific amino acid proton can be observed, but this requires work with near-equimolar concentrations of ligand and protein, as well as full resonance assignment for both the ligand and the protein. A far more widely applied experiment sacrifices knowledge about specific protons on the protein for an ability to work with very large unlabeled and unassigned proteins, again at ratios of ligand to protein approaching 100:1. This experiment is called a saturation transfer difference (STD) experiment. It relies on the fact the magnetization transfer between protons in large proteins is so efficient that it makes little difference where a change in magnetization is initiated; it can be from saturation of a methyl proton having a resonance a one extreme of the spectrum (upfield), or an aromatic proton having a resonance at the other extreme (downfield). The saturation effect eventually diffuses to a ligand proton close to the protein surface and the resonance of this proton is reduced in intensity. Data are collected as a difference between one-dimensional proton spectra with and without saturation in the extremes of the protein spectrum. The resulting difference spectrum is dominated by resonances from the ligand that have contact with the protein.
Figure 30.4 shows an example that probes the interaction between a complex N-glycan (Chapters 3 and 9) and an HIV broadly neutralizing antibody. These antibodies, specifically interact with surface glycans of HIV and are effective in inhibiting binding of the virus to target cells. Hence, there has been significant interest in exactly which glycans are recognized. Antibodies are large glycosylated proteins that are not usually amenable to NMR investigation by isotope-dependent methods, but STD methods are applicable. The example uses a sample 20 µm in protein (Fab fragment) and 2 mm in glycan. Normal and STD spectra are superimposed to show the enhancement of resonances which include some that come specifically from the Neu5Ac residues (Sia) on the termini of the glycan branches. STD investigations have been conducted on some other very large and complex systems including receptors embedded in membrane fragments, whole cells, and viruses.

FIGURE 30.4.
Binding epitope identification in a complex-type glycan bound to the HIV-1 neutralizing antibody PG16 using saturation transfer difference (STD) information. (Reproduced from Bewley CA, Shahzad-ul-Hussan S. 2013. Biopolymers 99: 796−806, with (more...)
The above provides a glimpse of NMR experiments that can be used to investigate protein–glycan interactions. There are many others that take advantage of additional properties such as differences in translational diffusion constants and specific interactions with water molecules. Many of these have been adopted as screening methods used in fragment based drug discovery programs. Information about these is available in Further Reading.
COMPUTATIONAL MODELING
Experimental structural information obtained by crystallographic and NMR methods have clearly been of value in building an understanding of the molecular interactions that lead to glycan recognition by proteins. However, systems in which interactions are of interest far outnumber the cases in which these methods can be applied. Most crystal structures contain either small ligands or yield useful electron densities for only parts of larger ligands. NMR methods although giving detailed information on bound ligand geometries, frequently give only qualitative information on parts of ligands or protein that are in intimate contact with each other. Both methods require substantial effort, particularly in preparing samples for investigation. A particular problem for glycans of interest is that they are often complex molecules that are difficult to prepare in highly pure forms, or in the quantities needed for experimental investigation. There are also functionally important dynamic processes (e.g., enzymatic conversions of glycan substrates to product and transport of glycans) that are not well represented by static, thermodynamically stable structures. Computational methods can extend analysis into these less accessible regions of structural investigation.
Computational Methods
Computational contributions to our understanding of glycan properties have a long history, beginning with a very fundamental understanding of factors influencing anomeric configuration and glycosidic torsion angles. These glycan specific factors, such as the anomeric effect and the exo-anomeric effect, are described more thoroughly in Chapters 2, 3, and 50. When protein–glycan interactions are of interest, the situation becomes more complex with hydrogen bonding, van der Waals interactions, and electrostatic interactions between glycan and various amino acids becoming important. For very limited sets of atoms, it is possible to pursue an understanding of interactions using advanced quantum mechanical (QM) methods, but for larger systems other approaches based on semiempirical “force fields” are used, such as molecular mechanics (MM) and molecular dynamics (MD).
Empirical “force fields” used in MM and MD modules of packages such as Amber, CHARMM, and GROMOS are typically represented in terms of bond, bond angle, torsion angle, van der Waals, and electrostatic contributions to a molecular energy. Parameters in functions representing each of these terms have been optimized to reproduce QM as well as a selection of thermodynamic and spectroscopic data. Initially, these force fields were developed for proteins alone, so did not include contributions such as the anomeric and exo-anomeric effects found in glycans. Subsequently, force fields explicitly designed to represent the energetics of glycans have been developed for use with these packages (e.g., the GLYCAM force field that is widely used with Amber). There still are challenges in simulating molecular interactions with these packages, among them perfecting models for solvent and accurately representing electrostatic interactions. These issues are very important for glycans, which are rich in hydroxyl groups that act as both hydrogen bond donors and acceptors in their interactions with water. Some glycans (e.g., GAGs) are highly charged, having both carboxylate groups and sulfate groups that interact strongly with positively charged amino acids in proteins and with water. Early simulations were performed with implicit solvent models based on dielectric behavior. Given the recent improvements in computational capabilities, trends are toward the use of explicit solvent models, such as TIP3P and TIP5P.
MM uses terms in the force fields to derive potential energy contributions that can be summed to generate a potential energy for any fixed conformation. In principle, these conformations can be sampled using various search algorithms to generate an ensemble of possible conformations from which thermodynamic parameters can be extracted. More frequently, MD, which uses the forces directly in Newton's second law of motion, is used to simulate movement of all atoms and to generate an ensemble of conformations and orientations that can be reached over times accessible to simulation (nsec to msec depending on the size of the system and efficiency of the computational platform). One advantage of MD is that certain important motional properties, such as the time for diffusion through a channel or the time needed for a conformational transition, can be modeled. One must remember, however, that force fields are meant to represent molecules near energy minima of a conformational surface and are not likely to accurately represent the height of larger barriers separating different conformational states and certainly cannot represent changes in bonding that occur in a chemical reaction.
The actual characterization of how a ligand (a glycan in our case) interacts with a protein involves not just the conformational energetics of the free glycan, but also the conformational energetics of amino acid residues involved in the binding site and the energetics of the glycan–protein interaction. In some cases, there may be relatively little information on where the binding site on a protein is, so the characterization involves locating the best binding site, finding the best conformation for the ligand in the bound state, and finding the best conformations for the parts of the protein involved in binding. The whole process is referred to as “docking” a ligand onto the protein surface. Most docking programs (e.g., Dock, AutoDock, AutoDock Vina, and Glide) are designed to make the initial search for a site very efficient. To do this, they break the process into stages beginning with a rigid-body docking step that is designed to identify the best docking site and best initial “poses” for the ligand. Force fields are often simplified or interaction energies precalculated on a gird to speed the process. Rigid-body docking generally works well for many small drug-like molecules. Also, in many situations, there is a crystal structure of the protein with a native ligand in the binding site, mitigating the problem of optimizing side chain conformations. For glycans, the situation is more complicated; the ligands are often flexible and protein structures with a native glycan in a binding site are often lacking.
In molecular docking, the objective is not to generate a single-bound structure in the first stage but hundreds of “poses” that can be scored and ranked so that a subset can be selected for subsequent stages. Scoring functions are variable, but usually include some sort of interaction energy as part of the score. Subsequent phases typically allow increased flexibility of side-chains and finally an MD refinement of poses, often in explicit water. Final scoring or ranking of poses by energy, even when performed with force fields used in MD programs, seldom leads to a single clear solution, and it has become common to filter poses with additional experimental information such as binding epitopes from STD NMR experiments, or interactions with residues that have been identified as important in mutational studies.
Some docking programs are emerging (e.g., HADDOCK) that make use of experimental data in earlier stages to guide the selection of initial poses as well as maintain known preferences for glycan conformations or specific ligand–protein contacts. Some of the contributions to understanding of glycan–protein interactions that have come from docking exercises, as well as more advanced applications that merge QM with MM or MD are described in more detail in the following sections.
Docking of Heparan Sulfate Oligomers
Heparan sulfate (HS) chains, synthesized initially as a repeating disaccharide of glucuronic acid (GlcA) and N-acetylglucosamine (GlcNAc), and modified by sulfation and epimerization of some GlcA residues to iduronic acid (IdoA), are known to interact with a number of growth factors, receptors, and chemokines (Chapters 17 and 38). Despite the interest in the roles of these interactions in cell migration and differentiation, there are relatively few experimental structures depicting interactions with HS fragments larger than tetra-saccharides. Notable exceptions are the structures of fibroblast growth factor (FGF) in complex with heparin oligosaccharides or their mimics (Chapter 38). Although crystal structures for the protein components of many other systems exist, suitable crystals are less apt to form in the presence of HS oligomers. Also, it is difficult to obtain homogeneous preparations of oligomers larger than tetramers because of the variable sulfation patterns and variable conversion of GlcA to IdoA.
The dearth of experimental structural information has led to the use of computational modeling to predict structures for many of these complexes. Specific sulfation patterns and IdoA substitution can be performed with ease. Yet, the applications of modeling methods to these systems are far from trivial because of the flexibility of the HS chains and the ionic character of interactions that dominate their energetics. Not only are the glycosidic angles variable in HS chains, but the IdoA rings also sample several conformations including a chair, 1C4, and a skew-boat conformer, 2S0. Moreover, orientations of the sulfate groups are variable as are the side-chains of the lysine and arginine residues with which they tend to interact. Nevertheless, applications are made, and a discussion of one of these seems appropriate.
The chemokine CXCL12α (also called SDF1) is a dimeric protein essential for the homing of hematopoietic stem cells to fetal bone marrow; it also plays a role in related developmental processes. Like many other chemokines, it binds to HS in a way that modulates its chemotactic signaling, and there is much speculation as to whether it binds to a segment of HS with a particular sulfation or IdoA/GlcA pattern. Molecular docking methods applied to this system illustrate well the usage of these studies.
This particular study used AutoDock 4 followed by an MD refinement of top scoring poses. These hybrid methods have become common place, combining the efficiency of docking with the ability to refine local structure for better energetics. The initial protein structure in this case came from a dimer cocrystallized with a simple heparin disaccharide (2NWG). The initial geometry for an HS hexasaccharide (HS6) was taken from an NMR study. HS6 was fully sulfated at the nitrogen and 6-oxygen of GlcN and the 2-oxygen of IdoA rings that were held in the 2S0 conformation. Atomic charges for the residues in HS6 were set to match QM electrostatic potentials. The docking began with a semirigid simulated annealing phase in which the ligand is translated and rotated in steps toward the protein surface, but with additional simulated annealing (a short MD run with a programmed temperature variation) at each step, allowing the sulfate and hydroxyl groups of the ligand to move. The docked structures were scored with default parameters that mimic a free energy of binding. The best docked complexes were immersed in a TIP3P water box and subjected to 20 ns of constant temperature MD. For the MD steps, the Amber routine NAMD was used with standard Amber 99SB force field parameters for the protein and GLYCAM06 parameters for the glycan. Final scoring was performed by extracting coordinates for the complex and calculating free energies of binding in implicit solvent with a MM/PBSA procedure.
The resulting structures of HS6 from five selected MD runs are shown superimposed on the protein structure from 2NWG in Figure 30.5. The structures are not identical but lie along the same wide groove at the center of the dimer. Amino acid residue contacts found consistently in the simulations are indicated on the figure. The dominant residues in contact are largely positively charged residues (K1, K24, H25, K27, R41). The actual contacts with HS vary with the side chains adopting different conformations and the HS rolling somewhat in the groove, but there is experimental evidence to support participation of these residues. Further examination of these models may well identify substitutions on HS (deleting certain sulfation sites) that may suggest distinct patterns that would be more tightly bound by CXCL12α.

FIGURE 30.5.
Docking of a heparan sulfate (HS) hexamer to the chemokine CXCL12α. (Reproduced from Sapay N, et al. 2011. Glycobiology 21: 1181−1193, with permission from Oxford University Press.)
Docking of Enzyme Substrates
A large number of enzymes are involved in the synthesis and degradation of glycans (more than 300 human enzymes). Their relative activities, combined with cellular location, are essential to the proper balance of these processes and any alteration, including genetic mutation, can lead to disease in humans. Pathogens also depend on similar processes and understanding such mechanisms can facilitate the design of selective inhibitors of pathogen enzymes. This is another area where molecular docking can play a role. Structural studies of glycan–protein complexes usually require a stable system, not one that would continually convert substrates to products. Moreover, selective inhibitors are often modeled on transition states that are inherently high in energy and low in population for any system at equilibrium.
A recent example in which modeling has played a role involves the glycosyltranferase, ST6Gal1. This is the enzyme that adds a sialic acid (typically Neu5Ac) to the galactose terminated branches of N-glycans by transferring Neu5Ac from its nucleotide-sugar donor, CMP-Neu5Ac to an acceptor terminated with a Galβ1-4GlcNAc moiety (Chapter 6). The production of crystal structures of ST6Gal1, from both human and rat, opens the possibility of modeling at least a pretransition complex with both donor and acceptor. For the study discussed here, the crystal structure of the rat enzyme that contained neither donor nor acceptor (4MPS) was used as a starting point. The CMP-Neu5Ac was modeled into the active site based on the inactive donor analog in the crystal structure of the CstII protein (1RO7), which has less than a 20% sequence identity overall, but a much higher identity in the part of the active site that contains the donor. An initial structure for the minimal acceptor, Galβ1-4GlcNAc, was generated using the GLYCAM WebTool, but glycosidic bonds and hydroxyl groups were allowed to rotate during docking. Docking used the program AutoDock Vina which is similar to AutoDock in file format and overall strategy, but is much more efficient because of parallelism and improvements in the optimization routines, and it has improved scoring functions. As in the previous example, an additional MD step in explicit TIP3P water was used to refine the top ranked docked structure containing protein, donor, and acceptor. Here, MD routines in Amber12 were used with the FF99SB, GAFF, and GLYCAM06 force field parameters for the protein, CMP-Neu5Ac, and Galβ1-4GlcNAc, respectively. MD production runs of 100 ns were generated and interaction energies were analyzed using a MM/GBSAS routine.
Although the positions of donor and amino acid residues near the donor were modeled to be quite similar to those seen in other transferases, the docking/MD procedure provides a unique view of a possible acceptor position and its interactions. Most of the interaction energy holding the acceptor in place comes from interactions with the galactose ring which is well positioned to allow nucleophilic attack on the anomeric carbon of the nucleotide activated Neu5Ac. This energy results from hydrophobic stacking of Tyr-366 with the nonpolar face of the pyranose ring and a network of hydrogen bonds between Asp-271, Asn-230, His-367, and Gln-232 of the protein and O2, O3, O4, and O6 hydroxyl groups of Gal. The position of the GlcNAc is more variable, but does contribute to binding energy. The position and interactions among protein, donor, and acceptor are depicted in Figure 30.6.

FIGURE 30.6.
Interactions between the donor (CMP-Neu5Ac), acceptor (GlcNAcβ1-4Gal), and protein residues in the active site of ST6Gal1. (Reproduced, with permission, from Meng L, et al. 2013. J Biol Chem 288: 34680−34698.)
Although the O6 of Gal and the C2 of Neu5Ac, which will eventually form a bond, are oriented properly and a catalytic H367 is in position to facilitate removal of the 6-hydroxyl proton, the distance between Gal-O6 and Neu5Ac-C2 is still long and the Neu5Ac ring shows no significant distortion from a chair conformation. MD force fields do not allow this type of distortion. QM/MM methods do allow calculations that can predict energies along a minimum energy path between a pretransition geometry (as depicted here) and products. The maximum energy along this reaction path corresponds to the transition state which would be represented in this case as a boat structure for the Neu5Ac ring with a partial positive charge on the C2 carbon, a lengthened C2-O2 bond, and a much shortened C2-O1-Gal distance. A calculation using these methods would presumably identify specific protein residues involved in stabilizing this transition state.
FUTURE PROSPECTS
Structural biology is an evolving area of science both in terms of methodology and questions to be answered. The principle methodologies discussed here are each evolving: crystallographic methods using new X-ray sources such as X-ray lasers are allowing use of microcrystals in cases in which larger crystals fail to grow. Hyperpolarization methods are reducing the sensitivity limitations of NMR, particularly for applications to amorphous solids, including fibrils and membrane fragments. Finally, advances in computational technology are allowing simulation of ever larger systems. At the same time, structural targets are shifting from detailed characterization of single proteins and protein–glycan complexes to larger scale assemblies that cooperate to elicit a functional response. Improvements in methodology have the potential to provide near atomic resolution for systems in some of these more complex environments. SAXS, for example, is increasingly used to characterize complexes. Cryo-EM single particle methods are approaching X-ray resolution for complexes such as a β-glycosidase with a bound inhibitor, and cryo-EM tomography methods may well extend observation to cell surfaces. This is a promising situation for improved understanding of glycan function in biological systems.
ACKNOWLEDGMENTS
The authors appreciate helpful comments and suggestions from Steve M. Fernandes, Jason W. Labonte, Vered Padler-Karavani, and Tong Zhu.
FURTHER READING
- Bewley CA, Shahzad-ul-Hussan S. 2013. Characterizing carbohydrate–protein interactions by nuclear magnetic resonance spectroscopy. Biopolymers 99: 796–806. [PMC free article: PMC3820370] [PubMed: 23784792]
- Grant OC, Woods RJ. 2014. Recent advances in employing molecular modelling to determine the specificity of glycan-binding proteins. Curr Opin Struct Biol 28: 47–55. [PMC free article: PMC4252743] [PubMed: 25108191]
- Perez S, Tvaroska I. 2014. Carbohydrate–protein interactions: Molecular modeling insights. In Advances in carbohydrate chemistry and biochemistry (ed. Baker DA, Horton D, editors. ), Vol. 71, pp. 9–136. Elsevier, Amsterdam. [PubMed: 25480504]
- Bartesaghi A, Merk A, Banerjee S, Matthies D, Wu X, Milne JLS, Subramaniam S. 2015. 2.2 Å resolution cryo-EM structure of β-galactosidase in complex with a cell-permeant inhibitor. Science 348: 1147–1151. [PMC free article: PMC6512338] [PubMed: 25953817]
- Pérez S, Sarkar A, Rivet A, Breton C, Imberty A. 2015. Glyco3D: A portal for structural glycosciences. Meth Mol Biol 1273: 241–258. [PubMed: 25753716]
- Pomin VH, Mulloy B. 2015. Current structural biology of the heparin interactome. Curr Opin Struct Biol 34: 17–25. [PubMed: 26038285]
- Glaeser RM. 2017. How good can cryo-EM become? Nat Methods 13: 28–32. [PubMed: 26716559]
- Review Structural Biology of Glycan Recognition.[Essentials of Glycobiology. 2022]Review Structural Biology of Glycan Recognition.Angulo J, Zimmer J, Imberty A, Prestegard JH. Essentials of Glycobiology. 2022
- Review Biophysical Analyses for Probing Glycan-Protein Interactions.[Adv Exp Med Biol. 2018]Review Biophysical Analyses for Probing Glycan-Protein Interactions.Nagae M, Yamaguchi Y. Adv Exp Med Biol. 2018; 1104:119-147.
- CHARMM-GUI Glycan Modeler for modeling and simulation of carbohydrates and glycoconjugates.[Glycobiology. 2019]CHARMM-GUI Glycan Modeler for modeling and simulation of carbohydrates and glycoconjugates.Park SJ, Lee J, Qi Y, Kern NR, Lee HS, Jo S, Joung I, Joo K, Lee J, Im W. Glycobiology. 2019 Apr 1; 29(4):320-331.
- Review Integrated structural biology to unravel molecular mechanisms of protein-RNA recognition.[Methods. 2017]Review Integrated structural biology to unravel molecular mechanisms of protein-RNA recognition.Schlundt A, Tants JN, Sattler M. Methods. 2017 Apr 15; 118-119:119-136. Epub 2017 Mar 16.
- 3D Computational Modeling of Proteins Using Sparse Paramagnetic NMR Data.[Methods Mol Biol. 2017]3D Computational Modeling of Proteins Using Sparse Paramagnetic NMR Data.Pilla KB, Otting G, Huber T. Methods Mol Biol. 2017; 1526:3-21.
- Structural Biology of Glycan Recognition - Essentials of GlycobiologyStructural Biology of Glycan Recognition - Essentials of Glycobiology
Your browsing activity is empty.
Activity recording is turned off.
See more...