This book is distributed under the terms of the Creative Commons Attribution-NonCommercial-NoDerivs License which permits noncommercial use and distribution provided the original author(s) and source are credited. (See https://creativecommons.org/licenses/by-nc-nd/4.0/
NCBI Bookshelf. A service of the National Library of Medicine, National Institutes of Health.
Structured Abstract
Background:
We implemented the electronic-Asthma Tracker (e-AT) at 11 clinics to support a new ambulatory care model for children with asthma. The e-AT is a web and mobile web, user-friendly tool designed to engage parents in monitoring their child's asthma, with real-time alerts and recommendations to promote timely care.
Objectives
- (1a) Compare outcomes between clinics randomized to standard vs intensive e-AT intervention. (We initially planned a cluster randomization but modified the study design per stakeholders' request, allowing all participants to receive the e-AT).
- (1b) Use nonrandomized comparisons to determine the e-AT effectiveness vs usual care.
- (2) Assess whether the e-AT effect on child outcomes varies across parent characteristics.
- (3) Determine factors associated with sustained parent participation in self-management.
Methods:
Participants 2 to 18 years old were enrolled between January 1, 2014, and December 31, 2015, with a 1-year follow-up. e-AT (standard) implementation was staggered, with 6 clinics starting early (January 1, 2014) and 5 late (April 1, 2014), with 3 months' delay. On September 17, 2014, 5 clinics (3 early and 2 late) were randomized to continue with the standard e-AT and 6 clinics (3 early and 3 late) to transition to intensive e-AT. We used prespecified analyses, including randomized comparisons between clinics on the standard (n = 261) vs intensive (n = 57) e-AT. Nonrandomized comparisons included the following:
- (1) longitudinal change within participants (n = 318);
- (2) emergency department (ED)/hospital admissions/oral steroid use (n = 325) in the year following vs before patient's enrollment (pre-post);
- (3) ED/hospital admissions/oral steroid use in patients in early (n = 198) vs late (n = 129) starting clinics (clinic-level) with the 3-month delay (or usual care period);
- (4) ED/hospital admissions/oral steroid use in patients (patient-level) who started the e-AT in the first year (January 2014-December 2014) or early starting patients (n = 234), during the 1-year period after e-AT start dates vs those starting the e-AT in the second year (January 2015-December 2015) or late starting patients (n = 91), during the 1-year period before e-AT start dates (usual care); and
- (5) ED/hospital admissions/oral steroid use in e-AT participants (n = 325) vs nonrandomized matched controls from clinics that did not use e-AT (n = 603).
Results:
We enrolled 325 children and parents. Average e-AT use adherence was 65% at 12 months. In randomized analyses (analysis 1), we found no significant differences in outcomes of intensive vs standard e-AT. When compared with their own baseline (analysis 2), e-AT participants had (P < .001) increased quality of life (QOL), reduced missed school/workdays at 3, 6 and 12 months, and increased asthma control at quarters 1 to 4. Parent satisfaction remained high. ED/hospital admissions and oral steroid use (analysis 3) were reduced (P = .024 and P = .003, respectively) in the year post vs year before patient's enrollment. We found only 2 patients in early starting clinics with ED/hospital admissions and 4 who used oral steroids, during the 3-month delayed start of late-starting clinics, too few for statistical analysis (analysis 4). We found no significant differences in ED/hospital admissions (P = .183) and oral steroid use (P = .937) between early starting vs late starting patients (analysis 5). Relative to matched controls from clinics that did not implement e-AT (analysis 6), e-AT participants had reduced ED/hospital admissions (P = .004) and oral steroid use (P = .019). Finally, having Medicaid insurance was associated with higher ED/hospital admission risk, with some QOL reductions on more educated but infrequent e-AT user parents. Barriers and facilitators of e-AT use were identified.
Conclusions:
The e-AT was effective in improving asthma outcomes. Dissemination can improve asthma care broadly.
Limitations:
Resulted from study design change, replacing a cluster randomization design and relying on nonrandomized comparisons to assess the e-AT effectiveness relative to usual care.
Background
Pediatric asthma is associated with frequent exacerbations (attacks) and a significant impact on a child and family's quality of life (QOL) and health care use.1 In 2009, an estimated 7.1 million children younger than 18 years of age had asthma.1 Asthma is also one of the most common causes of school absenteeism and missed work for parents.2 The annual economic impact of pediatric asthma in the United States is estimated at $20.7 billion in total costs.3
Despite guidelines, asthma remains a poorly controlled disease.4 Poor control leads to increased risk for asthma attacks, poor QOL, and frequent acute health care use.5 To improve control and reduce asthma attacks, asthma guidelines recommend patient self-management.6-9 Asthma self-management support interventions have focused mostly on adults10-16 and have been associated with improved outcomes.13,14,16-20 However, self-management support interventions in children remain rare and are challenging to achieve.7
The few studied self-management support interventions in children have limitations. They have (1) been complex and required a significant amount of staff time, creating physician practice operational challenges21; (2) rarely included regular monitoring with timely feedback; (3) not effectively engaged parents and primary care providers (PCPs); and (4) either not evaluated the effect on asthma outcomes22,23 or reported mixed results with no effect 21,24-27 or little effect on outcomes.28,29 Also, children are less able to verbalize complaints.30 Their subtle complaints often go unrecognized by parents,31 who often are unaware of early signs of deterioration31,32 and lack effective tools to guide them in recognizing and acting on warning signs of deterioration.33
Several validated tools exist to assess asthma control and support self-management. Spirometry is limited by availability, cost, and effort required by younger children to perform the test.7 Peak flow (PF)34 is effort-dependent and is not always reproducible over time.7,35-37 Symptom-based questionnaires like the Asthma Control Questionnaire (ACQ) or Asthma Control Test (ACT) have equal validity with PF,38,39 but with several limitations.40-42 Our team addressed limitations of symptom-based questionnaires and was the first to validate the ACT as a weekly self-management tool (within Asthma Tracker) for use by children or parent proxies.43,44
This project was built on our previous efforts to transform and improve asthma care, beginning with the development and validation of the paper-Asthma Tracker (paper-AT) and moving to the design, validation, and pilot testing of the electronic-Asthma Tracker (e-AT).43,45,46 The paper-AT is a user-friendly tool we developed in 2010 to engage parents in their child's asthma self-management, using the ACT questionnaire,47 adapted and validated for weekly assessment of asthma control and augmented with a longitudinal graphical display of weekly ACT scores, and coupled with decision support to guide appropriate and timely responses by parents/children and PCPs to warning signs of asthma control deteriorations. Although the paper-AT tool was successful in improving asthma outcomes in our preliminary study,46 there were limitations. Specifically, there was no real-time feedback for patients/parents, who had to interpret results themselves based on preset recommendations. This approach delayed the time for identifying early signs of asthma control deteriorations, for at least 1 week. There was also a lack of incentive to engage patients/parents in sustained use, particularly if their child's asthma was well controlled. Thus, parents thought a web or mobile phone version would be more useful in engaging them.
With parent stakeholders, we developed the e-AT (http://asthmatracker.utah.edu)43 to address limitations of the paper-AT by providing (1) parents with immediate prompts when their children are doing well and early warnings when an action or a PCP visit is needed, (2) PCPs with objective and real-time patient data to guide appropriate adjustments of asthma therapy, and (3) longitudinal patient data to monitor disease progression. The e-AT can be used by older children with or without parental assistance or by parents of younger children (< 11 years old). In our initial focus group, parents were interested in assessing the e-AT effectiveness and identifying critical barriers associated with sustained e-AT use. Factors associated with sustained use of self-management support interventions are not well known. Knowing these factors would help promote uptake of these interventions.
Our goal was to implement the e-AT at multiple clinics and assess the impact on outcomes. We hypothesized that participants/clinics in the intensive e-AT would have better outcomes. In addition, participants/clinics in the new care model (both standard and intensive e-AT) would have better outcomes relative to usual care. We also hypothesized that child outcomes would be better in parents who were frequent e-AT users with a high health literacy as well as private insurance, but that they would be similar in frequent e-AT users, regardless of parent characteristics. Finally, we hypothesized that sustained parental participation in asthma self-management would be influenced by prespecified factors (Figure 1) guided by the literature and that cross-cutting themes (barriers and facilitators), generalized to other chronic diseases, would be identified across demographic and socioeconomic factors.
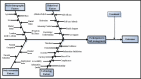
Figure 1
Conceptual Framework of the Relationship Between Factors and Parent Participation.
Participation of Patients and Other Stakeholders in the Design and Conduct of Research and Dissemination
Parent/Stakeholder Engagement in the Current Proposal
We engaged 10 parents of children with asthma in the design and validation of the paper-AT. While developing the current proposal, we worked with 1 parent representative who used the paper-AT for her child. Upon funding, we recruited 5 more parents for a total of 6 parents. We also engaged 2 PCPs to provide insight in facilitating e-AT implementation at clinics. We also involved other stakeholders, including representatives from insurance companies (SelectHealth and Medicaid), the Utah Department of Health (school nurse and asthma program), and the Intermountain Healthcare (IH) system (pediatric and primary care clinical program directors), as well as an external advisory committee of recognized health care leaders. Overall, we engaged 16 parents and 13 stakeholders to solicit their input in designing and testing the paper-AT and e-AT, and in developing the current proposal, including objectives, study design, and outcome measures. Input was received through focus group sessions43 and facilitated discussions.
Methods to Identify/Recruit Parents/Stakeholders
We recruited parent partners from a cohort of parents of children who used the paper-AT. To ensure a good representation in characteristics that could affect participation, we divided parents into 2 groups based on their adherence with weekly use of the paper-AT, and selected 2 frequent and 2 infrequent users. We also solicited referral from PCPs, who helped us enroll 2 more parents. We recruited community stakeholders based on established relationships from previous collaborations.
Engagement Frequency
After funding, we held a 2-day kickoff retreat with all stakeholders. We watched 2 PCORI videos on stakeholder engagement in research and discussed expectations regarding their participation and required trainings (eg, Human Subjects Protection, HIPAA). We discussed the provisional study protocol for additional stakeholder input, reviewing and recording direction regarding study objectives, design, and outcome measures. During the study, we held monthly meetings with clinic care coordinators (CCCs), parents, and the research team, and quarterly meetings with the entire team to discuss progress and issues raised in the study. Other parent engagement activities included (1) developing clinic/participant recruitment materials, (2) participating in monthly research progress conference calls, (3) reviewing performance data and assisting in solving issues, (4) monitoring project goals and providing ongoing support, and (5) conducting site visits to address barriers to study recruitment. The advisory community members were included in the various work phases, providing their input through phone calls or emails, or via face-to-face meetings (2 times per year).
Perceived or Measured Impact of Parent/Stakeholder Engagement
Development of the Research Questions
As stated above during the e-AT pilot test, parents motivated us to develop our study questions focusing on e-AT effectiveness and in identifying barriers to sustained e-AT use.
Study Design and Processes
We originally planned to cluster randomize clinics to the e-AT intervention or usual care and compare outcomes. However, during our kickoff retreat (before we launched the study), our stakeholders strongly opposed withholding the e-AT intervention from control clinics and patients, given our preliminary data showing asthma outcome improvements. (Note: Our team previously pilot tested the e-AT effectiveness in a prospective study of children aged 2-12 enrolled during asthma hospitalization: 210 e-AT users vs 353 nonusers (controls). The e-AT was used weekly for 6 months post-hospital discharge. Overall, e-AT users had longer time to first readmission. Our study also demonstrated a direct dose-effect relationship with frequent e-AT users having fewer emergency department (ED)/hospital admissions than less frequent users).46 Specifically, our stakeholders requested changing the study design to allow all clinics to use the e-AT (see also the “Study Design Changes/Updated Objectives” section). They also requested a change in the enrollment age criteria (from 2-12 to 2-17 years old) to include teenagers, a population with high asthma morbidity and poor adherence to treatment. Finally, they requested changes to a few outcomes (eg, from missed school/workdays to interrupted or missed school/workdays), reflecting the parental reality that their days were often interrupted due to their child's asthma. PCORI staff approved their suggestions, and changes were made before the study started.
Selection of Outcome Measures
Child's QOL, the primary outcome (objective 1), was selected to capture 2 of the most relevant asthma outcomes that parents were concerned about, including nighttime awakenings and activity limitations. Children who are awakened at night due to asthma are often tired during the day and have more difficulty concentrating in school. In addition, parents are affected by productivity reduction due to lost sleep.48 Secondary outcomes reflected parent/stakeholder interest in improving asthma control, satisfaction with care, and avoiding ED/hospital admissions. As stated earlier, our research questions also focused on identifying and addressing barriers to sustained e-AT use. We also included assessment of the e-AT effectiveness across different parent characteristics (objective 2) and critical factors associated with sustained parent participation in asthma self-management (objective 3).
Study Rigor and Quality
Although stakeholder engagement led to the selection of outcome measures that were meaningful to them, changes to the initial design (due to stakeholders' request) to allow the e-AT to be provided to all participants and clinics, reduced the rigor and quality of the study and led to a more complex study design (see the Methods section).
Transparency of the Research Process
We engaged parents and other stakeholders through the entire research process. All major decisions regarding the study process were made with parent partners and stakeholders, often using language understandable to them, leading to increased transparency. We can share upon request the study documentation (eg, study protocol, programming code and data definitions) and deidentified data, so others may reproduce results.
Adoption of Research Evidence Into Practice
Parent stakeholders were eager to facilitate clinic recruitment based on their own experience using the e-AT. They also worked to improve participant's adherence by developing patient recruitment materials and a Facebook page to strengthen parent support and to increase their ability to sustain ongoing use of the e-AT and study participation. Their presence during clinic recruitment and outreach visits increased the study's credibility and motivated clinic participation.
Methods
Setting/Initial Design/Objectives
The study involved 11 primary care clinics, with 9 clinics belonging to the IH system. These clinics are located in Salt Lake City and rural regions in northern and southern Utah. IH is a regional, not-for-profit integrated health care delivery system, with 22 hospitals and 185 clinics and urgent care facilities in Utah and southeastern Idaho,49 providing care to ∼1 680 000 patients, which includes ∼60% of Utah's residents and 85% of Utah's children.50 We enrolled children 2-17 years old with persistent asthma and their parents. The study was initially a prospective, cluster-randomized trial with 480 children (2-12 years old) and parents from 10 clinics to be randomized to the e-AT vs usual care.
Study Design Change/Updated Objectives
We made changes (see details under the “Perceived or Measured Impact of Parent/Stakeholder Engagement” section) to the study design, age criteria, and outcome measures, and 1 clinic was added (making 11 clinics), leading to a new design with staggered implementation of clinics, split in 2 groups, early (started on January 1, 2014) vs late (started on April 1, 2014) starting clinics, with a 3-month delay period between the 2 starting dates. We then cluster-randomized clinics to standard vs intensive e-AT. Figure 2 illustrates the updated study design.
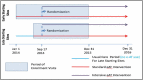
Figure 2
Study Design and Randomization Procedures.
Final objectives included the following:
- Objective 1: To assess the effectiveness of the new ambulatory care model, by comparing outcomes for the (1a) child (QOL, asthma control, and interrupted/missed school days due to asthma); (1b) parent (satisfaction and interrupted/missed workdays due to a child's asthma); (1c) clinic (ED/hospital visits due to asthma), between clinics randomly assigned to the standard vs intensive e-AT intervention; and (1d) use of several nonrandomized comparisons to determine the e-AT effectiveness relative to usual care periods or control groups (see the “Five Nonrandomized Comparisons Between e-AT Interventions and Usual Care” section for details) in which the e-AT was not used.
- Objective 2: In the intervention groups, to assess whether the effect on child outcomes varies across parent characteristics (high vs low health literacy, Medicaid vs private insurance, frequent vs infrequent users).
- Objective 3: In the intervention groups, to determine (demographic, socioeconomic, behavioral, and technology) factors associated with sustained parent participation in asthma self-management (defined as sustained use of the e-AT).
Forming the Study Cohort
We conducted an outreach visit and education at clinics that met the eligibility criteria and showed interest to participate in the study.
Clinic Eligibility
Primary care clinics with (1) capacity (eg, available CCCs and time) and leadership to adopt e-AT, (2) patients <18 years old with persistent asthma, and (3) capacity to accommodate patient enrollment and training about e-AT use.
Not all IH clinics were contacted for participation. Of the 11 clinics contacted and accepted to participate, 9 are part of the IH system. PCPs from the 2 non-IH clinics are familiar with the IH systems and asthma care process model, as they admit and see their patients hospitalized at their reference local IH hospitals.
Clinic Training
Clinics (including PCPs, nurses, CCCs, and other clinical staff) received an introductory presentation about gaps in asthma care, the e-AT application, how it can be implemented in the clinic, how it can guide step therapy, and results of a pilot study. The CCCs also received one-on-one training with the research coordinator on how to enroll, monitor (using the dashboard), respond to alerts, and follow up with patients in collaboration with the patient's PCP.
Participant Eligibility
Inclusion criteria were as follows: (1) children 2 to 17 years old with persistent asthma and their parents (patient's eligibility was verified first using the enterprise data warehouse (EDW), with persistent asthma defined as being on a controller medication, and then from physician documentation in the medical records of persistent asthma (based on NIH classification criteria); (2) English speakers; (3) children who had received asthma care at participating clinics; and (4) families with internet access.
As this was a pragmatic trial in a real clinical environment, no patients were excluded, except those who had previously used the paper-AT.*
Participant Identification/Enrollment
Eligible participants were identified (as described above) by each clinic and approached for enrollment (in standard e-AT) in early (January 1, 2014) or late (April 1, 2014) starting clinics, using 2 processes: (1) phone calls by the clinic CCC, inviting them to the office for enrollment; and (2) direct CCC invitation during clinic visits (asthma or non-asthma related). Clinics were randomized (on September 17, 2014) to standard vs intensive e-AT. Participants who consented were educated about the e-AT using a standardized teaching flipchart, given e-AT access with a temporary username/password to be changed at first login, and asked to use the e-AT weekly for a 1-year period after enrollment. Participant enrollment occurred from January 1, 2014, to December 31, 2015.
Control groups (see the “Five Nonrandomized Comparisons Between e-AT Interventions and Usual Care” section) included (1) late starting clinics (vs early starting clinics), (2) late starting patients (vs early starting patients), and (3) matched controls drawn from 42 nonparticipating clinics within the IH system located in Salt Lake City and Utah County, where randomized clinics are located.
Participant Withdrawal
Participants who elected to withdraw from the study had no data collected after the withdrawal date. Data collected before withdrawal remained in the study database. Research personnel contact information was available to participants during the study to facilitate communication and decision to withdraw.
Clinics did not collect reasons why participants declined enrollment, although they reported that most (>90%) accepted participation. Only 9 patients/families decided to withdraw, for 3 reasons: (1) lack of time (n = 1), (2) misdiagnosis of asthma (n = 1), and (3) because they were no longer interested in the study (n = 7).
Interventions
Comparator Control Intervention/Rationale for Selection
The e-AT was chosen because parents were interested in its effectiveness. We have 2 levels of comparisons: (1) standard vs intensive e-AT and (2) e-AT interventions (standard/intensive) vs nonrandomized usual care. Asthma care at these clinics (e-AT and non-e-AT) is standardized through prior quality improvement projects. (Although the other 2 clinics are from non-IH systems, their PCPs also work at IH hospitals and have been involved with various quality improvement projects implemented by IH to standardize asthma care.) Usual care was directed by national guidelines, with rescue therapy for acute symptoms, controllers (medications for long-term control of asthma symptoms) based on asthma severity/control (step therapy), asthma education, and a written action plan.
Standard e-AT Intervention
The e-AT, web (Figure 3) and mobile web (Figure 4) versions have 4 components: (1) patient portal, (2) survey questionnaire, (3) report generator creating color-coded dynamic run charts (Figure 5), and (4) decision support pop-up messages/recommendations) tied to asthma control scores, displaying green, yellow, and red zones, with personalized feedback (email/text messages tailored to each patient).43,46 The standard e-AT features include (1) automated reminders to sustain use; (2) real-time result graphing; and (3) alerts that are sent to patients/parents (via email/text messages) and the PCP's office (via clinic email and e-AT clinic web-based dashboard) on the patient's deteriorating asthma control; and (4) real-time decision support (pop-up messages recommending appropriate actions) based on color zones: green (continue current care), yellow (follow asthma action plan, and contact PCP if score remains in the yellow zone the following week), and red (contact PCP immediately for evaluation). The dashboard (Figure 6) facilitates patient management by a CCC or PCP, providing real-time access to a patient's asthma control status, poor asthma control alerts, graphs/decision support, adherence to weekly e-AT use, and patient contact methods. Following the alert, the clinic proactively contacts patients/parents to identify and address asthma care issues and adjust treatments if needed using step therapy.35
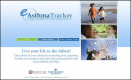
Figure 3
e-AT Web Version.
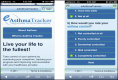
Figure 4
e-AT Mobile Web Version.
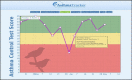
Figure 5
User Interface (Dynamic Run Chart): Standard e-AT Intervention.
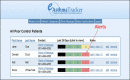
Figure 6
Care Coordinator Dashboard.
Intensive e-AT intervention
Intensive e-AT was developed to increase participant adherence with weekly use through additional motivational features (Figure 7), including a progress bar, which adds 25 points/participant completion of an assessment. At 100 points (∼4 assessments per month), a pop-up message is generated that includes fireworks, a congratulatory message, and a notice that an incentive ($10 gift certificate) is being sent by mail. The incentive system resets to 0 after it reaches 100 points. The second motivational feature is the leader board, which allows participants to see adherence of the 5 best e-AT users, motivating them to increase their own adherence. Standard e-AT users were also sent the gift after 4 assessments per month but did not see the progress bar, leader board, fireworks, or congratulatory message. Yet, the graph about the child's asthma status, recommendations about management, and clinic dashboard were similar in both standard and intensive interventions.
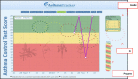
Figure 7
User Interface (Dynamic Run Chart): Intensive e-AT Intervention.
Follow-up
Recruitment and Consent Procedures
Planned recruitment/enrollment was to occur from June 1, 2013, to June 30, 2015, but it was delayed by the study design change and PCORI approval process. We therefore extended recruitment to December 31, 2015 (with follow-up data collection through December 31, 2016) to reach the updated sample size of 315 participant dyads. Eligible participants with persistent asthma (using NIH criteria) were consented (informed consent or parental permission plus assent), allocated to a study arm (early vs late clinics, and standard vs intensive e-AT), and provided asthma education/instructions on how to access and use the e-AT, including a temporary username/password to be modified upon first login, allowing full e-AT access.
Randomization Procedure
Across the 11 enrolled clinics, including 6 early clinics (3 large [>100 potential enrollees] and 3 small [≤100]) and 5 late starting clinics (3 large and 2 small), participants were cluster randomized by computer algorithm on September 17, 2014, to the standard or intensive e-AT intervention (Figure 2).
Study Outcomes
Primary Outcomes
QOL scores were collected using the Integrated Therapeutics Group Child Asthma Short Form (ITG-CASF).51,52 QOL scores range from 1 (poor QOL) to 100 (better QOL).
Secondary Outcomes
Asthma control (scores ranging from 5 [poorest asthma control] to 25 [optimal asthma control]), interrupted/missed school days (number), parent satisfaction (scores reported at 1 = very dissatisfied, 2 = dissatisfied, 3 = neutral, 4 = satisfied, and 5 = very satisfied), parent interrupted/missed workdays (number), oral steroid use (number), and ED/hospital admissions (number).
Data Collection and Sources
At enrollment, parents completed surveys addressing demographic, socioeconomic, and behavioral factors; parent satisfaction; and education level (replaced health literacy). A technology factor survey also included 2 open-ended questions assessing barriers/facilitators of parent participation. Baseline surveys were collected at the time of enrollment on paper, but follow-up surveys were collected online using the Research Electronic Data Capture (REDCap) database. Outcomes data, including ED/hospital admissions—using ICD-9 and ICD-10 codes and oral corticosteroid use, 1 year pre- and 1 year post-e-AT initiation—were collected using the IH EDW,53 an integrated database linking administrative and clinical data from IH hospitals and clinics. The EDW was also used to collect patient demographics (age, gender, race, and insurance) and to create a matched control group. Table 1 lists data elements, validated survey instruments and references, data sources, and frequency of data collection.
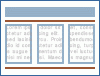
Table 1
Timeline of Data Collection and Survey Instrument and Data Collection Source.
Follow-up and Retention Strategies
We used multiple strategies to increase retention, including obtaining contact information of at least 2 family members at enrollment, providing incentive, mailing thank you cards, and confirming contact information at follow-up assessments. Patients who stopped using the e-AT were contacted by the research coordinator and/or CCC to motivate ongoing adherence or identify and document reasons for withdrawal or loss to follow-up (triggered by ∼3 months of inactivity).
Statistical Analysis
We used SAS 9.4 statistical software (https://www.sas.com/en_us/software/sas9.html) to conduct all analyses. The new design allowed randomized comparisons of 2 levels of e-AT intensity, but not e-AT effectiveness vs usual care. Consequently, we included 2 general categories of analyses:
- Randomized comparisons of standard vs intensive e-AT, comparing QOL and secondary outcomes.
- Four nonrandomized analyses to assess the e-AT effectiveness compared with usual care.
- The first analysis assessed longitudinal change within-patient (both standard and intensive e-AT users) in outcomes (QOL, satisfaction, asthma control, interrupted/missed school days, and interrupted/missed workdays) compared between baseline (before e-AT initiation) and follow-up assessments while using the e-AT.
- The second analysis (early vs late starting clinics) or clinic-level analysis compared oral steroid use and hospital/ED admissions between enrolled participants in clinics that started the e-AT early vs participants in clinics that started the e-AT late (see also the “Comparison of ED/hospital admissions and oral steroid use between patients enrolled…” subsection).
- The third analysis (early vs late starting patients) or patient-level analysis involved both standard and intensive e-AT and compared oral steroid use and ED/hospital admissions between enrolled participants that started the e-AT in the first year vs those that started the e-AT.
- in the second year, during the 1-year period before their e-AT start dates (serving as usual care period) when late-starting participants were not using the e-AT (see also the “Comparison of ED/hospital admissions and oral steroid use 1 year post-e-AT initiation …” subsection).
- The last analysis (limited to ED/hospital admissions and oral steroid use) compared participants in the e-AT (standard and intensive) groups vs nonrandomized matched controls or participants with persistent asthma from clinics that did not participate in the e-AT study.
Original Power Calculation
The original calculation for the primary (QOL) outcome assumed 480 participants would be enrolled, with 400 completing 12 months of follow-up, distributed across clinics, and assumed an SD of 20 points for QOL with an intraclass correlation (ICC) of 0.015 to account for potential clustering of QOL scores by clinics. Under these assumptions, the design would have provided 80% power with a 1-sided α of .05 to detect a difference in the mean QOL score of 6.3 points between the treatment groups, representing a relatively small treatment effect of about 31.5% of 1 SD in QOL score. This calculation was conservative in 3 aspects: (1) the detectable effect of 31.5% of 1 SD was small, (2) the power calculation did not account for added precision resulting from having multiple QOL assessments during the follow-up period, and (3) the power calculation did not account for likely gains in power resulting from statistical adjustment for the baseline QOL score. Thus, while there was considerable uncertainty in the projected ICC, the design left some margin of error in the power calculation that would have mitigated the effects of a smaller-than-projected enrollment.
Updated Power Calculation (June 2016)
The actual number of patients with 6-month follow-up was 52 in the intensive e-AT and 233 in the standard e-AT intervention. Enrollment was substantially imbalanced across sites, with ≥20 patients randomized in 4 sites assigned to the standard and 1 site on the intensive e-AT. Thus, the amount of data to estimate between-site variation in QOL and other outcomes was very limited, making statistical inferences under mixed models problematic. As a result, the primary analysis was modified to be a fixed-effects analysis that would apply statistical inferences specifically to sites included in the study, with the recognition that generalization of the study results beyond sites in this specific trial would have to be based on subject-matter considerations rather than direct statistical inference. Assuming an SD of 20 units, the sample sizes of 233 and 52 patients would provide 80% power with a 1-sided α = .05 to detect a mean difference of 7.65 points (or 38% of 1 SD). If we further assumed the correlation between the baseline QOL score and the mean follow-up QOL score was 0.5 or greater, the minimum detectable effect size was reduced to 6.63 points (or 33% of 1 SD). In spite of the lower-than-planned sample size in the intensive e-AT group, these detectable effects were not substantially changed from the minimum detectable effects (MDEs) we projected in September 2015. This reflects 2 factors. First, the impact of the imbalance between 233 and 52 participants in the 2 groups was not as large as we expected at first glance, and the MDE with 233 in one group and 52 in the other group was just 29% greater than the MDE for an equal allocation of the 285 total patients to the 2 groups. Second, because we were now applying the statistical inferences specifically to the study sites under a fixed model instead of attempting more general inferences under a mixed model, it was no longer necessary to account for the design effect due to the facility-level ICC. In other words, the unbalanced randomization across sites has forced us to “lower the bar” and perform fixed-effects inference instead of using a mixed effects model, but the statistical power to reach these conclusions was not appreciably reduced.
Final Updated Power Calculation for Fixed-Effects Analysis (October 2016)
The actual numbers of patients with at least 12-month follow-up was 49 in the high-intensive e-AT and 214 in the standard e-AT intervention. Enrollment was substantially imbalanced across sites, with ≥20 patients randomized in 4 sites assigned to the standard e-AT and 1 site (with >20 patients) on the intensive e-AT. As noted above, we therefore planned to perform both mixed and fixed-effects analyses. In addition, the analysis plan had to be modified to account for varying follow-up times for each patient after randomization. We assumed a cross-sectional SD of 20 units, and that at least 90% of the 214 and 49 patients in the standard and intensive e-AT groups would have a nonmissing QOL measurement at their final (12-month) planned follow-up QOL, and that baseline covariates would not be substantially imbalanced between clinics randomized to the intensive and standard e-AT. Under these assumptions, when the fixed-effects analysis was used, the study provided 80% power with a 1-sided α = .05 to detect a mean difference of 8.33 points (or 42% of 1 SD). If we further assumed that the correlation between the baseline and the final follow-up QOL score was ≥0.5, the MDE size was reduced to 7.21 points (or 36% of 1 SD).
Objective 1: Assess the Effectiveness of the New Ambulatory Care Model
Descriptive Analyses
We summarized baseline characteristics and outcome measures at each follow-up, overall, and within each arm, using means, medians and ranges for continuous variables, and frequencies for categorical and ordinal variables. The balance of baseline measures across the 2 intervention arms were compared with robust variance estimates to account for clustering by clinic.
Randomized Comparisons Between Standard and Intensive e-AT Interventions (See Figure 2)
Data used
The first QOL and other outcomes obtained at enrollment were used as baseline values, reflecting patient outcomes under usual care (without e-AT exposure). When comparing both interventions, follow-up outcomes were restricted to postrandomization measurements. Measurements after baseline and before randomization were excluded so the analysis represents a true comparison of randomized groups.
Analyses of QOL
We used a linear fixed-effects (LFE) model to estimate the adjusted mean QOL score change at each postrandomization follow-up in the intensive and standard e-AT. Linear contrasts were used to compare mean QOL change at each follow-up between clinics assigned to intensive vs standard e-AT, each clinic weighted by the number of participants contributing to the analysis at each assessment. An adjustment for the length of time between randomization and postrandomization QOL assessments was performed and an unstructured covariance model was used to account for serial correlation.
In addition, we used a linear mixed-effects (LME) model to include a random effect for clinic to account for clinic clustering. An unstructured covariance model was used to account for correlations of model residuals between measurements in the same patient. We based the primary comparison on estimated treatment effect at the final follow-up (12 months). To account in part for the small number of clinics providing substantive information concerning random-effects variance, we used restricted likelihood estimation with Kenward-Roger estimates for degrees of freedom.
Secondary outcomes analyses
We used linear (continuous data) or generalized linear (categorical/count data) fixed- or mixed-effects models (LFE/GLFE or LME/GLME). Due to imbalanced/small patient numbers at some clinics, GLME modeling was limited. We relied on GLFE to compare categorical outcomes or count data between intervention groups. Analyses were also restricted to assessments occurring postrandomization.
- Parent satisfaction. As in QOL, we attempted to fit LME models to provide generalizability inferences beyond the study clinics and LFE models to provide inferences to participating clinics. The models included terms to adjust for the length of time between randomization and postrandomization follow-up assessments.
- Asthma control. Asthma control was analyzed similarly in random patient effects models using ACT scores. We compared the proportion of assessments with good control (score 19-25) vs poor control (scores 5-18).
- ED/hospital admissions and oral steroid use. The GLFE models, adjusting for baseline imbalances, for these outcomes used negative binomial distribution, with offsets for postrandomization follow-up time and adjustment terms to account for proportion of assessments occurring postrandomization, but fitted separate mean event rates for each clinic. The treatment effects were estimated by comparing weighted averages of the estimated event rates between clinics assigned to intensive vs standard e-AT. GLME models were also fitted for negative binomial outcomes with logarithmic link functions and random clinic effects to outcome events occurring postrandomization. Robust sandwich estimates of SEs were used for statistical inference.
- Interrupted or missed school and workdays. We compared proportion of school and workdays interrupted or missed during 3-month intervals before the final 12-month follow-up assessment and used the negative binomial distribution. Analyses used GLME and GLFE models as in ED/hospitalizations and oral steroid use, except events were ascertained only during the 3-month period before the final 12-month assessment, and the offset term was defined as the number of potential school or workdays for which absence was evaluated.
Five Nonrandomized Comparisons Between e-AT Interventions and Usual Care
Longitudinal within-participant analyses
We determined mean change (from baseline to follow-up assessments) of QOL, asthma control, satisfaction, and rates of interrupted/missed school/workdays.
- QOL. We fitted an LFE repeated-measures model for repeated QOL measurements, with study clinic, time (as a categorical factor), and clinic by time interaction terms used in the model as fixed effects, with a common unstructured covariance matrix to account for correlations in QOL measurements in the same patient. Linear contrasts were used to estimate mean change in QOL across all clinics (assigned to both intensive and standard e-AT) between the baseline assessment and each of the 3 follow-up assessments individually.
- Asthma control. We used random patient effects models using ACT scores as a continuous and binary outcome (19-25 vs 5-18). Since ACT scores were measured at irregular times and repeated multiple times for some patients, we used a compound symmetry working covariance to avoid estimating too many nuisance variance parameters. In analyses, similar to QOL, we provided mean changes from baseline to first, second, third, and fourth quarter, with initial tests treated as baseline values. We also related ACT scores to overall e-AT adherence by adding as a covariate cumulative proportion of weeks with e-AT use before and including that week (dichotomized as 60%+ vs 60%−) during follow-up period. We reported estimated mean changes from baseline to each quarter for compliers and noncompliers, and contrasts between compliers and noncompliers.
- Parent satisfaction. Analyses similar to QOL, were repeated for satisfaction score used as a continuous outcome using LFE model. We also dichotomized satisfaction score as satisfactory (>3) vs unsatisfactory (≤3). GLFE patient effects model with binomial distribution and logit link were used to fit this binary outcome.
- Interrupted/missed school/workdays, ED/hospital admissions, oral steroid use. We assessed changes in rates of ED/hospital admissions, oral steroid use, interrupted/missed school days, and interrupted/missed workdays, between baselines and 3-, 6-, and 12-month follow-up assessments, applying a generalized estimating equation (GEE) with robust SEs and compound symmetry working covariance model under Poisson or negative binomial model. The model estimated the overall rate ratios for the outcomes between baseline and the 3 follow-up assessments.
- Subgroups analyses of QOL and asthma control for Hispanic/Latino and Medicaid children. Analyses were similar to QOL and asthma control described above but restricted to the subgroups. The goal was to assess the e-AT impact on disadvantageous populations who experience asthma disparities. Most Hispanic patients in Utah are of low-income families and face multiple barriers to care.
Comparisons of ED/hospital admissions and oral steroids used between the year following and the year before each patient's enrollment visit
We used clustered negative binomial regression (to account for matching of the preperiods and postperiods within each participant) and included offsets to account for the duration of time each patient was evaluated for hospital/ED admissions and oral steroids used during the pre e-AT and post-e-AT periods. The analysis compared the baseline period with the full follow-up period for each participant, irrespective of whether a portion of the follow-up period was under the intensive or standard e-AT intervention.
Comparison of ED/hospital admissions and oral steroid use between patients enrolled in the 6 early starting clinics vs patients enrolled in the 5 late starting clinics, during months 1 to 3 delay
Patients enrolled in the early starting clinics (clinics that started on January 1, 2014) were already using the standard e-AT while those enrolled in the late starting clinics (clinics that started on April 1, 2014) were receiving usual care during the 3-month delay period (between January 1, 2014, and March 31, 2014), used as usual care period. Effects were compared during the delay period using weighted averages of estimated event rates between early vs late starting clinics. We applied GLME models for negative binomial with logarithmic link functions and random site effects to compare the rates of hospital/ED visits and oral steroid use occurring during the delay period. The outcome model included offsets to account for each participant's duration of follow-up during the period of analysis.
Comparison of ED/hospital admissions and oral steroid use 1 year post-e-AT initiation for patients who started the intervention early vs during a 1-year period before starting the e-AT for those who started the e-AT later
Analyses (patient level) compared rates of ED/hospital admissions and oral steroid use during 1-year post-e-AT initiation for those who were enrolled early (between January 2014 to December 2014) or in the first year (early starting patients), while using the e-AT (intervention period), to rates during a 1-year period before enrollment of patients who were enrolled late (between January 2015 to December 2015) or in the second year (late starting patients), while not using the e-AT (usual care period).
Rationale
Participants in the control group (late patients were receiving usual care) later met study eligibility criteria, and their preenrollment usual care time periods (including change in seasons) coincided with the postenrollment time periods of those who enrolled early, limiting the confounding effects of secular trends in asthma care. A GLME model was used with a negative binomial distribution, logarithmic link function, and random site effects to compare the rates between the 2 groups.
Nonrandomized comparison of outcomes between patients from randomized clinics while using the e-AT vs a nonrandomized matched control cohort of children with persistent asthma from nonparticipating clinics
The above-described analyses allowed comparisons between different times, leading to possible secular trends bias. The requirement that the control period ends before e-AT initiation and that the intervention period starts after index clinic visits was also a source of asymmetry that could lead to bias. To address these potential biases, we added another nonrandomized analysis, comparing outcomes between patients from randomized clinics while using the e-AT to a matched control group of patients from nonparticipating clinics. This analysis compared the e-AT with a control group not exposed to the e-AT, using concurrent evaluation periods; and addition of new clinics also increased statistical power.
Matching variables
Randomized patients (2 controls per 1 treated patient) were matched by age, sex, clinic location, asthma severity (persistent, based on controller use with at least 2 prescriptions within the past year), clinic visit dates for controls within ±2 months of enrollment dates of intervention patients, and clinic visit types (asthma vs non-asthma related).
Data elements
We pulled the following data from the IH EDW (both intervention groups and matched controls): unique patient identifier, account number, birthdate/age at clinic visit, clinic visit date, sex, insurance, clinic name, diagnosis (using ICD-9 and ICD-10 codes), controller prescription and steroid use (using national drug codes), and ED/hospital admissions. Matched controls were drawn from 42 nonparticipating clinics within the IH system located in Salt Lake City and Utah County, where randomized clinics are located.
Statistical analysis
We compared ED/hospital admissions and steroid use rates during 12-month follow-up period between patients on e-AT vs matched controls using GLME with a negative binomial outcome, logarithmic link, and random site effects. The model used each matching variable and prior ED/hospital admission and steroids use rates in the preceding 12 months as covariates. The basic model provided overall comparisons between e-AT intervention and controls, irrespective of the intervention used (standard or intensive e-AT).
Objective 2: Assess Whether the Effect on Child Outcomes Varies Across Parent Characteristics (High vs Low Education, Medicaid vs Private Insurance, Frequent vs Infrequent Users)
We assessed the e-AT (standard and intensive interventions) effectiveness in different parent subgroups (eg, high vs low education, private vs Medicaid insurance). Health literacy was replaced by education because of lack of time by CCC and difficulty of data collection during clinic visits.
For ED/hospitalization admissions, we used Cox regression to relate the risk of first hospital/ED admission to the cumulative proportion of e-AT use (numbers of weeks) before that admission in a 3-month window, along with indicator variables for low education and Medicaid insurance at baseline, and an interaction term between indicator variables. The baseline hazard function was stratified by clinic site. We also assessed the dose-response relationship between risk of hospital/ED admissions and the cumulative proportion of e-AT use (categorized), comparing patients with vs without low education and Medicaid vs private insurance subgroups.
For asthma control, we applied GEE for binary ACT scores (19-25 vs 5-18) with logistic link function and robust SEs under an independence working covariance model to relate the odds of good control during a given week to the cumulative proportion of e-AT use (in a 3-month window) before that week, using the same sets of covariates (plus clinic site as a covariate rather than a stratification factor) and interaction terms to compare dose-response relationships of the odds of good asthma control with prior cumulative proportion of e-AT use between low vs high education and Medicaid vs private insurance.
For QOL, we used GEE by applying a normal outcome model with an identity link function, and QOL measurements at 3, 6, and 12 months each related to the cumulative proportion of e-AT use before the week of QOL measurement, using the above covariates and interaction terms. We also compared dose-response relationships of mean QOL with prior cumulative proportion of e-AT use across the 2 subgroups.
Objective 3: Determine Factors Associated With Sustained Parent Participation in Asthma Self-management
We defined a dichotomous outcome for each month as “frequent” (if ≥3 weekly assessments recorded/month) or “nonfrequent” e-AT users otherwise. We used a logistic regression model to relate the odds of frequent e-AT use to sets of predictor variables after adjusting for clinic (categorical factor), with the following sequence:
- We applied subject-matter considerations to define baseline predictors, including demographic (parent age, sex, and race/ethnicity), socioeconomic (marital status, highest education completed, household income, number of children in household, and primary language spoken), behavioral, and technology factors, and subdivided predictors into modifiable and nonmodifiable.
- We examined correlation matrices, variance inflation factors, and contingency tables to identify any collinear factors and reduce the list of predictors, if needed.
- We examined the association of e-AT use frequency with each predictor by fitting separate GEE for each predictor, with clinic used as a categorical covariate.
- We introduced the assignment to the intensive e-AT as a time-dependent covariate in each GEE model. We examined interaction terms between baseline predictors and treatment assignment to determine if the association of sustained participation and predictor variables differs in time period when the intensive e-AT was applied vs time period when it was not applied.
- We used multivariable GEE regressions to relate frequent use to each individual modifiable risk factor after adjustment for nonmodifiable factors, the time-dependent intensive e-AT indicator variable, and clinic as covariates.
Strategies for Handling Missing Data
Analyses described above were all applied to all available data, allowing all nonmissing variables to be included regardless of whether measurements were missing at a time point, as missing data seemed to occur at random. In general, analyses using the mixed effects models utilize likelihood-based statistical inference and remain unbiased so long as data are missing at random. Also, analyses using GEE remain nearly unbiased as long as data are missing completely at random, making use of all nonmissing measurements of variables in the model, including baseline covariates.
Conduct of the Study
All changes to the original protocol, including reasons for changes, are described in previous sections of this report. Any changes that occurred after the study start date were documented in the study protocol. We obtained PCORI and IRB approvals before implementation of the study and subsequent changes.
Results
Objective 1: Assess the Effectiveness of the New Ambulatory Care Model
Of the 11 participating clinics, 2 had only 1 patient enrolled. These 2 clinics (and the 2 patients) were excluded from all analyses. Of the 325 children/parents enrolled, 318 (97.2%) completed their baseline surveys and were included in descriptive analysis of baseline characteristics. Figure 8 (CONSORT diagram) shows the study population at different times. The dropout rates at 3, 6, and 12 months were similar between the 2 groups, including 10.0%, 12.7%, and 20.9%, respectively, for the standard e-AT intervention, and 13.3%, 13.3%, and 20.0%, respectively, for the intensive intervention. (The 4 ClinicalTrials.gov tables [patient flow, baseline characteristics, outcomes and statistical analysis, and adverse events] are available at ClinicalTrials.gov.)
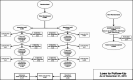
Figure 8
CONSORT Diagram.
Randomized Comparisons Between Standard and Intensive e-AT Interventions
Of the 318 children, 261 (82%) received the standard e-AT intervention and 57 (18%) the intensive e-AT intervention. Table 2 provides baseline characteristic distribution of the study population.
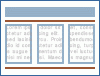
Table 2
Baseline Characteristics of Children Who Receive Intensive vs Standard e-AT Interventions.
Primary outcome (QOL)
Overall baseline average QOL scores were 77.5 (95% CI, 71.1-83.8) points for the standard e-AT and 79.2 (95% CI, 72.5-85.9) for the intensive e-AT (P = .713). There was a significant QOL improvement over baseline at 3, 6, and 12 months (P < .001) following implementation of the standard and intensive e-AT interventions individually and overall (Tables 3 and 4). However, when compared together there was no significant difference (P = .961) between the 2 interventions. Figure 9 shows change from baseline (x-axis) at different follow-up times, with highest QOL increase occurring at 3 and 12 months, for both interventions. Overall, both interventions were effective in improving QOL over time. Although participants on the standard e-AT intervention tended to have highest QOL scores at all follow-ups, no statistical difference were found between them. Therefore, additional motivational mechanisms built within the intensive e-AT intervention to improve user adherence did not make any differences in outcomes.
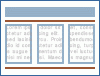
Table 3
Summary of QOL by Clinic and by Timeline.
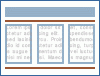
Table 4
QOL/Intensive vs Standard e-AT Interventions at Different Follow-ups (Fixed-effects Model).
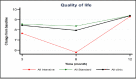
Figure 9
Least-Squares Means of Change (x-Axis) in QOL: Intensive vs Standard.
Asthma control
Overall, asthma control scores increased significantly (P < .001) in both intensive and standard e-AT interventions individually and overall (Figure 10 and Tables 5 and 6), across all follow-up quarters. However, increases in asthma control among participants on the intensive e-AT tended to be lower than increases seen in those on standard e-AT. However, when compared, there was no significant difference (P = .926) in asthma control between the 2 groups.

Figure 10
Least-Squares Means of Change (x-Axis) in Asthma Control Scores: Intensive vs Standard.
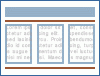
Table 5
Summary of Asthma Control Score per Quarter and per Clinic.
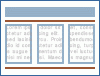
Table 6
Asthma Control/Intensive vs Standard Interventions at Different Follow-ups (Fixed Effect).
Interrupted/missed school days
Similar to QOL and asthma control, number of interrupted/missed school days were reduced significantly (P < .001) across all follow-ups (month) in both intensive and standard e-AT interventions individually and overall (Figure 11). Also, overall reduction in number of interrupted/missed school days among participants on the intensive e-AT tended to be lower (especially at 3 and 6 months) than reductions seen in patients on standard e-AT (Table 7). Yet, when compared, there was no significant difference (P = .493) in asthma scores between the 2 intervention groups.
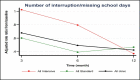
Figure 11
Least-Squares Means of Change (x-Axis) in Interrupted/Missed School Days: Intensive vs Standard.
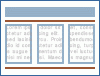
Table 7
Interrupted/Missed School Days/Intensive vs Standard Interventions Across Follow-ups (Fixed Effect).
Interrupted/missed workdays
Fixed-effects analysis showed no change in the number of interrupted/missed workdays across all follow-up periods for the intensive e-AT, but there was a significant (P < .001) reduction in standard e-AT overall and at 3, 6, and 12 months. Although those patients receiving the intensive intervention tended to have fewer interrupted/missed workdays, there was no significant difference (P = .119, except at 6 months) between intensive and standard e-AT interventions (Figure 12 and Table 8).
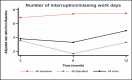
Figure 12
Least-Squares Means of Change (x-Axis) in Interrupted/Missed Workdays: Intensive vs Standard.
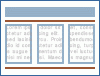
Table 8
Interrupted/Missed Workdays/Intensive vs Standard Interventions Across Follow-ups (Fixed Effect).
Parent satisfaction
Satisfaction scores ranged from 1 to 5 (1 = very dissatisfied, 2 = dissatisfied, 3 = neutral, 4 = satisfied, and 5 = very satisfied). Patients in the intensive group experienced a slight but not significant (P = .09) reduction of 0.14 points in satisfaction score, while patients in the standard e-AT experienced a significant (P < .001) but minimal reduction of 0.24 points in satisfaction score. Overall, there was no significant difference (P = .244) between the 2 groups.
ED/hospital admissions
The average ED/hospital admission was 0.15 (0.50 SD) for the overall study population, and 0.22 (0.60 SD) during preintervention and 0.09 (0.35 SD) during postintervention. Compared with baseline, both intensive (relative risk [RR], 0.12; 95% CI, 0.05-0.25; P < .001) and standard (RR, 0.08; 95% CI, 0.04-0.16; P < .001) interventions achieved a significant reduction in ED/hospital admissions. However, there was no significant difference (P = .505) in ED/hospital admissions between the 2 intervention groups.
Longitudinal Within-Participant Analyses
These analyses assessed within-patient longitudinal change in outcomes (QOL, satisfaction, asthma control, interrupted/missed school days, and interrupted/missed workdays) compared between baseline (before e-AT initiation) and follow-up assessments while using the e-AT.
Quality of life
Overall, average QOL scores were 79.1 at baseline and 90.9, 90.0, and 90.6 at 3, 6, and 12 months, respectively. The increase from baseline was the highest (12 points) at 3 months and then plateaued around 90 through the end of the study (Table 9). Patterns of increase (P < .001) in QOL scores occurred not only overall but also at almost all individual clinics (Figure 13 and Figure 14).
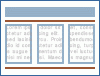
Table 9
Longitudinal Within-Participant Analysis of QOL: Summary by Clinic and Timeline.
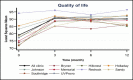
Figure 13
Least-Squares Means of Change (x-Axis) in QOL Scores Over Time.
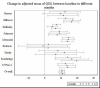
Figure 14
Change in QOL Between Different Follow-up Time and Baseline Score, and by Clinic.
Asthma control
Average asthma control scores at baseline and quarters 1, 2, 3, and 4 were 18.8, 22.3, 22.8, 22.8, and 22.9, respectively, with the highest increase occurring in the first quarter and then plateauing at about 22 to 23 for the remaining follow-up quarters (Figure 15). Overall, asthma scores increased significantly (P < .001) in most clinics and across all follow-up quarters (Figure 16).
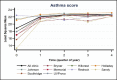
Figure 15
Least-Squares Means of Change (x-Axis) in Asthma Control Scores Over Time.
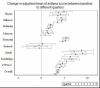
Figure 16
Change in Asthma Control Between Different Follow-up Times and Baseline Score, and by Clinic.
Interrupted/missed school days
The overall average number of interrupted/missed school days at baseline was 1.91, and 0.79, 0.52, and 0.79 at 3, 6, and 12 months, respectively, with the largest reduction occurring by 3-month follow-up. A reduction (P < .001) in the number of interrupted/missed school days was seen across most clinics (Figure 17) and follow-up schedules (Figure 18).
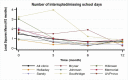
Figure 17
Least-Squares Means of Change (x-Axis) in Number of Interrupted/Missed School Days Over Time.
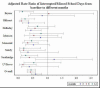
Figure 18
Change in Interrupted/Missed School Days Between Different Follow-ups and Baseline, and by Clinic.
Interrupted/missed workdays
The overall average number of interrupted/missed workdays was 0.72 at baseline and 0.27, 0.25, and 0.19 at 3, 6, and 12 months, respectively, with the largest reduction occurring at 3-month follow-up. A reduction in the average number of interrupted/missed school days was seen (P < .001) at most clinics (Figure 19) and follow-up schedules (Figure 20).
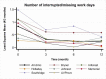
Figure 19
Least-Squares Means of Change (x-Axis) in Number of Interrupted/Missed Workdays Over Time.

Figure 20
Change in Interrupted/Missed Workdays Between Different Follow-ups and Baseline, and by Clinic.
Parent satisfaction
The overall average satisfaction score was 4.7 at baseline and was reduced (−0.21) to 4.5 points (P < .001) at 12 months (Figure 21). Fixed modeling analysis showed a significant reduction of points in satisfaction score. However, this reduction in parent satisfaction was slight and not clinically meaningful, as the 12-month score (4.5) was still within the highest satisfaction range (4-5). (Note: satisfaction score addressed multiple facets of overall asthma care, beyond the influence of the e-AT). Also, when scores were dichotomized to >3 vs ≤3, analyses showed no significant differences between baseline and 12-month scores.
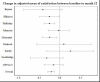
Figure 21
Change in Parent Satisfaction Between Different Follow-ups and Baseline, and by Clinic.
Comparisons of the Frequency of Hospital/ED Admissions and Oral Steroid Use Between the Year Following Each Patient's Enrollment Visit and the Year Before the Enrollment Visit
The analysis compared overall hospital/ED admissions and oral steroid use in the study population (both standard and intensive e-AT), 1 year before initiation vs 1 year postinitiation of the e-AT.
ED/hospital admissions
The average ED/hospital admission was 0.15 (0.50 SD) overall, and 0.22 (0.60 SD) during preintervention and 0.09 (0.35) postintervention, and with an adjusted RR from preintervention to postintervention of 0.68 (95% CI, 0.49-0.95; P = .024), demonstrating significant reduction in the postintervention period. At individual clinics, ED/hospital admissions were significantly reduced in 2 clinics and tended to have a lower RR (though not significant) at most of the remaining clinics (Figure 22), probably because of limited power.
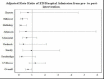
Figure 22
Adjusted Rate Ratio of ED/Hospital Admissions From Preintervention vs Postintervention, and by Clinic.
Steroid use
The overall average oral steroid use was 0.63 (1.1 SD and 0.74 [1.2 SD]) days during preintervention and 0.51 (0.95 SD) days postintervention, and with an adjusted RR from preintervention to postintervention of 0.74 (95% CI, 0.61-0.91; P = .003), demonstrating significant reduction in the postintervention period. At individual clinics, oral steroid use was significantly reduced in 2 clinics and tended to have a lower RR (although not significant) at most of the remaining clinics (Figure 23), probably because of limited power.
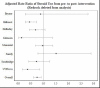
Figure 23
Adjusted Rate Ratio of Oral Steroid Use From Preintervention vs Postintervention, and by Clinic.
Comparison of ED/Hospital Admissions and Oral Steroid Use Between Patients Enrolled in the 6 Early Starting Clinics vs Patients Enrolled in the 5 Late Starting Clinics, During Months 1 to 3 Delay
This clinic-level (early vs late starting clinics) analysis compared oral steroid use and hospital/ED admissions between patients enrolled in the early starting clinics (clinics that started the e-AT on January 1, 2014, while late starting clinics were not using it) and patients enrolled in the late starting clinics (clinics that started the e-AT on April 1, 2014), during the 3-month delay period (between January 1, 2014, and March 31, 2014), used as usual care period. Although data were collected for 1 year before enrollment and 1 year following enrollment (see the “Data Collection and Sources” section), analysis was restricted to outcomes occurring during months 1 to 3 delay period (between January 1, 2014, and March 31, 2014). Only 2 participants had ED/hospital admissions and only 4 participants had steroid use during the 3-month period. Data were too sparse to be fitted for the LFE or GLME, and the delay period was not long enough to have enough power for ED/hospital admissions.
Comparison of ED/Hospital Admissions and Oral Steroid Use 1 Year Postinitiation of e-AT for Patients Who Started the Intervention Early With Those Who Started the e-AT Later, During a 1-Year Period Before Starting the e-AT Intervention
This patient-level analysis compared rates of ED/hospital admissions and oral steroid use during 1-year post-e-AT initiation for patients who were enrolled in the first year (between January 2014 to December 2014, also known as early starting patients) and were using the e-AT (or were in the intervention period), with rates during a 1-year period before enrollment of patients who were enrolled in the second year (between January 2015 to December 2015, or late starting patients), when they were not using the e-AT (usual care period).
Rationale
Participants in the control group (late starting patients were receiving usual care) later became e-AT users (or met study eligibility criteria) and their preenrollment usual care time periods (including change in seasons) coincided with the postenrollment time periods of those who enrolled early, limiting the confounding effects of seasonal change and secular trends in asthma care.
Due to sparseness of data (Table 10), we were not able to fit a GLME model with either fixed or random site effects to compare the rates of ED/hospital admissions and oral steroid use between the 2 groups. We had to combine all 1-year-before-enrollment data for late starting participants and compare it to all 1-year-after-enrollment data for early starting participants. Overall, the average number of ED/hospital admissions was high, at 0.18 (0.57 SD) per year, in the late starting patients (during the time they were not exposed to the e-AT) and low, at 0.10 (0.38 SD) per year, in the early starting patients (during the time they were exposed to the e-AT). However, when comparing late vs early patients, this difference was not statistically significant (P = .183), with an RR of 1.74 (95% CI, 0.77-3.93). In addition, the average numbers of oral steroid use were similar, with 0.53 (0.92 SD) per year in the late starting patients and 0.53 (0.99 SD) per year in the early starting patients; we found no significant differences (P = .937) between the 2 groups.
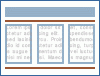
Table 10
Number of Early vs Late Starting Patients by Clinic.
Nonrandomized Comparison of Outcomes Between Patients From Randomized Clinics While Using the e-AT vs a Matched Control Cohort of Children With Persistent Asthma From Nonparticipating Clinics
The analyses in the preceding 2 sections allowed us to compare rates of poor asthma control in different periods, some in which e-AT was used and some in which it was not used. These comparisons of care outcomes with vs without e-AT at different times could be confounded by secular trends in asthma care. The nonrandomized comparison in this section addresses this potential bias. We compare asthma outcomes during the same periods in patients (cases) from randomized clinics that were using the e-AT to a matched control group of patients from nonparticipating clinics.
Comparison of ED/hospital admissions
There were 928 participants (325 cases and 603 matched controls) for the analyses (Table 11). The overall average ED/hospital readmissions (per 1000 days) was 0.42 (1.36 SD) for cases and 0.23 (0.99 SD) for controls. Among cases, ED/hospital readmissions were 0.59 (1.64 SD) during the pre-e-AT period and 0.24 (0.97 SD) during the post-e-AT period. However, among controls, ED/hospital readmissions were 0.23 (1.01 SD) during pre-e-AT initiation and 0.24 (0.97 SD) during post-e-AT initiation. Random-effects analysis showed the ED/hospital readmissions for cases were significantly reduced among cases (RR, 0.43; 95% CI, 0.27-0.67; P = .0003) but not among controls (RR, 1.04; 95% CI, 0.69-1.58; P = .817). The intervention effect was RR of 0.41 (95% CI, 0.22-0.75; P = .0041).
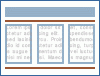
Table 11
Baseline Characteristics Between Cases and Matched Controls.
Comparison of oral steroid use
Overall average oral steroid use (per 1000 patient days) was 1.71 (2.90 SD) for cases and 1.93 (6.0 SD) for controls. Among cases, oral steroid use was 2.02 (3.14 SD) during the pre-e-AT initiation and 1.41 (2.60 SD) during post-e-AT initiation. Among controls, oral steroid use was 1.87 (3.12 SD) during preinitiation and 1.99 (7.89 SD) during postinitiation of the e-AT. Fixed-effects models showed the oral steroid use for cases was significantly reduced preinitiation to postinitiation of e-AT among cases (RR, 0.69; 95% CI, 0.52-0.93; P = .0139) but not controls (RR, 1.06; 95% CI, 0.87-1.31; P = .5565). The intervention effect was RR, 0.65 (95% CI, 0.46-0.93; P = .0191).
Subgroup Analyses (Longitudinal Within-Participant Analyses)
We conducted the following subgroup analyses on the overall study population, or 318 patients who received either standard or intensive e-AT intervention and completed baseline assessments.
QOL for Hispanic/Latino ethnicity
Overall, average QOL scores were low (73.9 points) at baseline and increased to 87.3, 87.8, and 90.9 at 3, 6, and 12 months, respectively. The increase (P < .001) from baseline was 12.6 and 13.7 points at 3 and 6 months, with the highest increase of 16.6 points (higher than the increase observed in the overall study population) occurring at 12 months. We saw similar patterns of high improvements among other minority subgroups.
Asthma control for Hispanic/Latino ethnicity
Average asthma control scores were 17.3, 22.4, 22.8, 23.2, and 22.9, respectively, at baseline and at quarters 1, 2, 3, and 4, with the highest increase occurring by the first quarter and plateauing around 22 to 23 the remaining quarters, similar to the overall study population. The adjusted mean changes compared with baseline were 4.8, 5.1, 5.5, and 5.4 at quarters 1, 2, 3, and 4, respectively, and were constantly higher than the improvement seen in the overall study population, which was 3.0, 3.5, 3.5, and 3.6 at quarters 1, 2, 3, and 4, respectively. We also saw similar patterns of high improvements among other minority subgroups.
QOL for patients with Medicaid insurance
Overall, average QOL scores were low (76.0 points) at baseline and increased to 91.6, 89.1, and 87.7 at 3, 6, and 12 months, respectively. The increase (P < .001) from baseline was 14.1, 12.9, and 11.3 points at 3, 6, and 12 months, respectively. The increase at 12 months was similar to that of the overall study participants. We also saw similar patterns of high improvements among other minority subgroups.
Asthma control for patients with Medicaid insurance
Average asthma control scores at baseline and quarters 1, 2, 3, and 4 were 18.0, 22.2, 22.5, 22.3, and 23.6, respectively, with the highest increase occurring by the first quarter and then plateauing around 22 to 23 the remaining quarters, as seen in the overall study participants. The adjusted mean change compared with baseline was 4.0, 4.4, 4.1, and 4.7 at quarters 1, 2, 3, and 4, respectively, and was constantly higher than improvement seen in the overall study participants, which was 3.0, 3.5, 3.5, and 3.6 at quarters 1, 2, 3, and 4, respectively. We also saw similar patterns of improvements in other minority subgroups.
Objective 2: Assess Whether the Effect on Child Outcomes Varies Across Parent Characteristics
Demographics
Analysis involved the overall study population (325 children), for which 53 (16.3%) had Medicaid coverage, 203 (62.5%) had private insurance, 5 (1.5%) were self-paying, and 64 (19.7%) had unknown insurance. The median number of children in these families was 3 (interquartile range [IQR], 2-4); 226 (83%) were from married families, and 243 (89%) had 1 member of the household with some college education (Table 12). Overall, average adherence (as measured by frequency of weekly use of the e-AT) to e-AT dropped in the first month and stabilized afterward. The overall average adherence was about 65% at the end of the 1-year study period, slightly lower in patients using the intensive e-AT vs those on standard e-AT intervention (Figure 24).
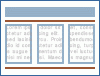
Table 12
Family Demographics of Children With vs Without ED/Hospital Admissions.
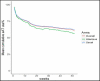
Figure 24
Average Cumulative Proportion of e-AT Adherence (or Use) by Week.
Association of ED/Hospital Admissions (First Admission) and Frequency of e-AT use
Of the 325 families included in the analysis, 212 (65.2%) were frequent and 113 (34.8%) infrequent e-AT users. Thirty-eight (18%) and 18 (16%) of frequent and infrequent e-AT users had first ED/hospital admissions during the study period. Reduction in ED/hospital readmissions among patients with private insurance was slightly higher (62%) when they were frequent e-AT users (hazard ratio [HR], 0.38; 95% CI, 0.18-0.83; P = .02); however, being infrequent users did not have this association. Overall, we found ED/hospital admissions varied by insurance coverage. Specifically, patients with private insurance were 60% less likely (HR, 0.40; 95% CI, 0.21-0.75; P = .005) to have first ED/hospital readmission compared with those with Medicaid.
Association of Asthma Control and Frequency of e-AT Use
We found that child's asthma control did not vary across education level and insurance coverage, and frequency of e-AT use did not change this relationship.
Association of QOL and Frequency of e-AT Use
Overall, we found that child's QOL did not vary across education level and insurance coverage. However, parents with some college or more education had significantly reduced QOL (effect: −9.60; 95% CI, −15.35 to −3.85; P = .001) relative to those with high school graduate or less education, among those who were infrequent e-AT users.
Objective 3: Determine Factors Associated With Sustained Parent Participation in Asthma Self-management
Analysis of individual univariate factors revealed that higher income (β = .2343; SE = .0784; P = .0028) was associated with sustained e-AT use, while being married (β = −1.3327; SE = .4801; P = .0055) and having high education (β = −1.2596; SE = .6088; P = .0387) were associated with less sustained e-AT use; however, none of these factors was significant in a multivariable model, when controlling for time-dependent intensive intervention. Further, analyses of open-ended questions revealed that improved management of asthma symptoms, provider and staff support, and use of gift card/incentives were the most common factors that facilitated use of e-AT in clinics, while lack of physician buy-in or staff support, and lack of time and e-AT integration with the electronic medical records were commonly reported barriers.
Discussion
Decisional Context
When comparing clinics randomized to intensive vs standard e-AT, we found no statistical differences in all outcomes. We also found no significant differences in oral steroid use and ED/hospital admissions when comparing outcomes between early starting clinics and late starting clinics during the 3-month delay period. Similarly, we did not find any statistically significant differences (although late starting patients had higher ED/hospital readmissions) between early starting patients and late starting patients. However, when we compared outcomes between receiving the e-AT intervention relative and not receiving the e-AT (or usual care), we found clinically and statistically significant differences. Specifically, we found a significant improvement over time in outcomes from baseline (with no e-AT use) in either standard or intensive or both e-AT interventions overall (see Figures 9-12), as well as in individual clinics. We saw this change across all outcomes (except parent satisfaction), including improvement of child's QOL and asthma control, reduction of child's interrupted/missed school days and parent's interrupted/missed workdays, and reduction of child's oral steroid use and ED/hospital admissions. Also, when comparing the year following patient's e-AT enrollment to the year before enrollment, we found a significant reduction in oral steroid use and ED/hospital admissions overall, and in individual intervention arms, as well as at most clinics. Further, when comparing with matched controls from clinics that did not implement e-AT, we found participants from clinics that received e-AT interventions had significantly lower oral steroid use and ED/hospital admissions.
The current ambulatory asthma care model is reactive and inefficient, and focuses on episodic acute care, addressing treatment of recurrent asthma attacks. Our study is among the few to demonstrate multiple outcomes for the child, parent, and clinic can be improved through implementation of a potentially low-cost health information technology (the e-AT), promoting early identification of signs of asthma control deterioration to prompt timely interventions. Our intervention represents a shift in ambulatory asthma care, from the current reactive acute care to a new model that is continuous and proactive, focusing on prevention of asthma attacks. Our results are helpful not only to families but also to health care providers, payers, and other community stakeholders willing to improve asthma care.
Study Results in Context
Previous studies have reported that adults who engage in effective self-management of a chronic disease have less activity limitation, improved QOL, improved perceived disease control, and improved medication adherence.13,17-20 However, self-management interventions in children with asthma remain rare. The few previous studies of self-management interventions have either not evaluated the effect on asthma outcomes22,23 or have reported mixed results with no effect21,24-27 or little effect on asthma outcomes,28,29 limiting their use in clinical practice.56 Our intervention is among the few to demonstrate a significant improvement in child, parent, and clinic outcomes. With the e-AT, improvements in asthma control and QOL exceed those previously reported. For instance, Voorend-van Bergen reported a 2-point change in ACT scores as the minimal important difference.57 In our e-AT intervention, improvement of ACT scores for patients who received the e-AT was 4 points already by the first quarter and was sustained through the end of the 12-month study period. Contrary to Rikkers-Mutsaerts's study (consisted of weekly asthma control monitoring with the asthma control questionnaire and treatment advice by a web-based algorithm), which reported improvement in QOL at 3 but not 12 months, our study of e-AT, a web-based intervention, showed sustained QOL improvement throughout the study duration.58
Studies have reported that parents often lack critical information and tools to guide them in recognizing and acting on warning signs.33 The e-AT has the potential to improve child asthma self-management, and thereby outcomes, by (1) increasing patient/parent roles in managing symptoms, including provision of self-care decision support; and (2) increasing awareness of chronic asthma symptoms through regular assessment and prompting patients to seek appropriate and timely visits with their PCPs when chronic control is deteriorating instead of waiting until they experience a severe asthma attack.5,59-61 Ultimately, parents are empowered to recognize and act on early warning signs of declining asthma control or impending asthma attacks, including subtle symptoms that are usually missed. By increasing awareness of chronic asthma symptoms, the e-AT led to improved treatment of asthma, which ultimately resulted in better QOL and reduced use of acute health care services, potentially from optimal use of asthma medication.62
Asthma guidelines recommend health care providers complete regular assessments of chronic asthma control, as it prompts timely and appropriate adjustments of therapy.6 However, studies continue to show that providers often lack or do not assess information about chronic asthma control. Lack of assessment results in failure to prescribe or appropriately adjust asthma therapy, leading to under treatment.63,64 Provider failure to assess asthma control is attributed to a busy schedule, lack of resources, limited ability and time to support ongoing monitoring outside clinical encounters, and lack of an effective follow-up process between regular clinic visits.63,64 The e-AT provides care providers with an effective way to monitor patients outside clinical encounters, providing real-time information about child asthma control status over time to capture early signs of deteriorations. Longitudinal information about a patient's clinical status is available to the provider for use in medical decision making rather than the provider relying on 1 assessment at the time of an acute visit and patient/family recall.
Despite potential benefits, implementation of self-management interventions in clinical practice remains low, and their uptake by patients and patients' adherence is often poor and declines significantly over time with high participant attrition.65,66 van der Meer et al, for instance, reported a low adherence with weekly use of the ACQ for self-management of about 53% at 12 months.16 In our study, although adherence decreased over time, parent engagement remained high and stayed above 60% at the end of the 12-month study period. High adherence was likely due to our approach of providing training and support to PCPs' offices to deliver the intervention themselves. Participants were motivated to comply with weekly use of the e-AT knowing that the PCP would examine their data (based on verbal communication with some participants and clinic staff).
Implementation of Study Results
Implementing e-AT at clinics requires a designated CCC and support from PCPs. In a typical clinic, asthma patients are identified (and approached) at the time of an office visit or by mail/phone invitation. Clinic personnel enroll the patient (providing training, connecting families to the e-AT), which takes about 30 minutes. Although not formally measured, ongoing monitoring is very efficient. For instance, in one clinic, the CCC spent 30 to 40 minutes per week on average managing about 100 patients (this included receiving alerts, logging into the e-AT dashboard, reviewing the list of patients with low scores, making follow-up calls to identify and address issues, and scheduling an appointment with the PCP if needed). The e-AT dashboard has a tab that provides the list of patients (usually about 5/week) with low scores (ie, ones that trigger an alert) for the CCC to follow up, allowing the CCC to quickly focus attention only on those in need of follow-up (rather than reviewing the data of each patient) and thereby minimizing workload.
Morrison et al reported that digital interventions are poorly described, with insufficient information about barriers and facilitators to their uptake and utilization.67 In our survey, the most important barriers to clinical staff adoption of e-AT in clinics were lack of physician buy-in and staff support. Clinic-level implementation needs to focus on securing PCP buy-in through education and training. The anticipated risks of e-AT use are minimal and primarily related to disclosure of protected health information. Fortunately, the e-AT has rigorous security protections as well as adherence to HIPAA privacy and data security requirements. Overall, we observed no adverse events.
Generalizability
The e-AT supports the use of evidence for best asthma care practices in the ambulatory care setting and helps meet self-management needs of not only children but also parents and PCPs. Also, our study showed outcome improvements with use of the e-AT among disadvantaged populations (see the “Subpopulation Considerations” section), and the e-AT may be generalized to inner-city children who experience asthma care and outcome disparities. Therefore, dissemination of our e-AT care model can lead to major improvements in asthma care and outcomes, with potential reductions in asthma-related health care costs in the United States, and can serve as a model to improve care for other pediatric chronic diseases.
Subpopulation Considerations
Underserved populations, especially low-income Hispanic and black children, experience asthma care and outcome disparities compared with well-served populations. In our study, we found, among those who used the e-AT, that having Medicaid insurance (compared with private insurance) and low education level (compared with high education level) tended to be associated with shorter time to hospital readmission. Yet, we noted nonsignificant improvements for frequent e-AT users with Medicaid or low education level. Also, our subgroup analysis demonstrates that QOL and asthma control also significantly improved among Hispanic patients (the largest minority population in Utah), other minority patients, and those with Medicaid, who also achieved improvements that were higher across all follow-up quarters than the overall study population. Thus, disadvantaged populations seem to benefit more from this new care model, suggesting the e-AT might also be a tool to address asthma disparities among disadvantaged and minority populations.
Study Limitations
Our study has several limitations, resulting from the following:
- We changed the design to compare intensive to standard e-AT interventions, rather than a randomized comparison of the e-AT intervention with usual care (control). We relied on several nonrandomized controls and pre-post comparisons to evaluate the e-AT effectiveness compared with usual care. Our study results should be interpreted with caution as unrecognized biases may have been introduced by using nonrandomized controls and pre-post comparisons. Thus, factors other than e-AT may be responsible of our results.
- Enrollment was unbalanced across the clinics in the randomized comparison (standard vs intensive e-AT), with 3 of the 4 high-patient-enrolling clinics (>20 patients per clinic) randomly assigned to the standard intervention and only 1 clinic assigned to the intensity intervention, leading to 60 enrolled patients in the standard intervention vs 60 enrolled patients in the intensive e-AT intervention. As a result, the power to estimate between-site variation in QOL and other outcomes was limited due to variable participant enrollment numbers across the clinics, particularly for statistical inferences under mixed models that account for intersite variation, and for nonnormally distributed outcomes. As a result, we also used fixed-effects analyses that applied statistical inferences specifically to participating clinics. However, when the fixed-effects approach is used, generalization of results from the current study sample to all other potential samples not in the study (beyond the specific enrolled clinics) may be limited. Applicability of results should be based on subject-matter considerations, since it depends on other unmeasured clinic factors.
- Assignment of some (21%) patients to the standard e-AT before randomization led to different periods of postrandomization follow-up, adding complexity to the statistical analysis. Fortunately, 79% of the planned 1-year evaluation periods and all of the planned 12-month QOL assessments occurred after randomization, so the cost in statistical power incurred by variable evaluation periods after randomization was minimal.
- We initially intended to use, but did not actually perform, multiple imputation analyses to address missing data. We instead used all available data in our analysis because missing data seemed to occur at random (however, we did not conduct formal analyses of missingness). Therefore, our study results may be biased due to dropout rates that exceeded 10% in some outcomes.
Future Research
We plan to disseminate the e-AT across multiple clinics in Utah. We shared our results with IH leaders, and they have expressed interested in disseminating the e-AT care model in their system. We are discussing with them the potential for funding and resources needed to achieve this goal. We hope to use this opportunity to conduct a rigorous pragmatic study that will allow better comparison of e-AT effectiveness relative to usual care. Schools are an additional setting for dissemination, as the e-AT can support school nurses' work to efficiently monitor many children with asthma, extending their reach to those from low socioeconomic households and racial/ethnic minorities experiencing asthma care disparities.
Conclusions
The new ambulatory care model supported by the e-AT was effective in engaging parents/children in sustained asthma self-management and was associated with improved asthma outcomes for patients, parents, and clinics. Dissemination of our new care model to more clinics can lead to major improvements in asthma care and outcomes, with potential reductions in asthma-related health care burden in the United States. The e-AT may also serve as a useful model for reducing asthma care disparities among underserved populations, a model that can be generalized to other pediatric chronic diseases. However, barriers to uptake and sustained use of such a care model in clinics need to be addressed for optimal benefits.
References
- 1.
- Akinbami LJ, Moorman JE, Liu X. Asthma prevalence, health care use, and mortality: United States, 2005-2009. Natl Health Stat Report. 2011;(32):1-14. [PubMed: 21355352]
- 2.
- CDC. National Health Interview Survey (NHIS) Data. 2009.
- 3.
- American Lung Association. Asthma and children fact sheet. 2012.
- 4.
- Lieu TA, Quesenberry CP, Sorel ME, Mendoza GR, Leong AB. Computer-based models to identify high-risk children with asthma. Am J Respir Crit Care Med. 1998;157(4 Pt 1):1173-1180. [PubMed: 9563736]
- 5.
- Chapman KR, Boulet LP, Rea RM, Franssen E. Suboptimal asthma control: prevalence, detection and consequences in general practice. Eur Respir J. 2008;31(2):320-325. [PubMed: 17959642]
- 6.
- New NHLBI guidelines for the diagnosis and management of asthma. National Heart, Lung and Blood Institute. Lippincott Health Promot Lett. 1997;2(7):1, 8-9. [PubMed: 9300898]
- 7.
- National Asthma Education and Prevention Program. Expert Panel Report 3 (EPR-3): guidelines for the diagnosis and management of asthma-summary report 2007. J Allergy Clin Immunol. 2007;120(5 Suppl):S94-S138. [PubMed: 17983880]
- 8.
- Ressel GW; Centers for Disease Control and Prevention, National Asthma Education and Prevention Program. NAEPP updates guidelines for the diagnosis and management of asthma. Am Fam Physician. 2003;68(1):169-170. [PubMed: 12887123]
- 9.
- Williams SG, Schmidt DK, Redd SC, Storms W; National Asthma Education and Prevention Program. Key clinical activities for quality asthma care. Recommendations of the National Asthma Education and Prevention Program. MMWR Recomm Rep. 2003;52(RR-6):1-8. [PubMed: 12696781]
- 10.
- Adams WG, Fuhlbrigge AL, Miller CW, et al. TLC-Asthma: an integrated information system for patient-centered monitoring, case management, and point-of-care decision support. AMIA Annu Symp Proc. 2003;2003:1-5. [PMC free article: PMC1480156] [PubMed: 14728122]
- 11.
- Cruz-Correia R, Fonseca J, Lima L, et al. Web-based or paper-based self-management tools for asthma-patients' opinions and quality of data in a randomized crossover study. Stud Health Technol Inform. 2007;127:178-189. [PubMed: 17901611]
- 12.
- Finkelstein J, Cabrera MR, Hripcsak G. Internet-based home asthma telemonitoring: can patients handle the technology? Chest. 2000;117:148-155. [PubMed: 10631213]
- 13.
- Janson SL, McGrath KW, Covington JK, Cheng SC, Boushey HA. Individualized asthma self-management improves medication adherence and markers of asthma control. J Allergy Clin Immunol. 2009;123:840-846. [PMC free article: PMC2729175] [PubMed: 19348923]
- 14.
- Joshi A, Amelung P, Arora M, Finkelstein J. Clinical impact of home automated telemanagement in asthma. AMIA Annu Symp Proc. 2005;2005:1000. [PMC free article: PMC1560591] [PubMed: 16779287]
- 15.
- Ostojic V, Cvoriscec B, Ostojic SB, Reznikoff D, Stipic-Markovic A, Tudjman Z. Improving asthma control through telemedicine: a study of short-message service. Telemed J E Health. 2005;11(1):28-35. [PubMed: 15785218]
- 16.
- van der Meer V, van Stel HF, Bakker MJ, et al. Weekly self-monitoring and treatment adjustment benefit patients with partly controlled and uncontrolled asthma: an analysis of the SMASHING study. Respir Res. 2010;11(1):74. [PMC free article: PMC2893513] [PubMed: 20537124]
- 17.
- Ahmad E, Grimes DE. The effects of self-management education for school-age children on asthma morbidity: a systematic review. J Sch Nurs. 2011;27:282-292. [PubMed: 21478414]
- 18.
- Bonner S, Zimmerman BJ, Evans D, Irigoyen M, Resnick D, Mellins RB. An individualized intervention to improve asthma management among urban Latino and African-American families. J Asthma. 2002;39:167-179. [PubMed: 11990232]
- 19.
- Perneger TV, Sudre P, Muntner P, et al. Effect of patient education on self-management skills and health status in patients with asthma: a randomized trial. Am J Med. 2002;113:7-14. [PubMed: 12106617]
- 20.
- Rhee H, Belyea MJ, Hunt JF, Brasch J. Effects of a peer-led asthma self-management program for adolescents. Arch Pediatr Adolesc Med. 2011;165:513-519. [PMC free article: PMC3252732] [PubMed: 21646583]
- 21.
- Guendelman S, Meade K, Benson M, Chen YQ, Samuels S. Improving asthma outcomes and self-management behaviors of inner-city children: a randomized trial of the Health Buddy interactive device and an asthma diary. Arch Pediatr Adolesc Med. 2002;156:114-120. [PubMed: 11814370]
- 22.
- Bheekie A, Syce JA, Weinberg EG. Peak expiratory flow rate and symptom self-monitoring of asthma initiated from community pharmacies. J Clin Pharm Ther. 2001;26:287-296. [PubMed: 11493372]
- 23.
- Thompson R, Delfino RJ, Tjoa T, Nussbaum E, Cooper D. Evaluation of daily home spirometry for school children with asthma: new insights. Pediatr Pulmonol. 2006;41:819-828. [PubMed: 16847876]
- 24.
- Chan DS, Callahan CW, Hatch-Pigott VB, et al. Internet-based home monitoring and education of children with asthma is comparable to ideal office-based care: results of a 1-year asthma in-home monitoring trial. Pediatrics. 2007;119:569-578. [PubMed: 17332210]
- 25.
- de Jongste JC, Carraro S, Hop WC, Baraldi E. Daily telemonitoring of exhaled nitric oxide and symptoms in the treatment of childhood asthma. Am J Respir Crit Care Med. 2009;179:93-97. [PubMed: 18931330]
- 26.
- McPherson AC, Glazebrook C, Forster D, James C, Smyth A. A randomized, controlled trial of an interactive educational computer package for children with asthma. Pediatrics. 2006;117:1046-1054. [PubMed: 16585298]
- 27.
- Willems DC, Joore MA, Hendriks JJ, Nieman FH, Severens JL, Wouters EF. The effectiveness of nurse-led telemonitoring of asthma: results of a randomized controlled trial. J Eval Clin Pract. 2008;14:600-609. [PubMed: 19126178]
- 28.
- Jan RL, Wang JY, Huang MC, Tseng SM, Su HJ, Liu LF. An internet-based interactive telemonitoring system for improving childhood asthma outcomes in Taiwan. Telemed J E Health. 2007;13:257-268. [PubMed: 17603828]
- 29.
- Joseph CL, Peterson E, Havstad S, et al. A web-based, tailored asthma management program for urban African-American high school students. Am J Respir Crit Care Med. 2007;175:888-895. [PMC free article: PMC1899296] [PubMed: 17290041]
- 30.
- Cuijpers CE, Wesseling GJ, Swaen GM, Sturmans F, Wouters EF. Asthma-related symptoms and lung function in primary school children. J Asthma. 1994;31:301-12. [PubMed: 8040154]
- 31.
- Davis KJ, Disantostefano R, Peden DB. Is Johnny wheezing? Parent-child agreement in the Childhood Asthma in America survey. Pediatr Allergy Immunol. 2011;22:31-35. [PubMed: 21261742]
- 32.
- Halterman JS, Yoos HL, Kitzman H, Anson E, Sidora-Arcoleo K, McMullen A. Symptom reporting in childhood asthma: a comparison of assessment methods. Arch Dis Child. 2006;91:766-770. [PMC free article: PMC2082923] [PubMed: 16705016]
- 33.
- McQuaid EL, Koinis Mitchell D, Walders N, et al. Pediatric asthma morbidity: the importance of symptom perception and family response to symptoms. J Pediatr Psychol. 2007;32:167-177. [PubMed: 16717140]
- 34.
- Tirimanna PR, Den Otter JJ, Van Schayck CP, Van Herwaarden CL, Folgering H, Van Weel C. Evaluation of the suitability of weekly peak expiratory flow rate measurements in monitoring annual decline in lung function among patients with asthma and chronic bronchitis. Br J Gen Pract. 1996;46:15-18. [PMC free article: PMC1239505] [PubMed: 8745846]
- 35.
- NHLBI-EPR-3. Guidelines for the Diagnosis and Management of Asthma. Periodic Assessment and Monitoring: Essential for Asthma Management. National Heart, Lung, and Blood Institute; 2007.
- 36.
- Frischer T, Meinert R, Urbanek R, Kuehr J. Variability of peak expiratory flow rate in children: short and long term reproducibility. Thorax. 1995;50:35-39. [PMC free article: PMC473702] [PubMed: 7886646]
- 37.
- Goldberg S, Springer C, Avital A, Godfrey S, Bar-Yishay E. Can peak expiratory flow measurements estimate small airway function in asthmatic children? Chest. 2001;120:482-488. [PubMed: 11502647]
- 38.
- McGrath AM, Gardner DM, McCormack J. Is home peak expiratory flow monitoring effective for controlling asthma symptoms? J Clin Pharm Ther. 2001;26:311-317. [PubMed: 11679021]
- 39.
- Juniper EF, Gruffydd-Jones K, Ward S, Svensson K. Asthma Control Questionnaire in children: validation, measurement properties, interpretation. Eur Respir J. 2010;36:1410-1416. [PubMed: 20530041]
- 40.
- Jia CE, Zhang HP, Lv Y, et al. The Asthma Control Test and Asthma Control Questionnaire for assessing asthma control: systematic review and meta-analysis. J Allergy Clin Immunol. 2013;131:695-703. [PubMed: 23058645]
- 41.
- Nathan RA, Sorkness CA, Kosinski M, et al. Development of the asthma control test: a survey for assessing asthma control. J Allergy Clin Immunol. 2004;113:59-65. [PubMed: 14713908]
- 42.
- Schatz M, Sorkness CA, Li JT, et al. Asthma Control Test: reliability, validity, and responsiveness in patients not previously followed by asthma specialists. J Allergy Clin Immunol. 2006;117:549-556. [PubMed: 16522452]
- 43.
- Nkoy F, Stone B, Fassl B, et al. Development of a novel tool for engaging children and parents in asthma self-management. AMIA Annu Symp Proc. 2012;2012:663-672. [PMC free article: PMC3540453] [PubMed: 23304339]
- 44.
- Nkoy FL, Stone BL, Fassl BA, et al. Longitudinal validation of a tool for asthma self-monitoring. Pediatrics. 2013;132:e1554-e1561. [PMC free article: PMC4074668] [PubMed: 24218469]
- 45.
- Nkoy F, Stone B, Fassl B, al e. Validation of a pediatric asthma self-monitoring tool. Presented at: Pediatric Academic Societies Conference; April 28-May 1, 2012; Boston, MA.
- 46.
- Nkoy F, Stone B, Fassl B, et al. A self-monitoring tool for improving ambulatory asthma care in children. Presented at: AcademyHealth Annual Conference; 2012; Orlando, FL.
- 47.
- Liu AH, Zeiger R, Sorkness C, et al. Development and cross-sectional validation of the Childhood Asthma Control Test. J Allergy Clin Immunol. 2007;119:817-825. [PubMed: 17353040]
- 48.
- Diette GB, Markson L, Skinner EA, Nguyen TT, Algatt-Bergstrom P, Wu AW. Nocturnal asthma in children affects school attendance, school performance, and parents' work attendance. Arch Pediatr Adolesc Med. 2000;154:923-928. [PubMed: 10980797]
- 49.
- James BC, Savitz LA. How Intermountain trimmed health care costs through robust quality improvement efforts. Health Aff (Millwood). 2011;30(6):1185-1191. [PubMed: 21596758]
- 50.
- Byington CL, Reynolds CC, Korgenski K, et al. Costs and infant outcomes after implementation of a care process model for febrile infants. Pediatrics. 2012;130:e16-e24. [PMC free article: PMC4074609] [PubMed: 22732178]
- 51.
- Gorelick MH, Brousseau DC, Stevens MW. Validity and responsiveness of a brief, asthma-specific quality-of-life instrument in children with acute asthma. Ann Allergy Asthma Immunol. 2004;92:47-51. [PubMed: 14756464]
- 52.
- Kwok MY, Walsh-Kelly CM, Gorelick MH, Grabowski L, Kelly KJ. National Asthma Education and Prevention Program severity classification as a measure of disease burden in children with acute asthma. Pediatrics. 2006;117:S71-S77. [PubMed: 16777834]
- 53.
- Evans RS, Lloyd JF, Pierce LA. Clinical use of an enterprise data warehouse. AMIA Annu Symp Proc. 2012;2012:189-198. [PMC free article: PMC3540441] [PubMed: 23304288]
- 54.
- Parker RM, Baker DW, Williams MV, Nurss JR. The test of functional health literacy in adults: a new instrument for measuring patients' literacy skills. J Gen Intern Med. 1995;10:537-541. [PubMed: 8576769]
- 55.
- Varmi J. Development of the Pediatric Hematology/Oncology Parent Satisfaction Survey. Child Health Care. 2000;29:243-255.
- 56.
- Bonini M. Electronic health (e-Health): emerging role in asthma. Curr Opin Pulm Med. 2017;23:21-26. [PubMed: 27763999]
- 57.
- Voorend-van Bergen S, Vaessen-Verberne AA, Landstra AM, et al. Monitoring childhood asthma: web-based diaries and the asthma control test. J Allergy Clin Immunol. 2014;133:1599-1605.e2. [PubMed: 24290276]
- 58.
- Rikkers-Mutsaerts ER, Winters AE, Bakker MJ, et al. Internet-based self-management compared with usual care in adolescents with asthma: a randomized controlled trial. Pediatr Pulmonol. 2012;47:1170-1179. [PubMed: 22644646]
- 59.
- Rabe KF, Adachi M, Lai CK, et al. Worldwide severity and control of asthma in children and adults: the global asthma insights and reality surveys. J Allergy Clin Immunol. 2004;114:40-47. [PubMed: 15241342]
- 60.
- Sapra S, Chang E. Alignment with the revised NHLBI 2007 asthma guidelines, Expert Panel Report 3 (EPR 3) in a large payer database. J Allergy Clin Immunol. 2009;123:S117.
- 61.
- Stempel DA, McLaughin TP, Stanford RH, Fuhlbrigge AL. Patterns of asthma control: a 3-year analysis of patient claims. J Allergy Clin Immunol. 2005;115:935-939. [PubMed: 15867848]
- 62.
- Humbert M. The right tools at the right time. Chest. 2006;130:29S-40S. [PubMed: 16840366]
- 63.
- Barnes PJ. Achieving asthma control. Curr Med Res Opin. 2005;21 Suppl 4:S5-S9. [PubMed: 16138939]
- 64.
- Bloomberg GR, Banister C, Sterkel R, et al. Socioeconomic, family, and pediatric practice factors that affect level of asthma control. Pediatrics. 2009;123:829-835. [PMC free article: PMC2723164] [PubMed: 19255010]
- 65.
- Kaya Z, Erkan F, Ozkan M, et al. Self-management plans for asthma control and predictors of patient compliance. J Asthma. 2009;46:270-275. [PubMed: 19373635]
- 66.
- Wensley DC, Silverman M. The quality of home spirometry in school children with asthma. Thorax. 2001;56:183-185. [PMC free article: PMC1758778] [PubMed: 11182009]
- 67.
- Morrison D, Wyke S, Agur K, et al. Digital asthma self-management interventions: a systematic review. J Med Internet Res. 2014;16(2):e51. doi:10.2196/jmir.2814 [PMC free article: PMC3958674] [PubMed: 24550161] [CrossRef]
Acknowledgment
Research reported in this report was [partially] funded through a Patient-Centered Outcomes Research Institute® (PCORI®) Award (#IH-12-11-5330) Further information available at: https://www.pcori.org/research-results/2013/does-advanced-electronic-tracker-help-families-manage-childrens-asthma
Appendix
Survey Questionnaire (PDF, 174K)
Footnotes
*Note: As stated in the Background section, our efforts to improve ambulatory asthma care began with the development [in 2010] and validation of the paper-AT and moved to the design [in 2012] and pilot testing of the e-AT.43,45,46 The paper-AT is a user-friendly tool that engages parents in their child's asthma self-management. We developed it by using the ACT questionnaire,47 which we adapted and validated for weekly assessment of asthma control. We augmented the paper-AT with graphical display of weekly ACT scores, coupled with decision support to guide appropriate and timely responses by parents/children and PCPs to early signs of asthma control deteriorations. The e-AT addresses the paper-AT limitations by adding real-time feedback and alerts to parents and PCPs.46
Suggested citation:
Nkoy FL, Fassl BA, Wilkins VL, et al. (2019). Does an Advanced Electronic Tracker Help Families Manage Children's Asthma Symptoms Better Than a Standard Electronic Tracker? Patient-Centered Outcomes Research Institute (PCORI). https://doi.org/10.25302/10.2019.IH.12115330
Disclaimer
The [views, statements, opinions] presented in this report are solely the responsibility of the author(s) and do not necessarily represent the views of the Patient-Centered Outcomes Research Institute® (PCORI®), its Board of Governors or Methodology Committee.
- Suicidal Ideation.[StatPearls. 2024]Suicidal Ideation.Harmer B, Lee S, Duong TVH, Saadabadi A. StatPearls. 2024 Jan
- Ambulatory Management of Childhood Asthma Using a Novel Self-management Application.[Pediatrics. 2019]Ambulatory Management of Childhood Asthma Using a Novel Self-management Application.Nkoy FL, Fassl BA, Wilkins VL, Johnson J, Unsicker EH, Koopmeiners KJ, Jensen A, Frazier M, Gaddis J, Malmgren L, et al. Pediatrics. 2019 Jun; 143(6). Epub 2019 May 16.
- Folic acid supplementation and malaria susceptibility and severity among people taking antifolate antimalarial drugs in endemic areas.[Cochrane Database Syst Rev. 2022]Folic acid supplementation and malaria susceptibility and severity among people taking antifolate antimalarial drugs in endemic areas.Crider K, Williams J, Qi YP, Gutman J, Yeung L, Mai C, Finkelstain J, Mehta S, Pons-Duran C, Menéndez C, et al. Cochrane Database Syst Rev. 2022 Feb 1; 2(2022). Epub 2022 Feb 1.
- Review Examining Home Visits from Community Health Workers to Help Patients Manage Asthma Symptoms[ 2021]Review Examining Home Visits from Community Health Workers to Help Patients Manage Asthma SymptomsStout J, Chen R, Farquhar S, Kramer B, Song L. 2021 Dec
- Review Comparing Two Ways to Help Patients Get Follow-up Care after a Mental Health Visit to the Emergency Room—The EPIC Study[ 2021]Review Comparing Two Ways to Help Patients Get Follow-up Care after a Mental Health Visit to the Emergency Room—The EPIC StudyDruss B, Lally CA, Li J, Tapscott S, Walker ER. 2021 May
- Does an Advanced Electronic Tracker Help Families Manage Children's Asthma Sympt...Does an Advanced Electronic Tracker Help Families Manage Children's Asthma Symptoms Better Than a Standard Electronic Tracker?
Your browsing activity is empty.
Activity recording is turned off.
See more...